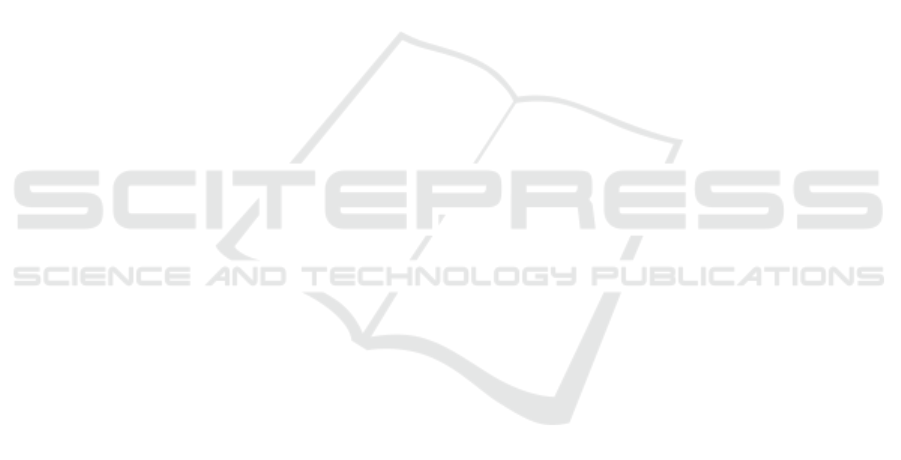
the injection molding process using multistage exper-
imental design. Quality Engineering, 26(3):319–334.
Buehlmann, P. and Hothorn, T. (2007). Boosting algo-
rithms: Regularization, prediction and model fitting.
Statist. Sci., 22(4):477–505.
Chang, T. C. and Faison III, E. (2001). Shrinkage behavior
and optimization of injection molded parts studied by
the taguchi method. Polymer Engineering & Science,
41(5):703–710.
Chen, W.-C., Nguyen, M.-H., Chiu, W.-H., Chen, T.-N.,
and Tai, P.-H. (2016). Optimization of the plastic
injection molding process using the taguchi method,
rsm, and hybrid ga-pso. The International Journal
of Advanced Manufacturing Technology, 83(9):1873–
1886.
Cribari-Neto, F. and Zeileis, A. (2010). Beta regression in r.
Journal of Statistical Software, Articles, 34(2):1–24.
Dang, X.-P. (2014). General frameworks for optimization
of plastic injection molding process parameters. Sim-
ulation Modelling Practice and Theory, 41:15 – 27.
Drucker, H., Burges, C. J. C., Kaufman, L., Smola, A.,
and Vapnik, V. (1996). Support vector regression ma-
chines. In Proceedings of the 9th International Con-
ference on Neural Information Processing Systems,
NIPS’96, pages 155–161, Cambridge, MA, USA.
MIT Press.
Fernandes, C., Pontes, A. J., Viana, J. C., and Gaspar-
Cunha, A. (2018). Modeling and optimization of the
injection-molding process: A review. Advances in
Polymer Technology, 37(2):429–449.
Ferrari, S. and Cribari-Neto, F. (2004). Beta regression for
modelling rates and proportions. Journal of Applied
Statistics, 31(7):799–815.
Hofner, B., Mayr, A., and Schmid, M. (2016). gamboostlss:
An r package for model building and variable selec-
tion in the gamlss framework. Journal of Statistical
Software, Articles, 74(1):1–31.
Jahan, S. A. and El-Mounayri, H. (2016). Optimal con-
formal cooling channels in 3d printed dies for plastic
injection molding. Procedia Manufacturing, 5:888
– 900. 44th North American Manufacturing Re-
search Conference, NAMRC 44, June 27-July 1,
2016, Blacksburg, Virginia, United States.
Kashyap, S. and Datta, D. (2015). Process parameter opti-
mization of plastic injection molding: a review. Inter-
national Journal of Plastics Technology, 19(1):1–18.
