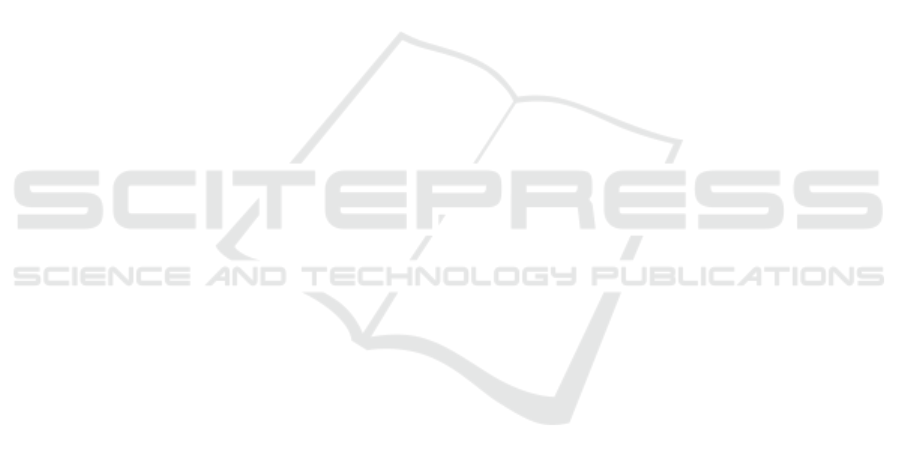
tained an accuracy of 95.2% for coarse grain using
SVM. Moreoever, The hierarchical classifier in (May
and Steinberg, 2004) achieved accuracy of 77.8% us-
ing different classifiers such as SVM, MaxEnt, NB
and Decision Tree. Finally, in (Xu et al., 2016) the
proposed SVM-based approach achieved accuracy of
93.4% using a Bi-Gram mode and SVM kernel func-
tion.
In conclusion, GQCC had a better results than
previous ones due to the ability of our approach to
identify different classes of the factoid question using
domain-specific information which facilitate the iden-
tification of domain categories, unlike previous works
which used additional fifty fine-grained categories.
6 CONCLUSION AND FUTURE
WORK
A framework has been adapted for question catego-
rization and classification. The framework consists of
three main features which are, grammatical features,
domain specific features and patterns. These features
help in utilizing the structure of the questions. In ad-
dition, the performance of different machine learning
algorithms (J48 and SVM) were investigated. The re-
sults show that our solution led to a good performance
in classifying questions compared with the state-of-
arts approaches. As future work, we aim to investigate
the impact of combing different features like seman-
tic, syntactic and lexical attributes and compare the
results. In addition, We are also planning to test other
machine learning algorithms to classify the questions.
REFERENCES
Bu, F., Zhu, X., Hao, Y., and Zhu, X. (2010). Function-
based question classification for general qa. In Pro-
ceedings of the 2010 conference on empirical methods
in natural language processing, pages 1119–1128.
Association for Computational Linguistics.
Bullington, J., Endres, I., and Rahman, M. (2007). Open
ended question classification using support vector ma-
chines. MAICS 2007.
Huang, Z., Thint, M., and Qin, Z. (2008). Question clas-
sification using head words and their hypernyms. In
Proceedings of the Conference on Empirical Methods
in Natural Language Processing, pages 927–936. As-
sociation for Computational Linguistics.
Kolomiyets, O. and Moens, M.-F. (2011). A survey
on question answering technology from an infor-
mation retrieval perspective. Information Sciences,
181(24):5412–5434.
Le-Hong, P., Phan, X.-H., and Nguyen, T.-D. (2015). Us-
ing dependency analysis to improve question classifi-
cation. In Knowledge and Systems Engineering, pages
653–665. Springer.
Li, F., Zhang, X., Yuan, J., and Zhu, X. (2008). Classi-
fying what-type questions by head noun tagging. In
Proceedings of the 22nd International Conference on
Computational Linguistics-Volume 1, pages 481–488.
Association for Computational Linguistics.
Li, X., Huang, X.-J., and WU, L.-d. (2005). Question clas-
sification using multiple classifiers. In Proceedings of
the 5th Workshop on Asian Language Resources and
First Symposium on Asian Language Resources Net-
work.
Li, X. and Roth, D. (2006). Learning question classifiers:
the role of semantic information. Natural Language
Engineering, 12(03):229–249.
May, R. and Steinberg, A. (2004). Al, building a ques-
tion classifier for a trec-style question answering sys-
tem. AL: The Stanford Natural Language Processing
Group, Final Projects.
Metzler, D. and Croft, W. B. (2005). Analysis of statistical
question classification for fact-based questions. Infor-
mation Retrieval, 8(3):481–504.
Mishra, M., Mishra, V. K., and Sharma, H. (2013). Ques-
tion classification using semantic, syntactic and lexi-
cal features. International Journal of Web & Semantic
Technology, 4(3):39.
Mohasseb, A., Bader-El-Den, M., and Cocea, M. (2018).
Question categorization and classification using gram-
mar based approach. Information Processing & Man-
agement.
Mohasseb, A., El-Sayed, M., and Mahar, K. (2014). Auto-
mated identification of web queries using search type
patterns. In WEBIST (2), pages 295–304.
Moldovan, D., Pas¸ca, M., Harabagiu, S., and Surdeanu, M.
(2003). Performance issues and error analysis in an
open-domain question answering system. ACM Trans-
actions on Information Systems (TOIS), 21(2):133–
154.
Nguyen, T. T. and Nguyen, L. M. (2007). Improving the ac-
curacy of question classification with machine learn-
ing. In Research, Innovation and Vision for the Fu-
ture, 2007 IEEE International Conference on, pages
234–241. IEEE.
Nguyen, T. T., Nguyen, L. M., and Shimazu, A. (2008).
Using semi-supervised learning for question classifi-
cation. In Information and Media Technologies, vol-
ume 3, pages 112–130. Information and Media Tech-
nologies Editorial Board.
Van-Tu, N. and Anh-Cuong, L. (2016). Improving ques-
tion classification by feature extraction and selection.
Indian Journal of Science and Technology, 9(17).
Xu, S., Cheng, G., and Kong, F. (2016). Research on ques-
tion classification for automatic question answering.
In Asian Language Processing (IALP), 2016 Interna-
tional Conference on, pages 218–221. IEEE.
Zhang, D. and Lee, W. S. (2003). Question classification
using support vector machines. In Proceedings of
the 26th annual international ACM SIGIR conference
on Research and development in informaion retrieval,
pages 26–32. ACM.
WEBIST 2019 - 15th International Conference on Web Information Systems and Technologies
184