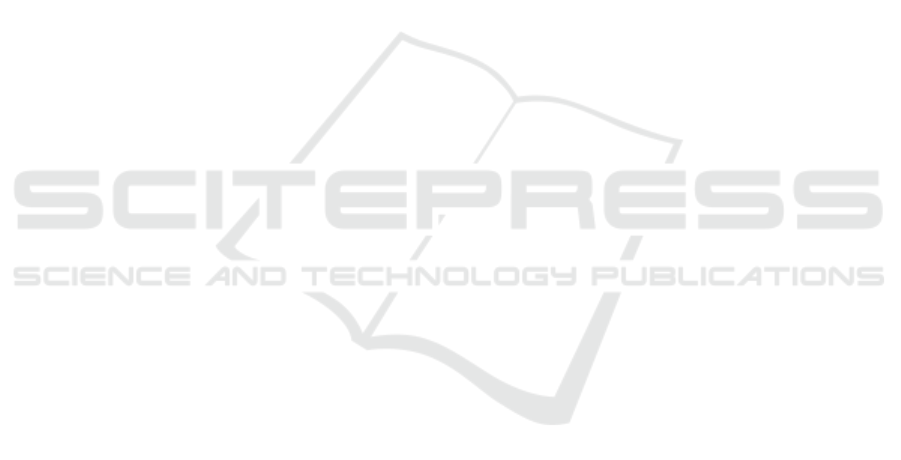
candidates, frequent failures. Alzheimer’s research &
therapy, 6(4):37.
Dipnall, J. F., Pasco, J. A., Berk, M., Williams, L. J., Dodd,
S., Jacka, F. N., and Meyer, D. (2016). Fusing data
mining, machine learning and traditional statistics to
detect biomarkers associated with depression. PloS one,
11(2): e0148195.
Eapen, A. G. (2004). Application of data mining in medical
applications. Master’s thesis, University of Waterloo.
Gamberger, D., Lavraˇc, N., Srivatsa, S., Tanzi, R. E., and
Doraiswamy, P. M. (2017). Identification of clusters of
rapid and slow decliners among subjects at risk for
alzheimer’s disease. Scientific reports, 7(1):6763.
Guisan, A., Edwards Jr, T. C., and Hastie, T. (2002).
Generalized linear and generalized additive models in
studies of species distributions: setting the scene.
Ecological modelling, 157(2-3):89–100.
Levine, S., Pastor, P., Krizhevsky, A., Ibarz, J., and Quillen,
D. (2018). Learning hand-eye coordination for robotic
grasping with deep learning and large-scale data
collection. The International Journal of Robotics
Research, 37(4-5):421–436.
Ni, H., Yang, X., Fang, C., Guo, Y., Xu, M., and He, Y.
(2014). Data mining-based study on sub-mentally
healthy state among residents in eight provinces and
cities in china. Journal of Traditional Chinese
Medicine, 34(4):511–517.
Scatena, R., Martorana, G. E., Bottoni, P., Botta, G.,
Pastore, P., and Giardina, B. (2007). An update on
pharmacological approaches to neurodegenerative
diseases. Expert opinion on investigational drugs,
16(1):59–72.
Shahbaz, M., Guergachi, A., Noreen, A., and Shaheen, M.
(2013). A data mining approach to recognize objects in
satellite images to predict natural resources. In IAENG
Transactions on Engineering Technologies, pages 215–
230. Springer.
Small, D. H. (2005). Acetylcholinesterase inhibitors for the
treatment of dementia in alzheimer’s disease: do we
need new inhibitors? Expert opinion on emerging
drugs, 10(4):817–825.
Soni, N. and Gandhi, C. (2010). Application of data mining
to health care. International Journal of Computer
Science and its Applications.
Stepanova, D., Gad-Elrab, M. H., and Ho, V. T. (2018).
Rule induction and reasoning over knowledge graphs.
In Reasoning Web International Summer School, pages
142–172. Springer.
Sumathi, S. and Sivanandam, S. (2006). Introduction to
data mining principles. Introduction to data mining and
its applications, pages 1–20.
Thenmozhi, K. and Deepika, P. (2014). Heart disease
prediction using classification with different decision
tree techniques. International Journal of Engineering
Research and General Science, 2(6):6–11.
Thomas, J. and Princy, R. T. (2016). Human heart disease
prediction system using data mining techniques. In
2016 International Conference on Circuit, Power and
Computing Technologies (ICCPCT), pages 1–5. IEEE.
Touhy, T. A., Jett, K. F., Boscart, V., and McCleary, L.
(2014). Ebersole and Hess’ Gerontological Nursing and
Healthy Aging, Canadian Edition-E-Book. Elsevier
Health Sciences.
Unay, D., Ekin, A., and Jasinschi, R. S. (2010). Local
structure-based region-of-interest retrieval in brain mr
images. IEEE Transactions on Information technology
in Biomedicine, 14(4):897–903.
Weiner, M. W., Veitch, D. P., Aisen, P. S., Beckett, L. A.,
Cairns, N. J., Green, R. C., Harvey, D., Jack, C. R.,
Jagust, W., Liu, E., et al. (2013). The alzheimer’s
disease neuroimaging initiative: a review of papers
published since its inception. Alzheimer’s & Dementia,
9(5):e111–e194.
Wolfson, C., Wolfson, D. B., Asgharian, M., M’lan, C. E.,
Østbye, T., Rockwood, K., and Hogan, D. f. (2001). A
reevaluation of the duration of survival after the onset
of dementia. New England Journal of Medicine,
344(15):1111–1116.
Yang, J., Li, J., and Xu, Q. (2018). A highly efficient big
data mining algorithm based on stock market.
International Journal of Grid and High Performance
Computing (IJGHPC), 10(2):14–33.
Zhang, M.-L. and Zhou, Z.-H. (2005). A k-nearest
neighbour based algorithm for multi-label
classification. In Granular Computing, 2005 IEEE
International Conference on, volume 2, pages 718–721.
IEEE.
APPENDIX
Decision Tree Model
CDRSB_bl>0.250
| CDRSB_bl>2.750
| | MMSE_bl>26.500
| | | AGE>75.300: LMCI {AD=0, CN=0, EMCI=0,
LMCI=13, SMC=0}
| | | AGE≤75.300: EMCI {AD=0, CN=0, EMCI=8,
LMCI=0, SMC=0}
| | MMSE_bl≤26.500: AD {AD=254, CN=0,
EMCI=5, LMCI=0, SMC=0}
| CDRSB_bl≤2.750
| | MMSE_bl>23.500
| | | RAVLT_perc_forgetting_bl>-9.167
| | | | AGE>88.850: AD {AD=5, CN=0, EMCI=0,
LMCI=0, SMC=0}
| | | | AGE≤88.850
| | | | | Fusiform_bl>23133.500
| | | | | | PTETHCAT = Hisp/Latino: EMCI
{AD=0, CN=0, EMCI=2, LMCI=0, SMC=0}
| | | | | | PTETHCAT = Not Hisp/Latino: SMC
{AD=0, CN=0, EMCI=0, LMCI=0, SMC=5}
| | | | | Fusiform_bl≤23133.500
| | | | | | Ventricles_bl>9770.500
| | | | | | | ICV_bl>1268370
DATA 2019 - 8th International Conference on Data Science, Technology and Applications
302