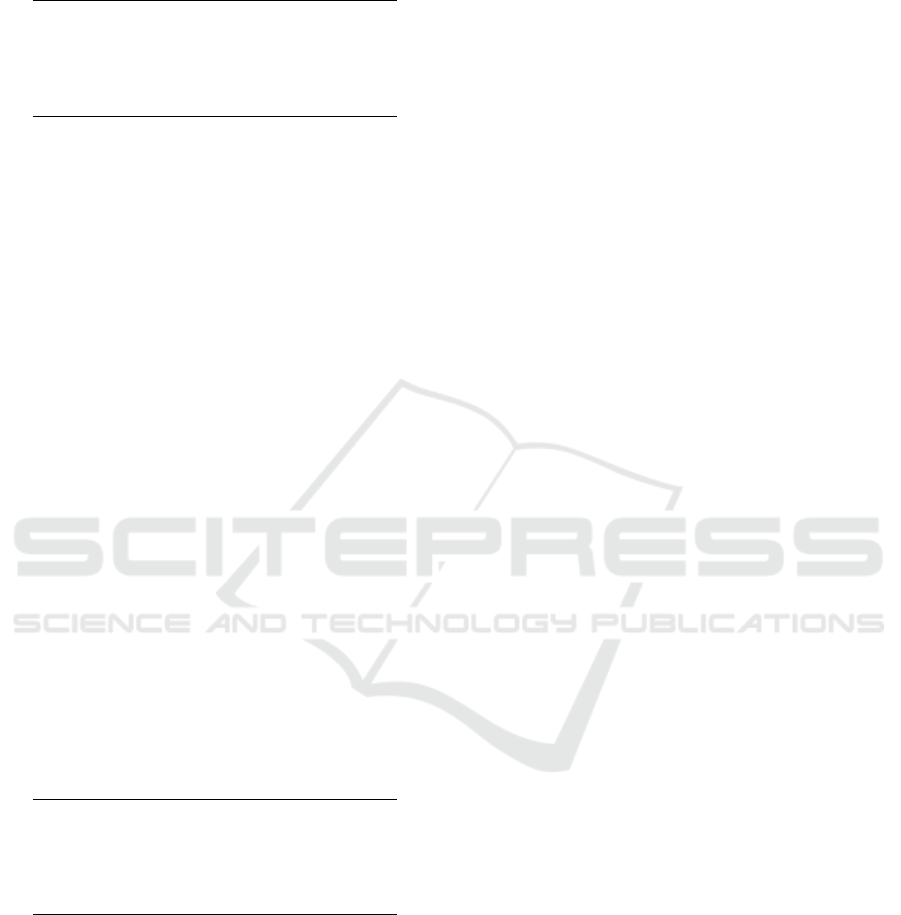
Table 3: Confusion matrix using the BN integrating a dis-
tance rejection criterion proposed by (Verron et al., 2010).
C
k
F4 F9 F11 UFC Total
F4 654 0 144 2 800
F9 0 580 216 4 800
F11 28 65 695 12 800
UFC 132 122 194 4352 4800
Total 814 767 1249 4370 7200
proposed in (Verron et al., 2010) with a misclassifica-
tion error rate equals 19.62%, see Table 3.
Also, we can see that 4454 from 4800 observa-
tions belonging to the class UFC have been recog-
nized by our approach as unknown faults (miscclas-
sification error rate = 7.20%). Further, we have ob-
tained better performance, in respect to UFC, com-
pared with the the BN proposed in (Verron et al.,
2010), 9.33%.
We have shown how our new approach outper-
forms and can be an alternative to the state of the art.
Our approach is also able to simultaneously detect
and diagnosis simultaneously known and unknown
faults in a single Bayesian network.
Once again, the reader should notice that the re-
sults obtained by our approach depend considerably
on the used BN classifier. Obviously, several BNCs
can be associated to our proposal. The structure and
parameters of a given BNC are generally learned from
data. Different BNCs could be obtained in respect
of variables relationships and considered assumptions
(Friedman et al., 1997). BNCs are not only consid-
ered as powerful tools for classification but also as
frameworks for different data-driven fault diagnosis
schemes (Atoui et al., 2015a).
