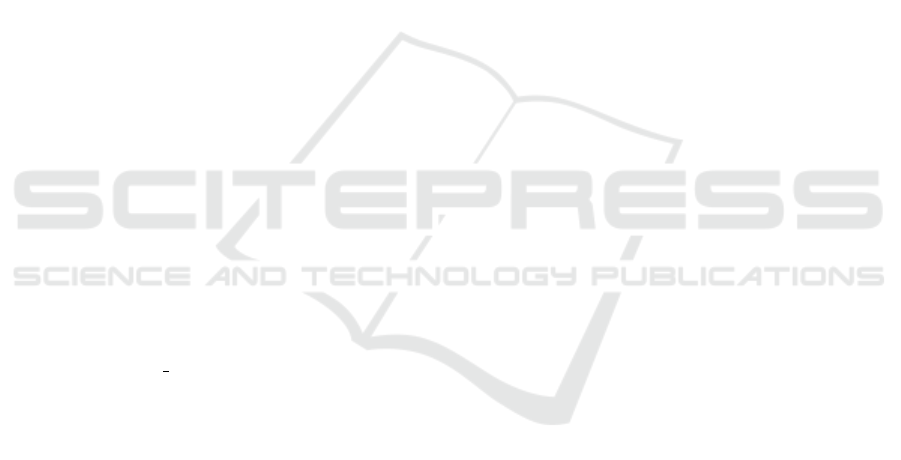
5 CONCLUSION AND FUTURE
WORK
In this paper, we presented an approach to predicting
sentiment polarity in Twitter. The prediction depends
on the past content of users according to identified
topics. The utilized collection is that of the senti-
ment classification task of the SemEval 2016 edition.
Added to that, we collected past tweets of each author
in the collection. Our approach depends on super-
vised learning. The used features are sentiment mea-
sures at the tweets level, semantic measures between
tweets and topics, and time scores. As a first experi-
ment, the results obtained are acceptable, and for this
reason we will try to improve the performance in fu-
ture work by adopting deep learning as well as testing
the approach on a larger collection.
REFERENCES
Asur, S. and Huberman, B. A. (2010). Predicting the fu-
ture with social media. In Proceedings of the 2010
IEEE/WIC/ACM International Conference on Web In-
telligence and Intelligent Agent Technology-Volume
01, pages 492–499. IEEE Computer Society.
Barbosa, L. and Feng, J. (2010). Robust sentiment detection
on twitter from biased and noisy data. In Proceed-
ings of the 23rd international conference on computa-
tional linguistics: posters, pages 36–44. Association
for Computational Linguistics.
Cambria, E., Das, D., Bandyopadhyay, S., and Feraco, A.
(2017). A practical guide to sentiment analysis, vol-
ume 5. Springer.
Cliche, M. (2017). Bb twtr at semeval-2017 task 4: Twitter
sentiment analysis with cnns and lstms. arXiv preprint
arXiv:1704.06125.
De Sousa Webber, F. (2015). Semantic folding theory
and its application in semantic fingerprinting. arXiv
preprint arXiv:1511.08855, page 38.
Gilbert, C. H. E. (2014). Vader: A parsimonious
rule-based model for sentiment analysis of so-
cial media text. In Eighth International Confer-
ence on Weblogs and Social Media (ICWSM-14).
Available at (20/04/16) http://comp. social. gatech.
edu/papers/icwsm14. vader. hutto. pdf.
Mikolov, T., Sutskever, I., Chen, K., Corrado, G. S., and
Dean, J. (2013). Distributed representations of words
and phrases and their compositionality. In Advances in
neural information processing systems, pages 3111–
3119.
Mnasri, M., De Chalendar, G., and Ferret, O. (2015).
Int
´
egration de la similarit
´
e entre phrases comme
crit
`
ere pour le r
´
esum
´
e multi-document. In 23
`
eme
Conf
´
erence sur le Traitement Automatique des
Langues Naturelles (JEP-TALN-RECITAL 2016), ses-
sion articles courts, pages 482–489.
Nakov, P., Ritter, A., Rosenthal, S., Sebastiani, F., and Stoy-
anov, V. (2016). Semeval-2016 task 4: Sentiment
analysis in twitter. In Proceedings of the 10th inter-
national workshop on semantic evaluation (semeval-
2016), pages 1–18.
Nguyen, L. T., Wu, P., Chan, W., Peng, W., and Zhang,
Y. (2012). Predicting collective sentiment dynamics
from time-series social media. In Proceedings of the
first international workshop on issues of sentiment dis-
covery and opinion mining, page 6. ACM.
Nielsen, F.
˚
A. (2011). A new anew: Evaluation of a
word list for sentiment analysis in microblogs. arXiv
preprint arXiv:1103.2903.
Pennebaker, J., Chung, C., Ireland, M., Gonzales, A., and
Booth, R. (2007). The development and psychometric
properties of liwc2007: Liwc. net. Google Scholar.
Stone, P. J., Dunphy, D. C., and Smith, M. S. (1966).
The general inquirer: A computer approach to content
analysis.
Tang, D., Wei, F., Yang, N., Zhou, M., Liu, T., and Qin, B.
(2014). Learning sentiment-specific word embedding
for twitter sentiment classification. In Proceedings of
the 52nd Annual Meeting of the Association for Com-
putational Linguistics (Volume 1: Long Papers), vol-
ume 1, pages 1555–1565.
Tumasjan, A., Sprenger, T. O., Sandner, P. G., and Welpe,
I. M. (2010). Predicting elections with twitter: What
140 characters reveal about political sentiment. In
Fourth international AAAI conference on weblogs and
social media.
Wilson, T. D. and Gilbert, D. T. (2003). Affective fore-
casting. Advances in experimental social psychology,
35(35):345–411.
WEBIST 2019 - 15th International Conference on Web Information Systems and Technologies
256