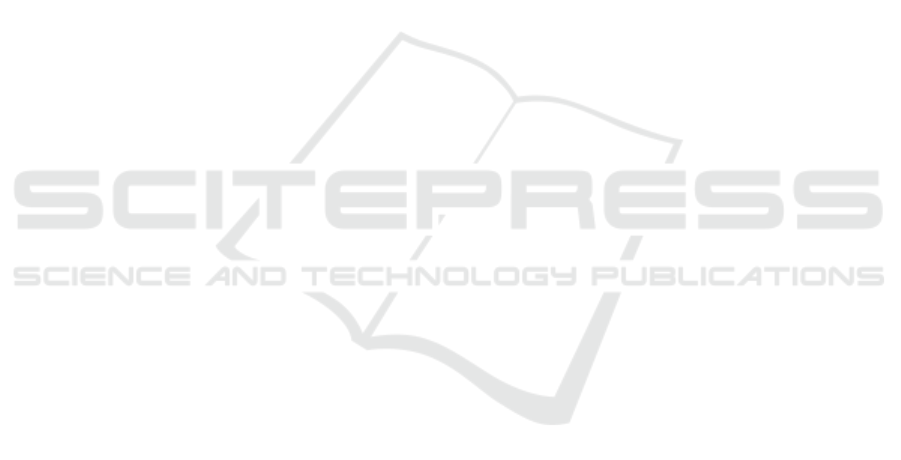
Energy Systems; ZIES) for their contributions to the
work presented in the DKV article.
REFERENCES
Basirat, M. and Roth, P. M. (2018). The quest for the golden
activation function. CoRR, abs/1808.00783.
Bechtler, H., Browne, M., Bansal, P., and Kecman, V.
(2001). Neural networks—a new approach to model
vapour-compression heat pumps. International Jour-
nal of Energy Research, 25(7):591–599.
Becker, M., Lippel, J., and Stuhlsatz, A. (2017). Regular-
ized nonlinear discriminant analysis. an approach to
robust dimensionality reduction for data visualization.
In International Conference on Information Visualiza-
tion Theory and Applications.
Burgu
´
es, J. and Marco, S. (2018). Multivariate estimation
of the limit of detection by orthogonal partial least
squares in temperature-modulated mox sensors. An-
alytica chimica acta, 1019:49–64.
Carriere, A. and Hamam, Y. (1992). Heat pump emula-
tion using neural networks. In [Proceedings 1992]
IEEE International Conference on Systems Engineer-
ing. IEEE.
Duda, Stork, and Hart (2001). Pattern Classification, 2nd
Edition. John Wiley & Sons, Inc.
Erhan, D., Bengio, Y., Courville, A., Manzagol, P.-A.,
and Vincent, P. (2010). Why does unsupervised pre-
training help deep learning? Journal of Machine
Learning Research, 11:625–660.
Frey, P., Ehrismann, B., and Dr
¨
uck, H. (2011). Devel-
opment of artificial neural network models for sorp-
tion chillers. In Proceedings of the ISES Solar World
Congress 2011. International Solar Energy Society.
Gaida, D., Wolf, C., Meyer, C., Stuhlsatz, A., Lippel, J.,
B
¨
ack, T., Bongards, M., and McLoone, S. (2012).
State estimation for anaerobic digesters using the
ADM1. 66(5):1088.
Goebel, J., Kowalski, M., Frank, L., and Adam, M. (2015).
Rechnersimulationen zum winter- und sommerbetrieb
einer abwasser-gasw
¨
armepumpe/-k
¨
altemaschine. In
Deutsche K
¨
alte- und Klimatagung.
Kose, A. and Petlenkov, E. (2016). System identification
models and using neural networks for ground source
heat pump with ground temperature modeling. In
Neural Networks (IJCNN), 2016 International Joint
Conference on, pages 2850–2855. IEEE.
Le Guennec, A., Malinowski, S., and Tavenard, R. (2016).
Data augmentation for time series classification us-
ing convolutional neural networks. In ECML/PKDD
Workshop on Advanced Analytics and Learning on
Temporal Data.
Ledesma, S. and Belman-Flores, J. M. (2016). Analysis
of cop stability in a refrigeration system using artifi-
cial neural networks. In Neural Networks (IJCNN),
2016 International Joint Conference on, pages 558–
565. IEEE.
Lippel, J., Becker, M., Frank, L., Goebel, J., and Zielke,
T. (2017). Modellierung dynamischer prozesse
mit deep neural networks am beispiel einer gas-
absorptionsw
¨
armepumpe. In Deutsche K
¨
alte- und Kli-
matagung 2017.
Maciejowski, J. (1996). Parameter estimation of multivari-
able systems using balanced realizations. NATO ASI
SERIES F COMPUTER AND SYSTEMS SCIENCES,
153:70–119.
Reich, M., Adam, M., and Lambach, S. (2017). Comparison
of different methods for approximating models of en-
ergy supply systems and polyoptimising the systems-
structure and components-dimension. In ECOS 2017
(30th International Conference on Efficiency, Cost,
Optimisation, Simulation and Environmental Impact
of Energy Systems).
Sak, H., Senior, A., and Beaufays, F. (2014). Long short-
term memory recurrent neural network architectures
for large scale acoustic modeling. Proceedings of the
Annual Conference of the International Speech Com-
munication Association, INTERSPEECH, pages 338–
342.
Shen, C., Yang, L., Wang, X., Jiang, Y., and Yao, Y.
(2015). Predictive performance of a wastewater
source heat pump using artificial neural networks.
Building Services Engineering Research and Technol-
ogy, 36(3):331–342.
Stuhlsatz, A., Lippel, J., and Zielke, T. (2010). Discrim-
inative feature extraction with deep neural networks.
In The 2010 International Joint Conference on Neu-
ral Networks (IJCNN). Institute of Electrical and Elec-
tronics Engineers (IEEE).
Stuhlsatz, A., Lippel, J., and Zielke, T. (2012). Feature ex-
traction with deep neural networks by a generalized
discriminant analysis. 23(4):596–608.
Tetko, I. V., Livingstone, D. J., and Luik, A. I. (1995). Neu-
ral network studies. 1. comparison of overfitting and
overtraining. Journal of chemical information and
computer sciences, 35(5):826–833.
Tsungnan Lin, Horne, B. G., Tino, P., and Giles, C. L.
(1996). Learning long-term dependencies in narx re-
current neural networks. IEEE Transactions on Neu-
ral Networks, 7(6):1329–1338.
Wang, G., Zhang, Y., Wang, R., and Han, G. (2013). Perfor-
mance prediction of ground-coupled heat pump sys-
tem using nnca-rbf neural networks. In Control and
Decision Conference (CCDC), 2013 25th Chinese,
pages 2164–2169. IEEE.
Zhu, Y., van Overschee, P., de Moor, B., and Ljung, L.
(1994). Comparison of three classes of identification
methods. IFAC Proceedings Volumes, 27(8):169–174.
SIMULTECH 2019 - 9th International Conference on Simulation and Modeling Methodologies, Technologies and Applications
326