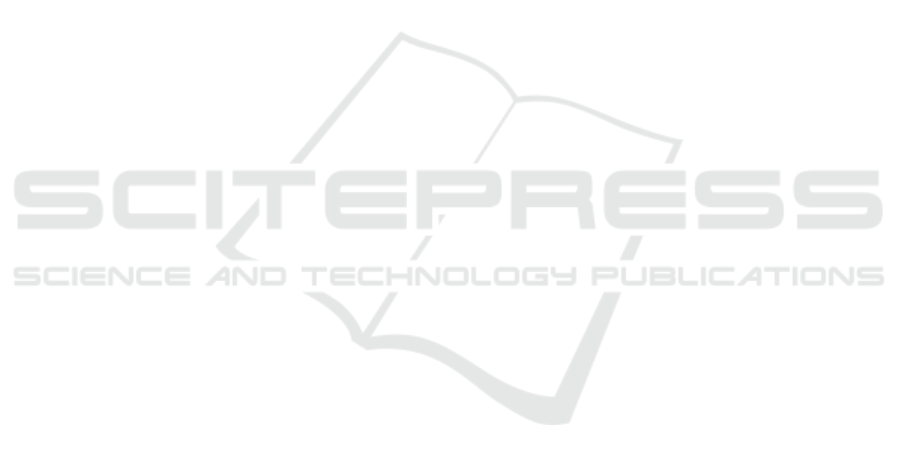
From the above calculations we got the values t
critical
and p-value from charts in (Piegorsch and Bailer,
2005) and found that t
obtained
>t
critical
and p-value < α in
call measures, which confirms our hypothesis that the
feature pivot approach performs better in a significant
way than the document pivot approach in the
performed experiments.
5 CONCLUSION AND FUTURE
WORK
From the above experiments we could deduce that
applying the feature pivot approach achieved
significantly better results than applying the
document pivot approach. That was proved by
applying both approaches on different data set sizes
(200, 400, 600, and 1200) from different domains
(sports, entertainment, news, and telecom). The Two-
sample paired one-tailed significance test was applied
to the values of the recall, precision and F1 measure
resulted from applying both approaches on the data
sets. The test showed that we could prove our
hypothesis that applying the feature pivot approach
achieves significantly better results.
This can lead us to the conclusion that applying
the feature pivot approach achieves our objective of
extracting trending topics from Egyptian dialect
tweets.
It is worth noting that each domain contains
special wording that is different in meaning from a
domain to another. Pre-processing through removing
irrelevant words from each domain enhanced the
results a lot. In the above experiments we used the
same set of stop words across all datasets, but we
noticed that if we customized a list for each domain
results would improve.
In our future work we are considering
investigating the performance of this approach on
different types of data such as customer care calls. We
are also considering representing the data using word
embedding and topic embedding techniques.
ACKNOWLEDGMENT
This research has been done with the support of the
fund granted by ITIDA ( Information Technology
Industry Development Agency) in Egypt, with the
collaboration of RDI ( The Engineering Co. For
Digital Systems Development) in Egypt and The
American University in Cairo.
REFERENCES
Aiello, L.M., Petkos, G., Martin, C., Corney, D.,
Papadopoulos, S., Skraba, R., Goker, A., Kompatsiaris,
I., Jaimes, A., 2013. Sensing Trending Topics in
Twitter. IEEE Transactions on Multimedia 15, 1268–
1282. https://doi.org/10.1109/TMM.2013.2265080
Alkhamees, N., Fasli, M., 2016. Event detection from social
network streams using frequent pattern mining with
dynamic support values, in: 2016 IEEE International
Conference on Big Data (Big Data). Presented at the
2016 IEEE International Conference on Big Data (Big
Data), IEEE, Washington DC,USA, pp. 1670–1679.
https://doi.org/10.1109/BigData.2016.7840781
Allan, J., 2002. Introduction to Topic Detection and
Tracking, in: Allan, J. (Ed.), Topic Detection and
Tracking. Springer US, Boston, MA, pp. 1–16.
https://doi.org/10.1007/978-1-4615-0933-2_1
Cataldi, M., Di Caro, L., Schifanella, C., 2010. Emerging
topic detection on Twitter based on temporal and social
terms evaluation, in: Proceedings of the Tenth
International Workshop on Multimedia Data Mining -
MDMKDD ’10. Presented at the the Tenth
International Workshop, ACM Press, Washington,
D.C., pp. 1–10.
https://doi.org/10.1145/1814245.1814249
Dai, X.-Y., Chen, Q.-C., Wang, X.-L., Xu, J., 2010. Online
topic detection and tracking of financial news based on
hierarchical clustering, in: 2010 International
Conference on Machine Learning and Cybernetics.
Presented at the 2010 International Conference on
Machine Learning and Cybernetics (ICMLC), IEEE,
Qingdao, China, pp. 3341–3346.
https://doi.org/10.1109/ICMLC.2010.5580677
Dror, R., Baumer, G., Bogomolov, M., Reichart, R., 2017.
Replicability Analysis for Natural Language
Processing: Testing Significance with Multiple
Datasets. arXiv:1709.09500 [cs].
Ha, Renee R., and James C. Ha, 2011. Integrative Statistics
for the Social and Behavioral Sciences. Sage.
Hammad, M., El-Beltagy, S.R., 2017. Towards Efficient
Online Topic Detection through Automated Bursty
Feature Detection from Arabic Twitter Streams.
Procedia Computer Science 117, 248–255.
https://doi.org/10.1016/j.procs.2017.10.116
Hasan, M., Orgun, M.A., Schwitter, R., 2018. Real-time
event detection from the Twitter data stream using the
TwitterNews+ Framework. Information Processing &
Management.
https://doi.org/10.1016/j.ipm.2018.03.001
Mathioudakis, M., Koudas, N., 2010. TwitterMonitor: trend
detection over the twitter stream, in: Proceedings of the
2010 International Conference on Management of Data
- SIGMOD ’10. Presented at the the 2010 international
conference, ACM Press, Indianapolis, Indiana, USA, p.
1155. https://doi.org/10.1145/1807167.1807306
Niwattanakul, S., Singthongchai, J., Naenudorn, E.,
Wanapu, S., 2013. Using of Jaccard Coefficient for
Keywords Similarity. Hong Kong 6.
Unsupervised Topic Extraction from Twitter: A Feature-pivot Approach
191