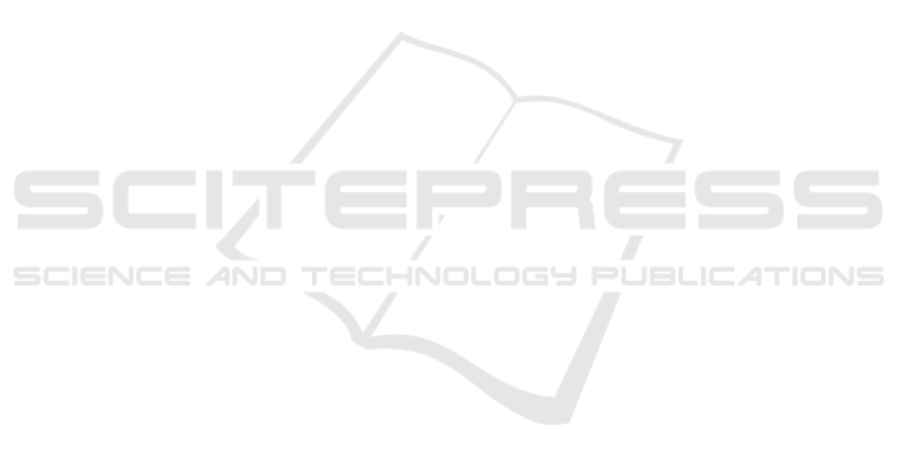
on the active periods of the machines using ARIMA
method was proposed by (Subramaniyan et al., 2018).
Similarly, a big data analytical architecture for prod-
uct life cycle management was presented by (Zhang
et al., 2017). Furthermore, (Shin et al., 2017) pre-
sented an analytic model for predicting energy con-
sumption of manufacturing machinery.
In the best of our knowledge, this paper is the first
to deal with in-depth analysis of sensor binary data
in order to enhance operational efficiency for smart
manufacturing based on the real world case study.
8 CONCLUSIONS AND FUTURE
WORK
This paper presents the fundamental concepts of data
analytics based on a real world case study. These
concepts include data understanding, data prepara-
tion, data pipeline and data analytics technologies. To
enhance the operational efficiency in-depth descrip-
tive and predictive analysis were performed. Super-
vised machine learning technique was used to create
the classification model to predicts machine stops. In
addition, Overall Equipment Effectiveness (OEE) and
the performance of the prediction method were com-
prehensively evaluated. The results have drawn atten-
tion towards improving the production performance
by reducing the machine downtime. Whereas, the
predictions made by the model are quite acceptable in
terms of predicting the unplanned stops, as unplanned
stops are one of the main reasons of reduced produc-
tion performance.
For the future work, several prediction based ma-
chine learning models will be used and compared. In
addition, a near real-time dashboard will be developed
to display the input/output pace along with the OEE
information. Finally, it will be investigated that how
descriptive analysis, predictive analysis and near real-
time dashboard help the smart manufacturing compa-
nies in general, to enhance their operational efficiency
and productivity.
REFERENCES
Auschitzky, E., Markus, H., and Agesan, R. (2014).
How big data can improve manufacturing.
Available online at: https://www.mckinsey.
com/business-functions/operations/our-insights/
how-big-data-can-improve-manufacturing.
Chapman, P., Clinton, J., Kerber, R., Khabaza, T., Reinartz,
T., Shearer, C., and Wirth, R. (1999). Crisp-dm 1.0
step-by-step data mining guide. In 4th CRISP-DM
SIG Workshop in Brussels in March (Vol. 1999). SPSS
Inc.
Gonzalez, S., Garcia, S., Li, S. T., and Herrera, F. (2019).
Chain based sampling for monotonic imbalanced clas-
sification. Information Sciences, 474:187–204.
Gooijer, J. G. D. and Hyndman, R. J. (2006). 25 years of
time series forecasting. International Journal of Fore-
casting, 22(3):443–473.
Iftikhar, N., Liu, X., and Nordbjerg, F. E. (2015).
Relational-based sensor data cleansing. In ADBIS’15,
East European Conference on Advances in Databases
and Information Systems, pages 108–118. Springer,
Cham.
Kamble, S. S., Gunasekaran, A., and Gawankar, S. A.
(2018). Sustainable industry 4.0 framework: A sys-
tematic literature review identifying the current trends
and future perspectives. Process Safety and Environ-
mental Protection, 117:408–425.
Lee, J., Kao, H. A., and Yang, S. (2014). Service innova-
tion and smart analytics for industry 4.0 and big data
environment. Procedia CIR, 16:3–8.
Moyne, J. and Iskandar, J. (2017). Big data analytics for
smart manufacturing: Case studies in semiconductor
manufacturing. Processes, 5(3):39–58.
Muller, O., Fay, M., and vom Brocke, J. (2018). The ef-
fect of big data and analytics on firm performance: An
econometric analysis considering industry character-
istics. Journal of Management Information Systems,
35(2):488–509.
Shin, S. J., Woo, J., and Rachuri, S. (2017). Predictive ana-
lytics model for power consumption in manufacturing.
Procedia CIRP, 15:153–158.
Subramaniyan, M., Skoogh, A., Salomonsson, H., Banga-
lore, P., and Bokrantz, J. (2018). A data-driven algo-
rithm to predict throughput bottlenecks in a produc-
tion system based on active periods of the machines.
Computers & Industrial Engineering, 125:533–544.
Tao, F., Qi, Q., Liu, A., and Kusiak, A. (2018). Data-driven
smart manufacturing. Journal of Manufacturing Sys-
tems, 48:157–169.
Wang, J., Ma, Y., Zhang, L., Gao, R. X., and Wu, D. (2018).
Deep learning for smart manufacturing. Journal of
Manufacturing Systems, 48:144–156.
Zhang, Y., Ren, S., Liu, Y., and Si, S. (2017). A big data
analytics architecture for cleaner manufacturing and
maintenance processes of complex products. Comput-
ers & Industrial Engineering, 142:626–641.
Data Analytics for Smart Manufacturing: A Case Study
399