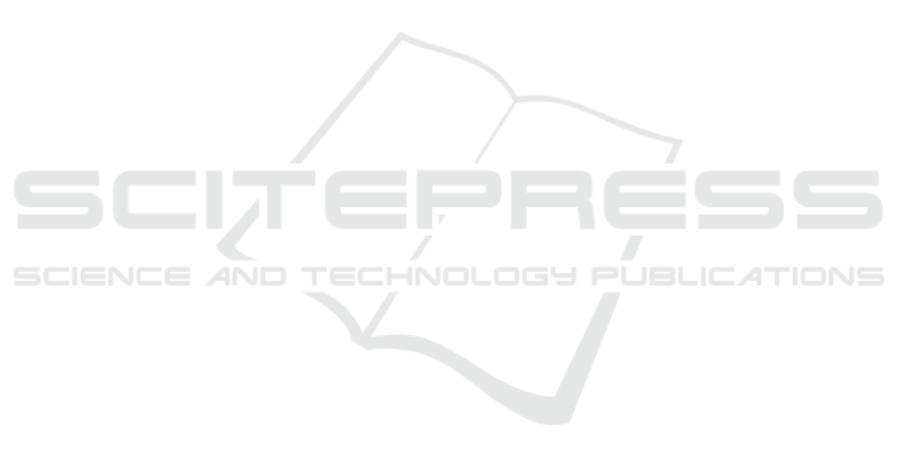
ACKNOWLEDGEMENTS
The work was partially supported by the Grant No.
0401/0136/18, WUS&T, Poland.
REFERENCES
Bai, E. (2010). Non-parametric nonlinear system identifi-
cation: an asymptotic minimum mean squared error
estimator. IEEE Transactions on Automatic Control,
55(7):1615–1626.
Biega
´
nski, M. (2018). Multi-level identification of
Hammerstein-Wiener (N-L-N) system in active exper-
iment. In Proceedings of the 15th International Con-
ference on Informatics in Control, Automation and
Robotics, ICINCO, Porto, Portugal, pages 355–361.
Boyd, S., Chua, L. O., and Desoer, C. (1984). Analytical
foundations of Volterra series. IMA Journal of Math-
ematical Control and Information, 1(3):243–282.
Cristobal, J., Roca, P., and Manteiga, W. (1987). A class
of linear regression parameter estimators constructed
by nonparametric estimation. The Annals of Statistics,
15(2):603–609.
Efromovich, S. (1999). Nonparametric Curve Estimation.
Springer, New York.
Gauss, C. F. and Davis, C. H. (1857). Theory
of the motion of the heavenly bodies moving
about the sun in conic sections a translation of
Gauss’s. Boston,Little, Brown and company,. https://
www.biodiversitylibrary.org/bibliography/19023.
Giannakis, G. and Serpedin, E. (2001). A bibliography on
nonlinear system identification. Signal Processing,
81(3):533–580.
Giri, F. and Bai, E. W. (2010). Block-Oriented Nonlinear
System Identification. Lecture Notes in Control and
Information Sciences 404. Springer.
Greblicki, W. (2010). Nonparametric input density-free es-
timation of the nonlinearity in Wiener systems. IEEE
Transactions on Information Theory, 56(7):3575–
3580.
Greblicki, W. and Mzyk, G. (2009). Semiparametric ap-
proach to Hammerstein system identification. In Pro-
ceedings of the 15th IFAC Symposium on System Iden-
tification, Saint-Malo, France, pages 1680–1685.
Greblicki, W. and Pawlak, M. (1986). Identification of
discrete Hammerstein systems using kernel regression
estimates. IEEE Transactions on Automatic Control,
31:74–77.
Greblicki, W. and Pawlak, M. (2008). Nonparametric Sys-
tem Identification. Cambridge University Press.
Gyorfi, L., Kohler, M., Krzy
˙
zak, A., and Walk, H. (2002).
A Distribution-Free Theory of Nonparametric Regres-
sion. Springer Verlag, New York.
H
¨
ardle, W. (1990). Applied Nonparametric Regression.
Cambridge University Press, Cambridge.
Hasiewicz, Z. and Mzyk, G. (2004). Combined parametric-
nonparametric identification of Hammerstein sys-
tems. IEEE Transactions on Automatic Control,
48(8):1370–1376.
Hasiewicz, Z. and Mzyk, G. (2009). Hammerstein system
identification by non-parametric instrumental vari-
ables. International Journal of Control, 82(3):440–
455.
Hasiewicz, Z., Pawlak, M., and
´
Sliwi
´
nski, P. (2005). Non-
parametric identification of nonlinearities in block-
oriented systems by orthogonal wavelets with com-
pact support. IEEE Transactions on Circuits and Sys-
tems I: Regular Papers, 52(2):427–442.
Mzyk, G. (2007). A censored sample mean approach
to nonparametric identification of nonlinearities in
Wiener systems. IEEE Transactions on Circuits and
Systems – II: Express Briefs, 54(10):897–901.
Mzyk, G. (2010). Parametric versus nonparametric ap-
proach to Wiener systems identification. Lecture
Notes in Control and Information Sciences, 404:111–
125.
Mzyk, G. (2012). Nonparametric recovering of nonlinear-
ity in Wiener-Hammerstein systems. In Proceedings
of the 9th International Conference on Informatics in
Control, Automation and Robotics, ICINCO, Rome,
Italy, pages 439–445. IEEE.
Mzyk, G. (2014). Combined Parametric-Nonparametric
Identification of Block-Oriented Systems. Lecture
Notes in Control and Information Sciences 454.
Springer.
Mzyk, G. and Wachel, P. (2017). Kernel-based identifi-
cation of Wiener-Hammerstein system. Automatica,
83:275–281.
Nadaraya, E. (1964). On estimating regression. Teoriya
Veroyatnostei i ee Primeneniya, 9(1):157–159.
Narendra, K. and Gallman, P. G. (1966). An iterative
method for the identification of nonlinear systems us-
ing the Hammerstein model. IEEE Transactions on
Automatic Control, 11:546–550.
Pawlak, M., Hasiewicz, Z., and Wachel, P. (2007). On non-
parametric identification of Wiener systems. IEEE
Transactions on Signal Processing, 55(2):482–492.
Pintelon, R. and Schoukens, J. (2004). System Identifica-
tion: a Frequency Domain Approach. Wiley-IEEE
Press.
Rochdi, Y., Giri, F., Gning, J., and Chaoui, F. (2010). Iden-
tification of block-oriented systems in the presence
of nonparametric input nonlinearities of switch and
backlash types. Automatica, 46(5):864–877.
Ruppert, D., Wand, M., and Carroll, R. (2003). Semipara-
metric Regression, volume 12. Cambridge University
Press.
´
Sliwi
´
nski, P. (2013). Nonlinear System Identification by
Haar Wavelets. Lecture Notes in Statistics 210.
Springer.
Stone, C. (1982). Optimal global rates of convergence for
nonparametric regression. The Annals of Statistics,
10(4):1040–1053.
Wachel, P. and Mzyk, G. (2016). Direct identification of the
linear block in Wiener system. International Journal
of Adaptive Control and Signal Processing, 30(1):93–
105.
Wand, M. and Jones, H. (1995). Kernel Smoothing. Chap-
man and Hall, London.
ICINCO 2019 - 16th International Conference on Informatics in Control, Automation and Robotics
698