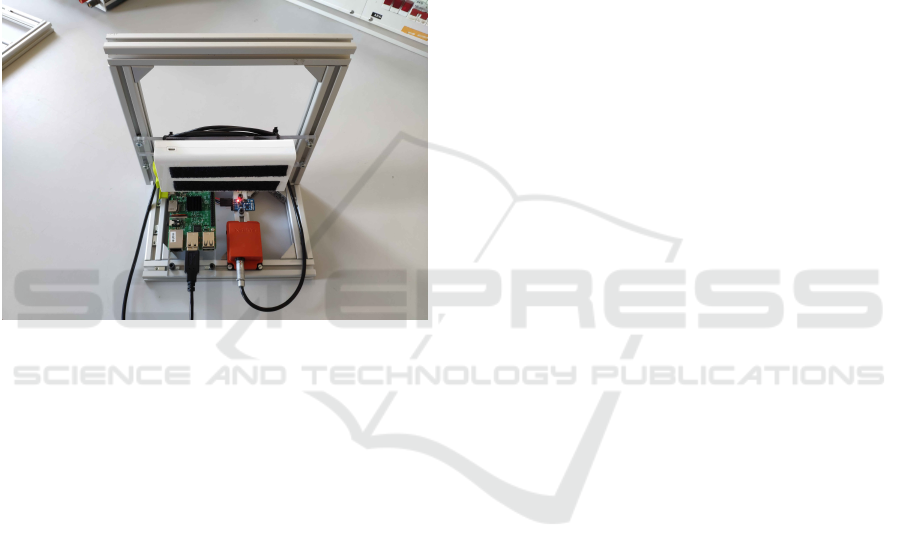
5 CURRENT STATE AND NEXT
STEPS
Currently, the sedan setup is finalized and for the
truck a custom FPGA board is designed. The sen-
sors are being calibrated, starting with the IMU and
with the method proposed by Tedaldi et al. (Tedaldi
et al., 2014). Figure 4 shows the IMU calibration
setup including data logging system and power sup-
ply to be able to carry out different movements with-
out the need for any external cables. For the Sharp
distance sensors an evaluation with a Lidar-generated
map is prepared.
Figure 4: IMU test frame. The orange block is the XSens
reference IMU, the MPU-9255 device under test IMU is
mounted on the small blue printed circuit board.
When the setup has been sufficiently completed, a
feasibility study for using the FPGA-based ML ac-
celerator in combination with a vehicle is planned.
This will include further considerations regarding the
test of traffic sign detection both for a hard-wired net
which has been trained beforehand and a version ac-
tively learning during and improving during the test
phase. This phase should provide insight, e.g., in the
ability of the setup to react to exceptional situations
not provided during the learning phase as well as in
the benefits of capturing exceptional situations during
the driving phase in comparison to simulation-based
generated scenarios. We assume that even in a minia-
ture setup, e.g., the manipulation of light, contrast,
partially hidden signs will be much easier than in sim-
ulations. Furtheron, we will consider if the setup
allows for an automatic optimization of driving be-
haviour under aggrevated circumstances such as take-
over or driving curves in different weather conditions.
6 CONCLUSIONS
There is indication that the use of miniature model
vehicles is a helpful testing means for ML-based au-
tonomous driving solutions – in addition to real-world
tests and simulations. Using miniature model vehi-
cles on a 1:87 scale has some specific advantages over
other common scales, either directly (using off-the-
shelf components) or indirectly (by the need to opti-
mize methods thoroughly to fit to the demanding con-
straints).
First steps were taken to set up a fleet of minia-
ture vehicles that could serve as testing platform for
– specifically ML-based – methods to facilitate au-
tonomous driving. Further implementation and tests
will be carried out to evaluate if miniature autonomy
can be realized and where it is applicable.
REFERENCES
Braunschweig, T. (2019). https://wiki.ifr.ing.tu-
bs.de/carolocup/.
Coulter, R. C. (1992). Implementation of the Pure Pur-
suit Tracking Algorithm. Robotics Institute, Carnegie
Mellon University.
Fuhrmann, J. (2018). Implementierung einer Tensor Pro-
cessing Unit mit dem Fokus auf Embedded Systems
und das Internet of Things. Bachelor Thesis.
Gebhardt, V., Rieger, G. M., Mottok, J., and Gießelbach, C.
(2013). Funktionale Sicherheit nach ISO 26262 - ein
Praxisleitfaden zur Umsetzung. dpunkt.verlag.
Jouppi, N. P., Young, C., Patil, N., Patterson, D., Agrawal,
G., Bajwa, R., Bates, S., Bhatia, S., Boden, N.,
Borchers, A., Boyle, R., Cantin, P., Chao, C., Clark,
C., Coriell, J., Daley, M., Dau, M., Dean, J., Gelb,
B., Ghaemmaghami, T. V., Gottipati, R., Gulland, W.,
Hagmann, R., Ho, C. R., Hogberg, D., Hu, J., Hundt,
R., Hurt, D., Ibarz, J., Jaffey, A., Jaworski, A., Ka-
plan, A., Khaitan, H., Killebrew, D., Koch, A., Ku-
mar, N., Lacy, S., Laudon, J., Law, J., Le, D., Leary,
C., Liu, Z., Lucke, K., Lundin, A., MacKean, G.,
Maggiore, A., Mahony, M., Miller, K., Nagarajan, R.,
Narayanaswami, R., Ni, R., Nix, K., Norrie, T., Omer-
nick, M., Penukonda, N., Phelps, A., Ross, J., Ross,
M., Salek, A., Samadiani, E., Severn, C., Sizikov, G.,
Snelham, M., Souter, J., Steinberg, D., Swing, A.,
Tan, M., Thorson, G., Tian, B., Toma, H., Tuttle, E.,
Vasudevan, V., Walter, R., Wang, W., Wilcox, E., and
Yoon, D. H. (2017). In-datacenter performance anal-
ysis of a tensor processing unit. In 2017 ACM/IEEE
44th Annual International Symposium on Computer
Architecture (ISCA), pages 1–12.
Koopman, P. (2018). Practical experience report: Automo-
tive safety practices vs. accepted principles. In Gal-
lina, B., Skavhaug, A., and Bitsch, F., editors, Com-
puter Safety, Reliability, and Security, pages 3–11,
Cham. Springer International Publishing.
Miniature Autonomy as One Important Testing Means in the Development of Machine Learning Methods for Autonomous Driving: How
ML-based Autonomous Driving could be Realized on a 1:87 Scale
487