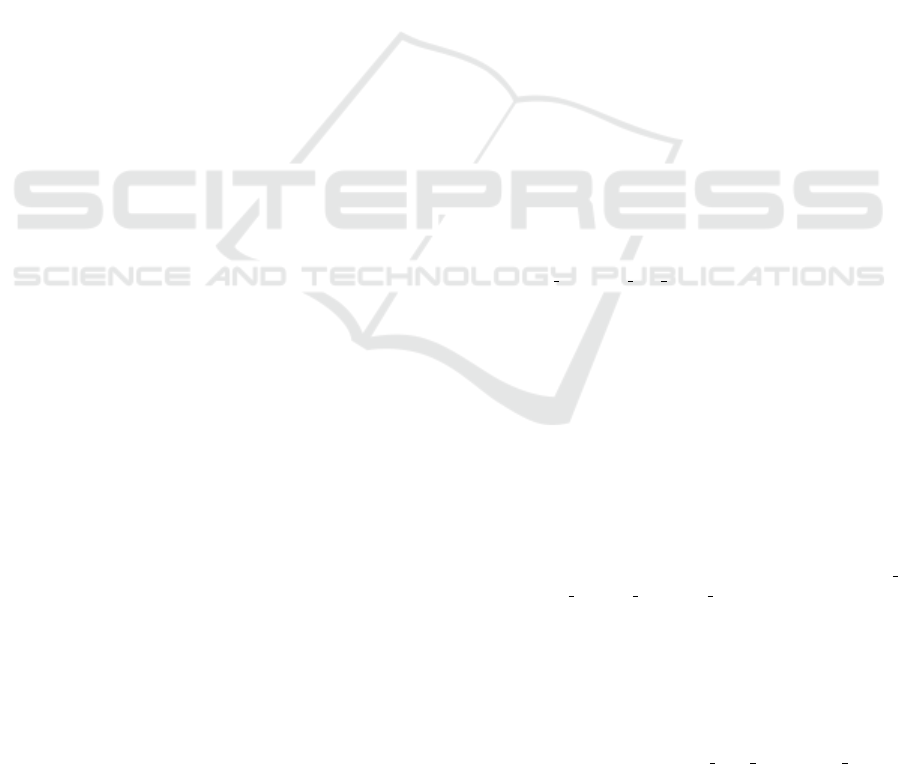
and Zdonik that defines eight requirements for real-
time stream processing. Particularly, these are the
need (1) to keep the data moving, (2) for a stream-
ing SQL language as highlighted before, (3) for the
ability to handle stream imperfections, (4) to generate
predictable outcomes, and (5) to integrate stored as
well as streaming data, which fits to the Industry 4.0
scenarios where business (stored) and IoT (streaming)
data are integrated. Furthermore, the requirement (6)
to ensure data safety and availability, (7) to automati-
cally scale and partition programs, and (8) to process
and respond immediately are highlighted.
6 CONCLUSION
The present paper pictures a point of view on Indus-
try 4.0 and on data stream processing systems in its
context. Thereby, contributions are threefold. First,
we present insights about current situations and opin-
ions at two selected companies with respect to Indus-
try 4.0. This includes information about data char-
acteristics and Industry 4.0 applications. All findings
were derived from site inspections and alike.
Secondly, a viewpoint on Industry 4.0 as well as
on further important and closely related aspects is
given. Among others, it ensures a common under-
standing needed for the third contribution.
This third part is about data stream processing sys-
tems. Particularly, it is about why and how this tech-
nology could become an enabler for Industry 4.0. A
possible architecture for Industry 4.0 scenarios is pro-
posed and obstacles hindering DSPSs from being ap-
plied more in this context are pointed out.
REFERENCES
Arasu, A., Cherniack, M., Galvez, E. F., Maier, D., Maskey,
A., Ryvkina, E., Stonebraker, M., and Tibbetts, R.
(2004). Linear Road: A Stream Data Management
Benchmark. In (e)Proc. International Conference on
Very Large Data Bases, pages 480–491.
Ashton, K. et al. (2009). That ’Internet of Things’ Thing.
