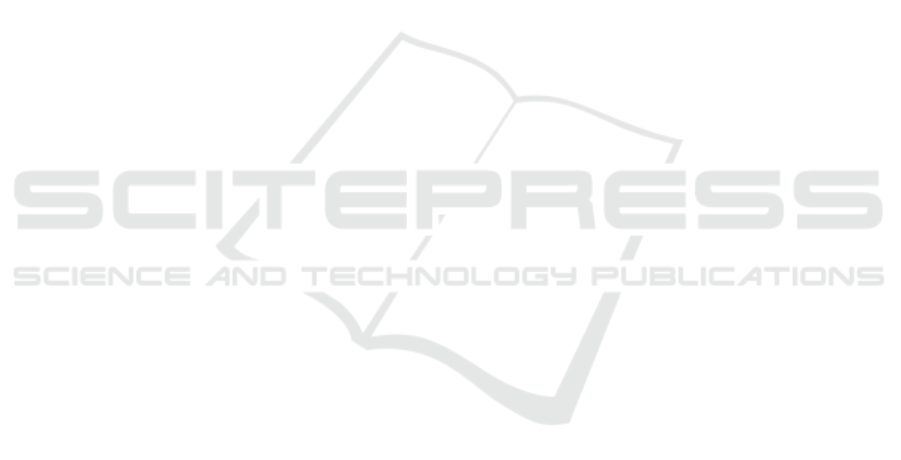
Experience bias exists towards early period (1-4
years) of the participants in Big data systems in
industry. This threat remains at the early stage of the
field of Big Data, but we hope that participants in the
future studies on the artefact-model will have gained
further experience to minimise this type of bias.
Finally, threats to conclusion validity are
concerned with whether conclusions are traceable to
the findings (Runeson and Host, 2009). This threat is
considered contained since all the conclusions
presented are shown to have been rooted in specific
sections of this paper.
7 CONCLUSIONS
Whereas much attention has been given to analytics
concerning Big Data, little amount of attention has
been invested in the development of software
applications and services leveraging Big Data. This
situation is also reflected in the field of RE where
domain models, processes, methods, techniques and
tools have not yet embraced Big Data in a significant
way. To ameliorate this situation, in 2017, we had
created a preliminary RE artefact model to aid the
development of Big Data software applications
(Arruda and Madhavji, 2017).
In this paper, we describe how we have taken the
early result to the next level by having the model
validated by ten third-party practitioners from diverse
Big Data software development projects.
Specifically, the model was validated on its qualities
such as: accuracy, completeness, usefulness, and
generalisability (see Section 4). This paper gives
details of the validation study, such as descriptive
statistics of the study participants and application
domains in industry (see Subsection 4.1); data
gathered and analysed (see Subsection 4.2); and the
resultant, improved, artefact model (see Figure 2,
Section 5).
The validation results indicate that the model
captures the key RE artefacts and relationships of a
Big Data software development project, currently
lacking in the literature. The validation results also
confirm consensus amongst the study participants
regarding the usefulness and applicability of the
model in practice (see Table 5, section 4).
This research is not terminal. Further possibilities
include: (1) enhancement of the model embracing
new application domains, such as IoT (internet of
things); (2) empirical studies of the application of the
model in Big Data projects to further assess the
model’s adaptability and generalisability; and (3) cost
analysis of adopting the artefact model in industry
projects.
ACKNOWLEDGEMENTS
This research is supported, in part, by grants from
CNPq, The National Council of Technological and
Scientific Development – Brazil (process:
200218/2015-8) and NSERC, Natural Science and
Engineering Research Council of Canada. We are
very thankful to the industry practitioners that took
part in the validation study. Finally, we are grateful to
the reviewers for their feedback, which has improved
the paper.
REFERENCES
Anderson, K.M., 2015. Embrace the Challenges: Software
Engineering in a Big Data World. In Proc. BIGDSE
2015 1st Int. Work. Big Data Softw. Eng. 19–25.
Arruda, D., Madhavji, N.H., 2018. State of Requirements
Engineering Research in the Context of Big Data
Applications. In Proc. Requir. Eng. Found. Softw.
Qual. Springer Nature.
Arruda, D., Madhavji, N. H., 2017. Towards a
Requirements Engineering Artefact Model in the
context of Big Data Software Development Projects. In
IEEE International Conference on Big Data, pp. 2232–
2237.
Berdie, Douglas R., Anderson, John F., Nibuhr, Marsha A.
1986. Questionnaires: Design and Use.Scarecrow
Press, Metuchen, NJ, 2
nd
edition.
Berenbach, B., Paulish, D.J., Kazmeier, J., Rudorfer, A.
2009. Soft. & Systems Requirements Eng. In Practice.
McGraw-Hill, New York.
Davenport. T.H., Bean, R. 2019. Big Companies Are
Embracing Analytics, But Most Still Don’t Have a
Data-Driven Culture (Online). Available at:
https://hbr.org/2018/02/big-companies-are-embracing-
analytics-but-most-still-dont-have-a-data-driven-
culture. Accessed March 20th, 2019.
Dalpiaz, F., Ferrari, A., Franch, X., Palomares, C., 2018.
Natural Language Processing for Requirements
Engineering The Best Is Yet to Come. IEEE Softw. 35,
115–119.
Dipti Kumar, V., Alencar, P., 2016. Software engineering
for Big Data projects: Domains, methodologies and
gaps. In Proc. - 2016 IEEE Int. Conf. Big Data, 2886–
2895.
Geisberger, E., Broy, M., Berenbach, B., Kazmeier, J.,
Paulish, D., Rudorfer, A., 2006. Requirements
Engineering Reference Model (REM).
Gibbs, G.R. 2007. Analyzing qualitative data, Qualitative
research kit, SAGE Publications, Ltd, London,
England.
A Validation Study of a Requirements Engineering Artefact Model for Big Data Software Development Projects
115