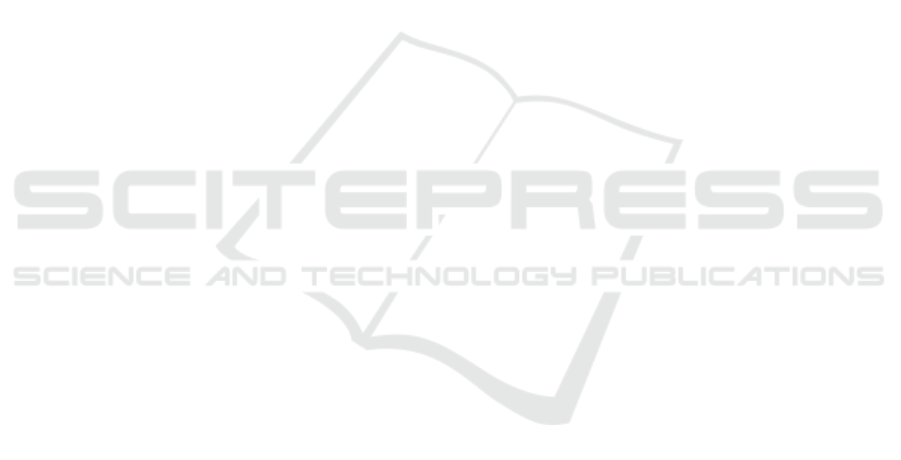
Lin, Y., Liu, Z., Luan, H., Sun, M., Rao, S., and Liu, S.
(2015). Modeling Relation Paths for Representation
Learning of Knowledge Bases.
Ma, Y., Peng, H., and Cambria, E. (2018). Targeted aspect-
based sentiment analysis via embedding common-
sense knowledge into an attentive LSTM. In Thirty-
Second AAAI Conference on Artificial Intelligence.
Mohamed, O. A., Masood, S. H., and Bhowmik, J. L.
(2015). Optimization of fused deposition model-
ing process parameters: a review of current research
and future prospects. Advances in Manufacturing,
3(1):42–53.
Nguyen, D. Q., Nguyen, T. D., Nguyen, D. Q., and Phung,
D. (2018). A Novel Embedding Model for Knowl-
edge Base Completion Based on Convolutional Neu-
ral Network. In Proceedings of the 2018 Conference
of the North American Chapter of the Association for
Computational Linguistics: Human Language Tech-
nologies, volume 2, pages 327–333.
Nickel, M., Murphy, K., Tresp, V., and Gabrilovich, E.
(2016). A review of relational machine learning for
knowledge graphs: From Multi-Relational Link Pre-
diction to Automated Knowledge Graph Construction.
Proceedings of the IEEE, 104(1):11–33.
Socher, R., Chen, D., Manning, C. D., and Ng, A. Y. (2013).
Reasoning With Neural Tensor Networks for Knowl-
edge Base Completion. In NIPS.
Stein, A., Maier, R., and H
¨
ahner, J. (2017a). Toward Cu-
rious Learning Classifier Systems: Combining XCS
with Active Learning Concepts. In Proceedings of the
Genetic and Evolutionary Computation Conference
Companion, GECCO ’17, pages 1349–1356, New
York, NY, USA. ACM.
Stein, A., Rauh, D., Tomforde, S., and H
¨
ahner, J. (2016).
Architecture of Computing Systems – ARCS 2016:
29th International Conference, Nuremberg, Germany,
April 4-7, 2016, Proceedings, chapter Augmenting the
Algorithmic Structure of XCS by Means of Interpola-
tion, pages 348–360. Springer International Publish-
ing, Cham.
Stein, A., Rauh, D., Tomforde, S., and H
¨
ahner, J. (2017b).
Interpolation in the eXtended Classifier System: An
Architectural Perspective. Journal of Systems Archi-
tecture, pages 79–94.
Stein, A., Tomforde, S., Diaconescu, A., H
¨
ahner, J., and
M
¨
uller-Schloer, C. (2018). A Concept for Proac-
tive Knowledge Construction in Self-Learning Au-
tonomous Systems. In 2018 IEEE 3rd International
Workshops on Foundations and Applications of Self*
Systems (FAS*W), pages 204–213.
Sun, Z., Yang, J., Zhang, J., Bozzon, A., Huang, L.-K., and
Xu, C. (2018). Recurrent knowledge graph embed-
ding for effective recommendation. In Proceedings of
the 12th ACM Conference on Recommender Systems,
pages 297–305. ACM.
Sutton, R. S., McAllester, D. A., Singh, S. P., and Man-
sour, Y. (2000). Policy Gradient Methods for Rein-
forcement Learning with Function Approximation. In
Solla, S. A., Leen, T. K., and M
¨
uller, K., editors, Ad-
vances in Neural Information Processing Systems 12,
pages 1057–1063. MIT Press.
Tandon, N., Varde, A. S., and de Melo, G. (2018). Com-
monsense knowledge in machine intelligence. ACM
SIGMOD Record, 46(4):49–52.
Urbanowicz, R. J., Granizo-Mackenzie, D., and Moore,
J. H. (2012). Using Expert Knowledge to Guide
Covering and Mutation in a Michigan Style Learn-
ing Classifier System to Detect Epistasis and Hetero-
geneity, pages 266–275. Springer Berlin Heidelberg,
Berlin, Heidelberg.
VDW (1997). Abteilungs
¨
ubergreifende Projektierung kom-
plexer Maschinen und Anlagen. VDW-Bericht.
Wang, H., Zhang, F., Xie, X., and Guo, M. (2018). Dkn:
Deep knowledge-aware network for news recommen-
dation. In Proceedings of the 2018 World Wide Web
Conference on World Wide Web, pages 1835–1844.
International World Wide Web Conferences Steering
Committee.
Wang, J., Wang, Z., Zhang, D., and Yan, J. (2017). Combin-
ing Knowledge with Deep Convolutional Neural Net-
works for Short Text Classification. In IJCAI, pages
2915–2921.
Wilson, S. W. (1995). Classifier Fitness Based on Accuracy.
In Evol. Comput. 3, page 149–175.
Wilson, S. W. (2000). Get Real! XCS with Continuous-
Valued Inputs. In Learning Classifier Systems, page
209–219. Springer Berlin Heidelberg,.
Wohlers, T. and Gornet, T. (2014). History of additive man-
ufacturing. Wohlers report, 24(2014):118.
Yang, B. and Mitchell, T. (2019). Leveraging knowl-
edge bases in LSTMs for improving machine reading.
arXiv preprint arXiv:1902.09091.
Towards Automated Parameter Optimisation of Machinery by Persisting Expert Knowledge
413