RFID journal, 22(7):97–114.
Bauer, W., Pokorni, B., and Findeisen, S. (2018). Produc-
tion Assessment 4.0 – Methods for the Development
and Evaluation of Industry 4.0 Use Cases. In Inter-
national Conference on Applied Human Factors and
Ergonomics, pages 501–510. Springer.
Christensen, C. M. (2013). THE INNOVATOR’S DILEMMA
- WHEN NEW TECHNOLOGIES CAUSE GREAT
FIRMS TO FAIL//. Harvard Business Review Press.
D
¨
ohler (2019). About D
¨
ohler — Who we are. https://www.
doehler.com/en/about-doehler/who-we-are.html. Ac-
cessed: 2019-04-03.
Hankel, M. and Rexroth, B. (2015). Industrie 4.0: The Ref-
erence Architectural Model Industrie 4.0 (RAMI 4.0).
ZVEI, 2:2.
Hermann, M., Pentek, T., and Otto, B. (2016). Design Prin-
ciples for Industrie 4.0 Scenarios. In Hawaii Interna-
tional Conference on System Sciences, HICSS, pages
3928–3937.
Hesse, G. and Lorenz, M. (2015). Conceptual Survey on
Data Stream Processing Systems. In IEEE Interna-
tional Conference on Parallel and Distributed Sys-
tems, ICPADS 2015, pages 797–802.
Hesse, G., Matthies, C., Glass, K., Huegle, J., and Uflacker,
M. (2019). Quantitative Impact Evaluation of an Ab-
straction Layer for Data Stream Processing Systems.
In International Conference on Distributed Comput-
ing Systems, ICDCS.
Hesse, G., Matthies, C., Reissaus, B., and Uflacker, M.
(2017a). A New Application Benchmark for Data
Stream Processing Architectures in an Enterprise
Context: Doctoral Symposium. In ACM International
Conference on Distributed and Event-based Systems
(DEBS), pages 359–362.
Hesse, G., Reissaus, B., Matthies, C., Lorenz, M., Kraus,
M., and Uflacker, M. (2017b). Senska - Towards an
Enterprise Streaming Benchmark. In TPC Technology
Conference, TPCTC, pages 25–40.
Intel (2014). A GUIDE TO THE INTERNET OF
THINGS. https://www.intel.com/content/www/us/
en/internet-of-things/infographics/guide-to-iot.html.
Accessed: 2019-04-03.
McKinsey&Company (2015). Industry 4.0 - How
to navigate digitization of the manufacturing sec-
tor. http://www.forschungsnetzwerk.at/downloadpub/
mck\ industry\ 40\ report.pdf. Accessed: 2019-04-
02.
McKinsey&Company (2016). Industry 4.0 after the ini-
tial hype - Where manufacturers are finding value and
how they can best capture it.
Miorandi, D., Sicari, S., Pellegrini, F. D., and Chlamtac, I.
(2012). Internet of things: Vision, applications and
research challenges. Ad Hoc Networks, 10(7):1497–
1516.
Mrugalska, B. and Wyrwicka, M. K. (2017). Towards Lean
Production in Industry 4.0. Procedia Engineering,
182:466 – 473.
SAP (2018). Cloud Experts Tell It Like It Is - D
¨
ohler’s
Best Practices on Setting Up a Hybrid IT Land-
scape. https://d.dam.sap.com/m/xmxUAO/Cloud
Experts Doehler Interview Brochure-V03.pdf. Ac-
cessed: 2019-04-03.
Stonebraker, M., C¸ etintemel, U., and Zdonik, S. B. (2005).
The 8 Requirements of Real-Time Stream Processing.
SIGMOD Record, 34(4):42–47.
Weiner, S. and Line, D. (2014). Manufacturing and the data
conundrum - Too much? Too little? Or just right?
https://www.eiuperspectives.economist.com/sites/
default/files/Manufacturing Data Conundrum Jul14.
pdf. Accessed: 2019-04-02.
DATA 2019 - 8th International Conference on Data Science, Technology and Applications
310