Table 4: Confusion matrix of an example of a BN classifier
integrating our distance rejection criterion.
C
k
F4 F9 F11 UFC Total
F4 655 0 141 4 800
F9 0 582 217 1 800
F11 28 65 702 5 800
UFC 0 132 214 4454 4800
Total 683 779 1274 4464 7200
5 CONCLUSIONS
In this paper, a new approach able to diagnosis faults
with a distance rejection criterion is proposed. Its per-
formances on the TEP gives excellent results com-
paratively to the literature. Obvious outlook of this
work is to expand our approach to simultaneously de-
tect and diagnose known and unknown faults. Also,
develop it for others data-driven BNCs to fault detec-
tion and diagnosis. Furthermore, it can be interesting
to enhance the decisions made by our approach by
considering different types of information.
REFERENCES
Atoui, M. A., Verron, S., and Abdessamad, K. (2014). Con-
ditional gaussian network as pca for fault detection.
IFAC Proceedings Volumes, 47(3):1935–1940.
Atoui, M. A., Verron, S., and Kobi, A. (2015a). Fault
detection and diagnosis in a bayesian network clas-
sifier incorporating probabilistic boundary1. IFAC-
PapersOnLine, 48(21):670–675.
Atoui, M. A., Verron, S., and Kobi, A. (2015b). Fault detec-
tion with conditional gaussian network. Engineering
Applications of Artificial Intelligence, 45:473–481.
Atoui, M. A., Verron, S., and Kobi, A. (2016). A bayesian
network dealing with measurements and residuals for
system monitoring. Transactions of the Institute of
Measurement and Control, 38(4):373–384.
Chiang, L., Russell, E., and Braatz, R. (2012). Fault De-
tection and Diagnosis in Industrial Systems. Springer
Science & Business Media.
Downs, J. J. and Vogel, E. F. (1993). A plant-wide indus-
trial process control problem. Computers & chemical
engineering, 17(3):245–255.
Friedman, N., Geiger, D., and Goldszmidt, M. (1997).
Bayesian network classifiers. Machine learning, 29(2-
3):131–163.
He, S., Wang, Z., Wang, Z., Gu, X., and Yan, Z. (2016).
Fault detection and diagnosis of chiller using bayesian
network classifier with probabilistic boundary. Ap-
plied Thermal Engineering, 107:37–47.
Jin, S., Liu, C., Lai, X., Li, F., and He, B. (2017). Bayesian
network approach for ceramic shell deformation fault
diagnosis in the investment casting process. The In-
ternational Journal of Advanced Manufacturing Tech-
nology, 88(1-4):663–674.
Kawahara, Y., Yairi, T., and Machida, K. (2005). Diag-
nosis method for spacecraft using dynamic bayesian
networks. In Proc. of 8th International symposium
on Artificial Intelligence, Robotics and Automation in
Space (i-SAIRAS). Citeseer.
Nielsen, T. D. and Jensen, F. V. (2009). Bayesian networks
and decision graphs. Springer Science & Business
Media.
Roychoudhury, I., Biswas, G., and Koutsoukos, X. (2006).
A bayesian approach to efficient diagnosis of incipi-
ent faults. In Proceedings of the 17th International
Workshop on Principles of Diagnosis (DX-06), pages
243–250. Citeseer.
Schwall, M. L. and Gerdes, J. C. (2002). A probabilistic
approach to residual processing for vehicle fault de-
tection. In Proceedings of the 2002 American Con-
trol Conference (IEEE Cat. No. CH37301), volume 3,
pages 2552–2557. IEEE.
Verron, S., Tiplica, T., and Kobi, A. (2010). Fault diagnosis
of industrial systems by conditional gaussian network
Fault Diagnosis by Bayesian Network Classifiers with a Distance Rejection Criterion
467