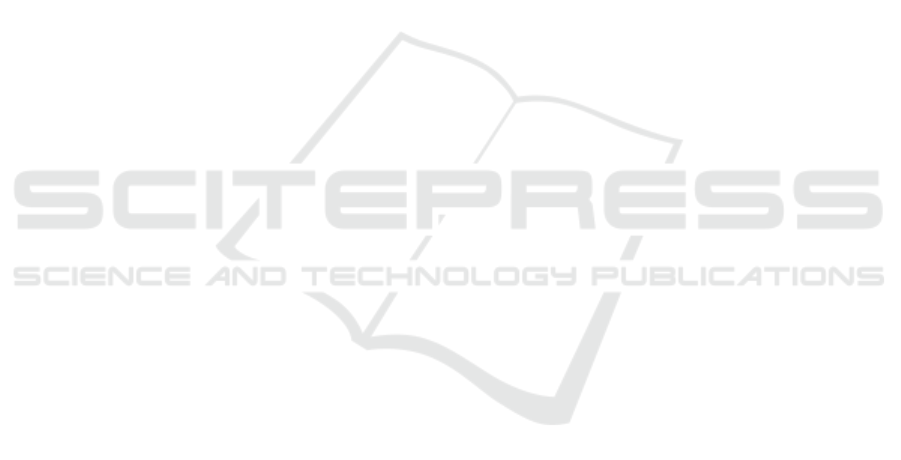
that the BACK location captures more of the dynam-
ics of the lower extremities than the RUA location.
Therefore by roaming the samples from the BACK lo-
cation to the RUA location, together with the relative
similarity in the dynamics captured by both locations,
near-native predictive performance is gained on activ-
ities whose effects have an impact on both the BACK
and RUA locations.
6.4 Discussion
In this section we summarize inferences obtained
from the results and their subsequent discussion in the
preceding subsections.
Considering the general pattern of results ob-
tained, it can be inferred that the proposed roaming
technique performs best between proximal body lo-
cations (e.g RLA-RUA, RUA-BACK, etc). This can
be attributed to the fact that proximal body locations
are similarly affected by the dynamics of a given set
of activities. Additionally, roaming performance is
maximized when the source body location is itself
suitably discriminative of the considered activities.
This follows due to the reason that the source loca-
tion must itself contain sufficient recognition infor-
mation, which may then be roamed for reuse. We
can thus surmise that, in general, the predictive per-
formances obtained through roaming (relative to the
reported upper-bounds) indicate that the roamed sam-
ples can be useful in solving the activity recognition
problem.
7 CONCLUSION AND FUTURE
WORK
In this work we explore the feasibility of constructing
”roaming” models, which are capable of transform-
ing samples between different body locations. We
propose a technique based on Bidirectional Recurrent
Neural Networks and apply our proposed technique
to a multi-sensor, multi-location dataset consisting of
33 activities involving different regions of the body.
The results obtained suggest that roaming models can
be feasible between proximal body locations, and the
highest gains from their use result from the consid-
eration of activities whose dynamics are adequately
captured by the source location.
In the future we would like to explore more body
locations to gain a more holistic insight into the per-
formance and feasibility of such models. Addition-
ally, we will investigate roaming models based on the
use of more than one source body location. Further-
more, the feasibility of activity class-specific (e.g up-
per body activities) roaming models may be explored
in order to obtain better roaming performance as op-
posed to using a general roaming model for all activ-
ities.
REFERENCES
Ba
˜
nos, O., Damas, M., Pomares, H., Rojas, I., T
´
oth, M. A.,
and Amft, O. (2012). A benchmark dataset to eval-
uate sensor displacement in activity recognition. In
Proceedings of the 2012 ACM Conference on Ubiqui-
tous Computing, pages 1026–1035. ACM.
Banos, O., Galvez, J.-M., Damas, M., Pomares, H., and Ro-
jas, I. (2014a). Window size impact in human activity
recognition. Sensors, 14(4):6474–6499.
Banos, O., Toth, M., Damas, M., Pomares, H., and Rojas,
I. (2014b). Dealing with the effects of sensor dis-
placement in wearable activity recognition. Sensors,
14(6):9995–10023.
Cho, K., Van Merri
¨
enboer, B., Gulcehre, C., Bahdanau, D.,
Bougares, F., Schwenk, H., and Bengio, Y. (2014).
Learning phrase representations using rnn encoder-
decoder for statistical machine translation. arXiv
preprint arXiv:1406.1078.
Cybenko, G. (1989). Approximation by superpositions of
a sigmoidal function. Mathematics of control, signals
and systems, 2(4):303–314.
Elbasiony, R. and Gomaa, W. (2018). Humanoids skill
learning based on real-time human motion imitation
using kinect. Intelligent Service Robotics, 11(2):149–
169.
Gomaa, W., Elbasiony, R., and Ashry, S. (2017). Adl clas-
sification based on autocorrelation function of inertial
signals. In 2017 16th IEEE International Conference
on Machine Learning and Applications (ICMLA),
pages 833–837. IEEE.
Hochreiter, S. and Schmidhuber, J. (1997). Long short-term
memory. Neural computation, 9(8):1735–1780.
Hoque, E. and Stankovic, J. (2012). Aalo: Activity recog-
nition in smart homes using active learning in the
presence of overlapped activities. In 2012 6th Inter-
national Conference on Pervasive Computing Tech-
nologies for Healthcare (PervasiveHealth) and Work-
shops, pages 139–146. IEEE.
Hu, D. H. and Yang, Q. (2011). Transfer learning for
activity recognition via sensor mapping. In Twenty-
second international joint conference on artificial in-
telligence.
Hu, D. H., Zheng, V. W., and Yang, Q. (2011). Cross-
domain activity recognition via transfer learning. Per-
vasive and Mobile Computing, 7(3):344–358.
Khan, M. A. A. H. and Roy, N. (2017). Transact: Trans-
fer learning enabled activity recognition. In 2017
IEEE International Conference on Pervasive Comput-
ing and Communications Workshops (PerCom Work-
shops), pages 545–550.
On the Feasibility of On-body Roaming Models in Human Activity Recognition
689