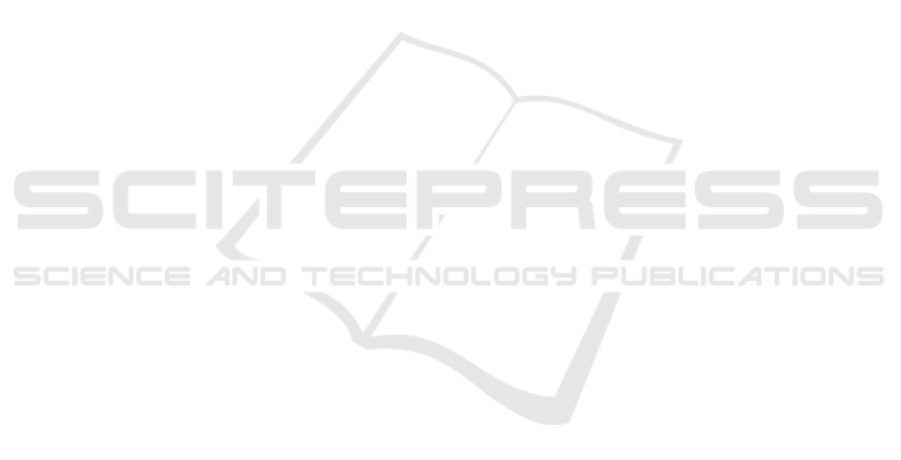
level of concentration of the user at the time of doing
the test, or that the user has more facility to visualize
imagined objects.
5 CONCLUSIONS
In this work we have demonstrated the possibility of
using visual imagination for the construction of BCI
systems, specifically using the imagination of simple
geometrical figures. A methodology for this task has
also been presented using the CSP filter together with
the calculation of the variance of the transformations
made with the CSP filter.
As future work it would be interesting to register
more people, study what frequency range and which
electrodes are better to perform the classification of
the geometrical figures, and study the impact of the
different geometrical shapes in the classification.
ACKNOWLEDGMENTS
The authors would like to thank the people who have
volunteered to make the EEG records, without them this
study would not have been possible. This work was par-
tially funded by the project TIN2017-88515-C2-2-R from
Ministerio de Ciencia, Inovaci
´
on y Universidades, Spain.
REFERENCES
Abiyev, R., Akkaya, N., Aytac, E., Gunsel, I., and C¸ agman,
A. (2016). Brain-computer interface for control of
wheelchair using fuzzy neural networks. BioMed Re-
search International, 2016, 1–9.
Bobrov, P., Frolov, A., Cantor, C., Fedulova, I., Bakhnyan,
M., and Zhavoronkov, A. (2011). Brain-computer in-
terface based on generation of visual images. PLoS
ONE, 6(6).
Chen, X., Wang, Y., Nakanishi, M., Gao, X., Jung, and
(2015), S. G. (2015). High-speed spelling with
a noninvasive brain–computer interface. Proceed-
ings of the National Academy of Sciences, 112(44),
E6058–E6067.
DaSalla, C., Kambara, H., Sato, M., and Koike, Y. (2009).
Single-trial classification of vowel speech imagery us-
ing common spatial patterns. Neural Networks 22
(2009) 1334–1339.
DaSalla, C., Sato, M., and Koike, Y. (2007). Emg vowel
recognition using a support vector machine. Interna-
tional symposium on measurement, analysis and mod-
eling of human functions (pp. 227–232).
Edlinger, G., Holzner, C., and (2011), C. G. (2011). A
hybrid brain-computer interface for smart home con-
trol. Lecture Notes in Computer Science, vol 6762.
Springer, Berlin, Heidelberg.
Ferreira, A., Bastos-Filho, T., Sarcinelli-Filho, M., Mart
´
ın-
S
´
anchez, J., Garc
´
ıa-Garc
´
ıa, J., and (2010), M. M.-Q.
(2010). Improvements of a brain–computer interface
applied to a robotic wheelchair. Communications in
Computer and Information Science 52 (2010) 64–73.
Gal
´
an, F., Nuttin, M., Lew, E., P.W. Ferrez, G. V.,
Philips, J., and (2008), J. M. (2008). A brain-
actuated wheelchair: asynchronous and non-invasive
brain–computer interfaces for continuous control
of robots. Clinical Neurophysiology 119 (2008)
2159–2169.
Guger, C., Harkam, W., Hertnaes, C., and Pfurtscheller, G.
(2002). Prosthetic control by an eeg-based brain- com-
puter interface (bci). AAATE 5th European Confer-
ence for the Advancement of Assistive Technology.
Lo, M., P.Huang, Tsai, Tsai, P. H., Lin, P., and Yue-Loong,
H. (2009). The nonlinear and nonstationary properties
in eeg signals: Probing the complex fluctuations by
hilbert-huang transform. Advances in Adaptive Data
Analysis 1(03):461-482.
Lotte, F., Bougrain, L., Cichocki, A., Clerc, M., Congedo,
M., Rakotomamonjy, A., and (2018), F. Y. (2018).
A review of classification algorithms for EEG-based
brain–computer interfaces: a 10 year update. Jour-
nal of Neural Engineering, 15(3), 031005, (November
2012).
Mariko, M. and Junichi, H. (2014). Classification of silent
speech using support vector machine and relevance
vector machine. Applied Soft Computing Volume 20,
pp 95-102.
Matlab (2016). version 9.0.0 (R2016a). The MathWorks
Inc., Natick, Massachusetts.
Mensh, B., Werfel, J., and Seung, H. (2004). Bci com-
petition 2003-data set ia: Combining gamma-band
power with slow cortical potentials to improve single-
trial classification of electroencephalographic signals.
IEEE Trans. Biomed. Eng., vol. 51, no. 6, pp. 1052–
1056.
Ramoser, H., M
¨
uller-Gerking, J., and Pfurtscheller, G.
(2000). Optimal spatial filtering of single trial eeg dur-
ing imagined hand movement. EEE Transactions on
Rehabilitation Engineering.
Singla, R. and Haseena, B. (2013). Bci based wheelchair
control using steady state visual evoked potentials and
support vector machines. International Journal of Soft
Computing and Engineering (IJSCE).
Song, X., Xie, S., and Meng, W. (2017). Signal process-
ing methods for ssvep-based bcis. Biomechatronics in
Medical Rehabilitation pp 53-70.
Teukolsky, S. and Vetterling, W. (2007). Radial Basis Func-
tion Interpolation.
Trunk (1979). A problem of dimensionality: A simple ex-
ample. IEEE Transactions on Pattern Analysis and
Machine Intelligence.
Wang, Y., Gao, S., and Gao, X. (2005). Common spatial
pattern method for channel selelction in motor im-
agery based brain-computer interface. IEEE Engi-
neering in Medicine and Biology 27th Annual Con-
ference.
Common Spatial Pattern for the Classification of Imagined Geometric Objects
155