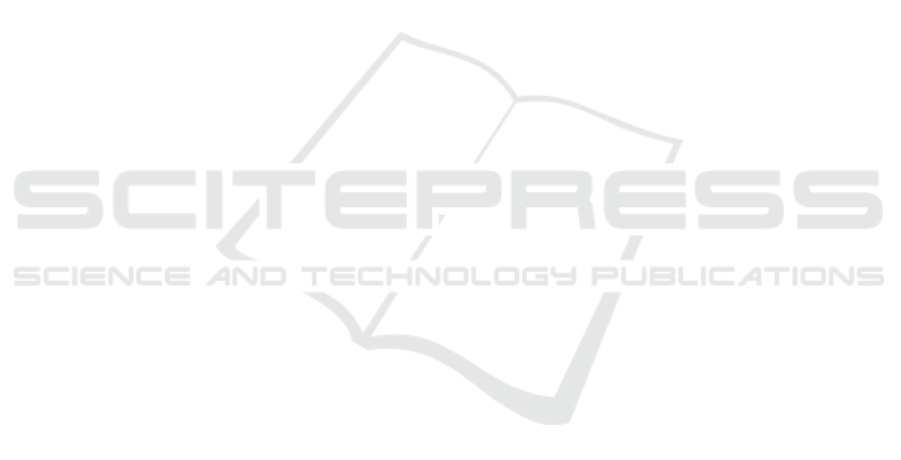
showed that the combination of AI and classic ap-
proach can make a good tool for designing effective
solutions for autonomous vehicle control, where addi-
tional information on the feasibility and stability can
be acquired.
The algorithm shows convergence during the
learning hence, the trained agent is basically capable
to generate effective trajectories. The visual inspec-
tion of the situations shows that the overall behavior
of the agent fulfills the requirements.
Further research will focus on extending the envi-
ronment with a variable speed solution and real-world
tests.
ACKNOWLEDGEMENTS
The research reported in this paper was supported by
the Higher Education Excellence Program of the Min-
istry of Human Capacities in the frame of Artificial
Intelligence research area of Budapest University of
Technology and Economics (BME FIKPMI/FM).
EFOP-3.6.3-VEKOP-16-2017-00001: Tal-
ent management in autonomous vehicle control
technologies- The Project is supported by the Hun-
garian Government and co-financed by the European
Social Fund. Supported by The UNKP-18-3 New
National Excellence Program of The Ministry of
Human Capacities
REFERENCES
Aradi, S., Becsi, T., and Gaspar, P. (2018). Policy gra-
dient based reinforcement learning approach for au-
tonomous highway driving. In 2018 IEEE Conference
on Control Technology and Applications (CCTA),
pages 670–675. IEEE.
B
´
ecsi, T., Aradi, S., Feh
´
er,
´
A., Szalay, J., and G
´
asp
´
ar, P.
(2018). Highway environment model for reinforce-
ment learning. IFAC-PapersOnLine, 51(22):429–434.
Chen, Y. F., Everett, M., Liu, M., and How, J. P. (2017). So-
cially aware motion planning with deep reinforcement
learning. In 2017 IEEE/RSJ International Confer-
ence on Intelligent Robots and Systems (IROS), pages
1343–1350. IEEE.
Feh
´
er,
´
A., Aradi, S., and B
´
ecsi, T. (2018). Q-learning based
reinforcement learning approach for lane keeping. In
2018 IEEE 18th International Symposium on Compu-
tational Intelligence and Informatics (CINTI). IEEE.
Gammell, J. D., Srinivasa, S. S., and Barfoot, T. D. (2015).
Batch informed trees (bit*): Sampling-based optimal
planning via the heuristically guided search of im-
plicit random geometric graphs. In 2015 IEEE In-
ternational Conference on Robotics and Automation
(ICRA), pages 3067–3074. IEEE.
Heged
¨
us, F., B
´
ecsi, T., and Aradi, S. (2017a). Dynamically
feasible trajectory planning for road vehicles in terms
of sensitivity and robustness. Transportation Research
Procedia, 27:799 – 807. 20th EURO Working Group
on Transportation Meeting, EWGT 2017, 4-6 Septem-
ber 2017, Budapest, Hungary.
Heged
¨
us, F., B
´
ecsi, T., Aradi, S., and G
´
ap
´
ar, P. (2017b).
Model based trajectory planning for highly automated
road vehicles. IFAC-PapersOnLine, 50(1):6958 –
6964. 20th IFAC World Congress.
Li, D., Zhao, D., Zhang, Q., and Chen, Y. (2019). Re-
inforcement learning and deep learning based lat-
eral control for autonomous driving [application
notes]. IEEE Computational Intelligence Magazine,
14(2):83–98.
Li, X., Sun, Z., He, Z., Zhu, Q., and Liu, D. (2015). A prac-
tical trajectory planning framework for autonomous
ground vehicles driving in urban environments. In
2015 IEEE Intelligent Vehicles Symposium (IV), pages
1160–1166.
Lillicrap, T. P., Hunt, J. J., Pritzel, A., Heess, N., Erez, T.,
Tassa, Y., Silver, D., and Wierstra, D. (2015). Contin-
uous control with deep reinforcement learning. arXiv
preprint arXiv:1509.02971.
Liu, L., Hu, J., Wang, Y., and Xie, Z. (2017). Neu-
ral network-based high-accuracy motion control of a
class of torque-controlled motor servo systems with
input saturation. Strojniski Vestnik-Journal of Me-
chanical Engineering, 63(9):519–529.
Minh, V. T. and Pumwa, J. (2014). Feasible path planning
for autonomous vehicles. Mathematical Problems in
Engineering, 2014.
Pacejka, H. B. (2012). Chapter 8 - applications of tran-
sient tire models. In Pacejka, H. B., editor, Tire and
Vehicle Dynamics (Third Edition), pages 355 – 401.
Butterworth-Heinemann, Oxford, 3rd edition edition.
Palmieri, L., Koenig, S., and Arras, K. O. (2016). Rrt-based
nonholonomic motion planning using any-angle path
biasing. In 2016 IEEE International Conference on
Robotics and Automation (ICRA), pages 2775–2781.
IEEE.
Paxton, C., Raman, V., Hager, G. D., and Kobilarov, M.
(2017). Combining neural networks and tree search
for task and motion planning in challenging envi-
ronments. In 2017 IEEE/RSJ International Confer-
ence on Intelligent Robots and Systems (IROS), pages
6059–6066. IEEE.
Plessen, M. G. (2019). Automating vehicles by deep re-
inforcement learning using task separation with hill
climbing. In Future of Information and Communica-
tion Conference, pages 188–210. Springer.
Silver, D., Lever, G., Heess, N., Degris, T., Wierstra, D., and
Riedmiller, M. (2014). Deterministic policy gradient
algorithms. In ICML.
Singh, S., Majumdar, A., Slotine, J.-J., and Pavone, M.
(2017). Robust online motion planning via contrac-
tion theory and convex optimization. In 2017 IEEE
International Conference on Robotics and Automation
(ICRA), pages 5883–5890. IEEE.
ICINCO 2019 - 16th International Conference on Informatics in Control, Automation and Robotics
428