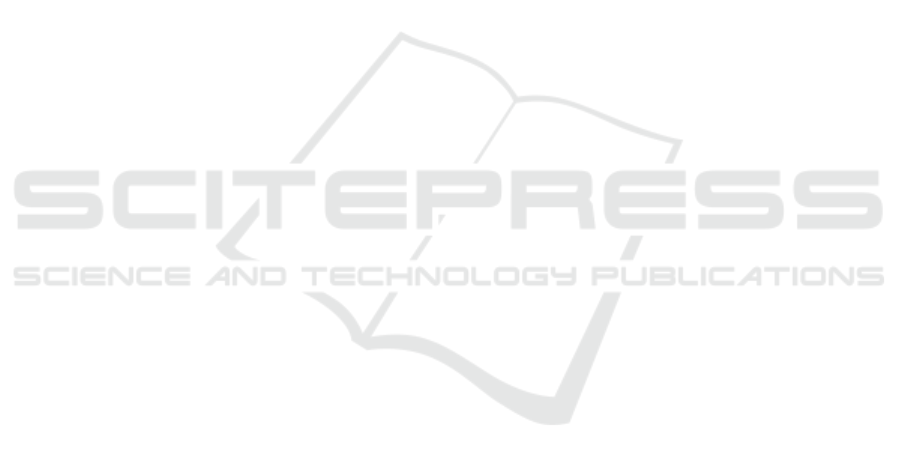
wavelet. The BTSPC algorithm capitalizes on this
fact and is able to then create a piecewise comparison
between the trading behaviors of the stock indexes
across all frequency ranges and across time avoiding
the problems of resolution which were inherent to tra-
ditional DFT analysis methods. Further, the BTSPC
cluster results create a dynamic image of the mar-
ket behavior in question which yields itself to data
driven analysis approach due to the fact that the al-
gorithm utilizes Morlet wavelets. Consequently, the
BTSPC method is vastly superior for analyzing poten-
tially thousands of market indexes compared with tra-
ditional analysis approaches which require active user
guidance such as ARIMA, neural networks or Fourier
transforms. Finally, unlike ARIMA, neural networks
and Fourier transform analysis, it’s important to note
that data analyzed by BTSPC requires no data pre-
processing (transformations to linearity, forcing sta-
tionarity via differencing, etc) which vastly simplifies
any given macro-economic analysis.
In this study, it was discovered that all of the
gold mining firms (AUY, BTG, CDE, AKG, EGO,
GG) exhibited increased high-frequency activity dur-
ing the recessionary period of 2008 followed by a
brief low-frequency/low intensity recovery phase un-
til 2013 when the stock prices across all indexes in
the study began declining. The BTSPC algorithm was
utilized to compare the motifs contained within the re-
spective time series by constructing a matrix of power
curves. It was then found that the gold mining firms
in this study formed two cluster families AUY, EGO
and GG and BTG, CDE, AKG. It was also shown that
CDE and AKG are the most dissimilar time series
in the analysis which is due to the more pronounced
volatility contained within the individual series itself.
It is speculated that the increased volatility is in part
due to the perception that CDE and AKG both may be
perceived as risky investments and negative investor
sentiment is therefore influencing these indexes.
Future work could include testing the BTSPC al-
gorithm to different market sectors in order to form a
broader understanding of trading behavior. Since the
BTSPC can be utilized to provide qualitative and pre-
dictive information from any two (or more) signals,
any situations where there exists two or more concur-
rent dynamic processes within a macro-process the
BTSPC can be utilized in order to dynamically ana-
lyze how these processes behave with respect to one
another within the framework of the macro-process
itself. For example, if we know that two or more
stock indexes currently exhibit similarity (aka co-
movement) as evidenced by the BTSPC algorithm and
clustering then one can predict radical cross-market
changes by merely examining whatever stock begins
to diverge from the other stocks in the analysis. Thus,
one can use the BTSPC to creating a dynamic or real
time visualization of market dynamics for investors
and financial institutions. Similarly, one could extend
the BTSPC algorithm to examinations of emergent
behavior in ecology, meteorological applications, bi-
ological systems, etc. Finally, BTSPC could be ap-
plied to social media data mining and search result
optimization as it would allow one to visualize the
differences between keywords across frequencies and
time which would provide information about user be-
havior. The applications of the BTSPC algorithm are
limitless.
REFERENCES
Aguiar-Conraria, L., Azevedo, N., and Soares, M. J. (2008).
Using wavelets to decompose the time–frequency ef-
fects of monetary policy. Physica A: Statistical me-
chanics and its Applications, 387(12):2863–2878.
Choudhary, M. A. and Haider, A. (2012). Neural network
models for inflation forecasting: an appraisal. Applied
Economics, 44(20):2631–2635.
Defays, D. (1977). An efficient algorithm for a complete
link method. The Computer Journal, 20(4):364–366.
Dowson, D. and Landau, B. (1982). The fr
´
echet distance
between multivariate normal distributions. Journal of
multivariate analysis, 12(3):450–455.
Ehlers, J. F. (2007). Fourier transform for traders. TECHNI-
CAL ANALYSIS OF STOCKS AND COMMODITIES-
MAGAZINE EDITION-, 25(1):24.
Fahimifard, S., Homayounifar, M., Sabouhi, M., and
Moghaddamnia, A. (2009). Comparison of anfis, ann,
garch and arima techniques to exchange rate forecast-
ing. Journal of Applied Sciences, 9(20):3641–3651.
Gallegati, M., Ramsey, J. B., and Semmler, W. (2014). In-
terest rate spreads and output: A time scale decompo-
sition analysis using wavelets. Computational Statis-
tics & Data Analysis, 76:283–290.
Goupillaud, P., Grossmann, A., and Morlet, J. (1984).
Cycle-octave and related transforms in seismic signal
analysis. Geoexploration, 23(1):85–102.
Huang, C., Huang, L.-l., and Han, T.-t. (2012). Financial
time series forecasting based on wavelet kernel sup-
port vector machine. In 2012 8th International Con-
ference on Natural Computation, pages 79–83. IEEE.
Huang, J.-N., Li, H., Maechler, M., Martin, R. D., and
Schimert, J. (1992). A comparison of projection
pursuit and neural network regression modeling. In
Advances in Neural Information Processing Systems,
pages 1159–1166.
Leybourne, S. J., McCabe, B. P., and Tremayne, A. R.
(1996). Can economic time series be differenced to
stationarity? Journal of Business & Economic Statis-
tics, 14(4):435–446.
Richette, P., Clerson, P., P
´
erissin, L., Flipo, R.-M., and
Bardin, T. (2015). Revisiting comorbidities in gout:
DATA 2019 - 8th International Conference on Data Science, Technology and Applications
82