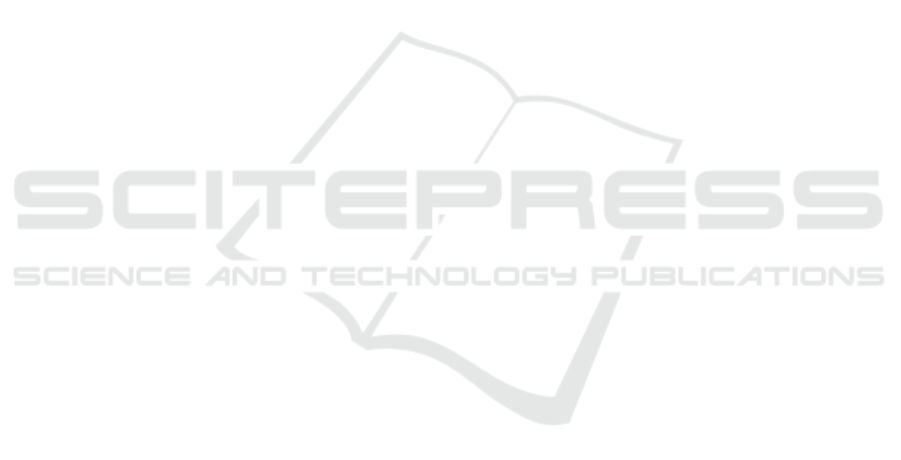
have complete knowledge. The user consents for its
data to the primary observer and by adjusting the pri-
vacy settings derives a false sense of privacy control.
Whereas, the secondary observers that participate in
user transactions either by users’ choices or by ser-
vice providers’ service composition choices are ig-
nored for their potential to collect, infer, and monetize
user data. Despite the users being provided with legal
rights to protect themselves from online tracking, pro-
filing by services, the users do not have a comprehen-
sive view of their personal infons scattered across the
data ecosystem and thus fail to exercise their rights.
We discussed the works that need to be done by the
four pillars of digital ecosystem in order to bring back
the user trust in online services for a greater good of
the ecosystem. We emphasize that the notion of an
observer is a helpful notion for users, system design-
ers in order to make informed decisions about privacy
settings, online behaviour and privacy-preserving sys-
tem designs. This will enable users to understand
and remediate their perceived privacy violations by
the environment in which they operate. Regulators
may seek an explainable proof of platform’s decision
making in building an audience for targeted adver-
tisement. This will inhibit data processors from re-
lying on data sources to which the targeted user had
not provided consent. The legal rights like right-to-
be-forgotten or right-to-consent cannot be effectively
exercised if the users do not have ability to identify
and locate their inversely private infons.
ACKNOWLEDGEMENTS
The work was carried out as part of research at IS-
RDC, supported by 15DEITY004, Ministry of Elec-
tronics and Information Technology, Govt of India.
REFERENCES
Athey, S., Catalini, C., and E. Tucker, C. (2017). The digital
privacy paradox: Small money, small costs, small talk.
SSRN Electronic Journal.
Barth, A., Datta, A., Mitchell, J. C., and Nissenbaum, H.
(2006). Privacy and contextual integrity: Framework
and applications. In IEEE S&P’06, pages 184–198.
Beaudin, L., Downey, S., Hartsoe, A., Renaud, C., and
Voorhees, J. (2019). Breaking the marketing mold
with machine learning. In MIT Tech Review Insights.
Chaabane, A., Kaafar, M. A., and Boreli, R. (2012). Big
friend is watching you: Analyzing online social net-
works tracking capabilities. In Proc. of ACM Work-
shop on Online Social Networks, pages 7–12. ACM.
Esteve, A. (2017). The business of personal data: Google,
Facebook, and privacy issues in the EU and the USA.
International Data Privacy Law, 7(1):36–47.
European Union (2018). 2018 reform of EU data protection
rules. online.
FTC (2012). Protecting Consumer Privacy in an Era of
Rapid Change: Recommendations for Businesses and
Policymakers. online.
Gurevich, Y., Hudis, E., and Wing, J. M. (2016). Inverse
Privacy. Communications of ACM, 59(7):38–42.
Kosinski, M., Stillwell, D., and Graepel, T. (2013). Pri-
vate traits and attributes are predictable from digital
records of human behavior. Proceedings of the Na-
tional Academy of Sciences, 110(15):5802–5805.
Kristensen, J., lbrechtsen, T., Dahl-Nielsen, E., Jensen, M.,
Skovrind, M., and Bornakke, T. (2017). Parsimonious
data: How a single facebook like predicts voting be-
havior in multiparty systems. PLOS ONE, 12(9):1–12.
Leon, P. G., Ur, B., Wang, Y., Sleeper, M., Balebako, R.,
Shay, R., Bauer, L., Christodorescu, M., and Cranor,
L. F. (2013). What Matters to Users?: Factors That
Affect Users’ Willingness to Share Information with
Online Advertisers. In SOUPS, pages 7:1–7:12. ACM.
Lessig, L. (1999). Code and Other Laws of Cyberspace.
Basic Books, Inc., New York, NY, USA.
Matz, S. C., Kosinski, M., Nave, G., and Stillwell, D. J.
(2017). Psychological targeting as an effective ap-
proach to digital mass persuasion. Proc. of the Na-
tional Academy of Sciences, 114(48):12714–12719.
McCallister, E., Grance, T., and Scarfone, K. A. (2010).
SP 800-122. Guide to Protecting the Confidentiality
of Personally Identifiable Information (PII). Technical
report, National Institute of Standards & Technology.
Nilizadeh, S., Kapadia, A., and Ahn, Y.-Y. (2014).
Community-enhanced de-anonymization of online so-
cial networks. In Proceedings of the 2014 ACM CCS,
pages 537–548.
Ohm, P. (2009). Broken promises of privacy: Responding
to the surprising failure of anonymization. UCLA Law
Review, Vol. 57, p. 1701, 2010.
Patil, V. T. and Shyamasundar, R. K. (2017). Privacy as a
Currency: Un-regulated? In Proceedings of the 14th
SECRYPT, pages 586–595. SciTePress.
Patil, V. T. and Shyamasundar, R. K. (2018). Efficacy of
GDPR’s Right-to-be-Forgotten on Facebook. In Infor-
mation Systems Security, volume 11281, pages 364–
385. LNCS, Springer International Publishing.
Wachter, S. and Mittelstadt, B. (2019). A Right to Reason-
able Inferences: Re-Thinking Data Protection Law in
the Age of Big Data and AI. In Columbia Business
Law Review, 2019(1). SSRN.
Xu, Y., Frahm, J.-M., and Monrose, F. (2014). Watching the
Watchers: Automatically Inferring TV Content From
Outdoor Light Effusions. In Proceedings of the 2014
ACM CCS, pages 418–428. ACM.
Youyou, W., Kosinski, M., and Stillwell, D. (2015).
Computer-based personality judgments are more ac-
curate than those made by humans. Proceedings of the
National Academy of Sciences, 112(4):1036–1040.
SECRYPT 2019 - 16th International Conference on Security and Cryptography
516