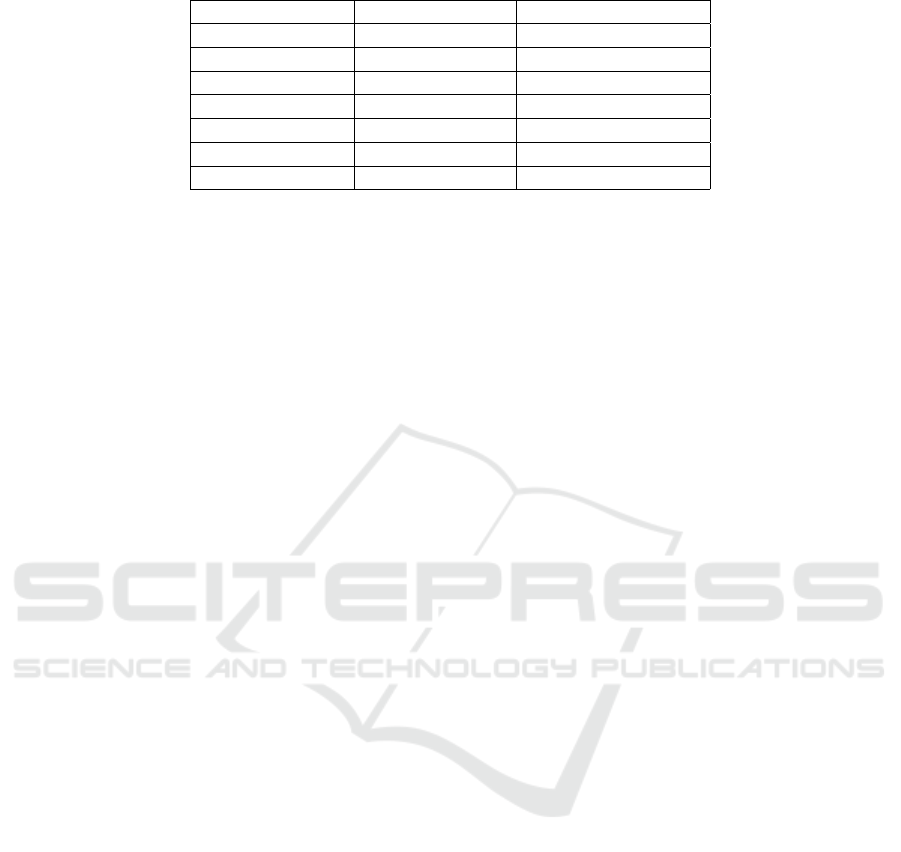
Table 6: Static and dynamic neural model comparison results.
Output parameter Static NN RMSE NARX model RMSE
N
L
0.01120 0.00098
N
I
0.00190 0.00039
N
H
0.00150 0.00047
POWER 0.21890 0.31890
TGT 0.00530 0.00170
Overall RMSE 0.23880 0.32250
Training time 25.404 sec 1333.9 sec
networks. The output data from this program were
recorded in a matrix form. After that, analysis of these
recorded data was performed by dividing the data into
different groups and selection of the best model struc-
ture from each group based on the minimum value
of RMSE. Finally, comparison results between static
NN and dynamic NN showed a good capability of the
dynamic neural networks over the static neural net-
works in representation of the dynamic response of
ADGTE. However, the training time of the dynamic
NN was higher than that in the static NN.
Since, there is no general methodology or rule to
define the neural network parameters, the way to se-
lect the best network configuration is traditionally ob-
tained by trial-and-error. This paper may work as
a guideline for researchers in selection of the best
feed forward and NARX neural network configura-
tion which can represent ADGTE during its full range
above its idle status.
In general, the developed NN models for the
ADGTE can be an effective tool for real time simu-
lation of gas turbines and model based control appli-
cations.
REFERENCES
Asgari, H., Chen, X., Menhaj, M. B., and Sainudiin, R.
(2013). Artificial neural network–based system identi-
fication for a single-shaft gas turbine. Journal of Engi-
neering for Gas Turbines and Power, 135(9):092601.
Asgari, H., Chen, X., Morini, M., Pinelli, M., Sainudiin,
R., Spina, P. R., and Venturini, M. (2016). Narx
models for simulation of the start-up operation of a
single-shaft gas turbine. Applied Thermal Engineer-
ing, 93:368–376.
Bahlawan, H., Morini, M., Pinelli, M., Spina, P. R., and
Venturini, M. (2017). Development of reliable narx
models of gas turbine cold, warm and hot start-up. In
ASME Turbo Expo 2017: Turbomachinery Technical
Conference and Exposition.
Beale, M. H., Hagan, M. T., and Demuth, H. B. (2015).
Neural network toolbox
TM
user’s guide. In R2015b,
The MathWorks, Inc., 3 Apple Hill Drive Natick, MA
01760-2098, , www.mathworks.com.
Cybenko, G. (1989). Approximation by superpositions of
a sigmoidal function. Mathematics of control, signals
and systems, 2(4):303–314.
Fast, M., Assadi, M., and De, S. (2009). Development and
multi-utility of an ann model for an industrial gas tur-
bine. Applied Energy, 86(1):9–17.
Hanachi, H., Liu, J., Banerjee, A., Chen, Y., and Koul, A.
(2015). A physics-based modeling approach for per-
formance monitoring in gas turbine engines. IEEE
Transactions on Reliability, 64(1):197–205.
Lazzaretto, A. and Toffolo, A. (2001). Analytical and neural
network models for gas turbine design and off-design
simulation. International Journal of Thermodynam-
ics, 4(4):173–182.
Norgaard, M., Ravn, O., Poulsen, N., and Hansen, L.
(2000). Neural networks for modelling and control
of dynamic systems: a practitioner’s handbook. Ad-
vanced textbooks in control and signal processing.
Springer, Berlin.
Rahmoune, M. B., Hafaifa, A., and Guemana, M. (2015).
Neural network monitoring system used for the fre-
quency vibration prediction in gas turbine. In Control,
Engineering & Information Technology (CEIT), 2015
3rd International Conference on, pages 1–5. IEEE.
Salehi, A. and Montazeri-Gh, M. (2018). Black box model-
ing of a turboshaft gas turbine engine fuel control unit
based on neural narx. Proceedings of the Institution of
Mechanical Engineers, Part M: Journal of Engineer-
ing for the Maritime Environment.
Neural Networks Modelling of Aero-derivative Gas Turbine Engine: A Comparison Study
745