Mao, T., Zhang, Y., Ruan, Y., Gao, H., Zhou, H., and Li, D.
(2018). Feature learning and process monitoring of
injection molding using convolution-deconvolution
auto encoders. Computers & Chemical Engineering,
118:77 – 90.
Oliaei, E., Heidari, B. S., Davachi, S. M., Bahrami, M.,
Davoodi, S., Hejazi, I., and Seyfi, J. (2016). Warpage
and shrinkage optimization of injection-molded plas-
tic spoon parts for biodegradable polymers using
taguchi, anova and artificial neural network meth-
ods. Journal of Materials Science & Technology,
32(8):710 – 720.
Packianather, M., Griffiths, C., and Kadir, W. (2015). Micro
injection moulding process parameter tuning. Proce-
dia CIRP, 33:400 – 405. 9th CIRP Conference on
Intelligent Computation in Manufacturing Engineer-
ing - CIRP ICME ’14.
Ribeiro, B. (2005). Support vector machines for qual-
ity monitoring in a plastic injection molding process.
IEEE Transactions on Systems, Man, and Cybernet-
ics, Part C (Applications and Reviews), 35(3):401–
410.
Rigby, R. A. and Stasinopoulos, D. M. (2005). Generalized
additive models for location, scale and shape. Jour-
nal of the Royal Statistical Society. Series C (Applied
Statistics), 54(3):507–554.
Schmid, M., Wickler, F., Maloney, K. O., Mitchell, R.,
Fenske, N., and Mayr, A. (2013). Boosted beta re-
gression. PLOS ONE, 8(4):1–15.
Simas, A. B., Barreto-Souza, W., and Rocha, A. V. (2010).
Improved estimators for a general class of beta regres-
sion models. Computational Statistics & Data Anal-
ysis, 54(2):348 – 366.
Singh, G. and Verma, A. (2017). A brief review on in-
jection moulding manufacturing process. Materials
Today: Proceedings, 4(2, Part A):1423 – 1433. 5th
International Conference of Materials Processing and
Characterization (ICMPC 2016).
Taguchi, G., Elsayed, E., and Hsiang, T. (1989). Qual-
ity engineering in production systems. McGraw-Hill
series in industrial engineering and management sci-
ence. McGraw-Hill.
Taguchi, G., Konishi, S., Konishi, S., and Institute, A. S.
(1987). Orthogonal Arrays and Linear Graphs: Tools
for Quality Engineering. Taguchi methods. American
Supplier Institute.
Thomas, J., Mayr, A., Bischl, B., Schmid, M., Smith, A.,
and Hofner, B. (2018). Gradient boosting for distri-
butional regression: faster tuning and improved vari-
able selection via noncyclical updates. Statistics and
Computing, 28(3):673–687.
Unal, R. and Dean, E. B. (1991). Taguchi approach to de-
sign optimization for quality and cost : An overview.
Vapnik, V. N. (1995). The Nature of Statistical Learning
Theory. Springer-Verlag, Berlin, Heidelberg.
Yin, F., Mao, H., and Hua, L. (2011a). A hybrid of back
propagation neural network and genetic algorithm for
optimization of injection molding process parame-
ters. Materials & Design, 32(6):3457 – 3464.
Yin, F., Mao, H., Hua, L., Guo, W., and Shu, M.
(2011b). Back propagation neural network model-
ing for warpage prediction and optimization of plas-
tic products during injection molding. Materials &
Design, 32(4):1844 – 1850.
Zafo
ˇ
snik, B., Bo
ˇ
zi
ˇ
c, U., and Florjani
ˇ
c, B. (2015). Mod-
elling of an analytical equation for predicting maxi-
mum stress in an injections moulded undercut geome-
try during ejection. International Journal of Precision
Engineering and Manufacturing, 16(12):2499–2507.
Approaches to Identify Relevant Process Variables in Injection Moulding using Beta Regression and SVM
241