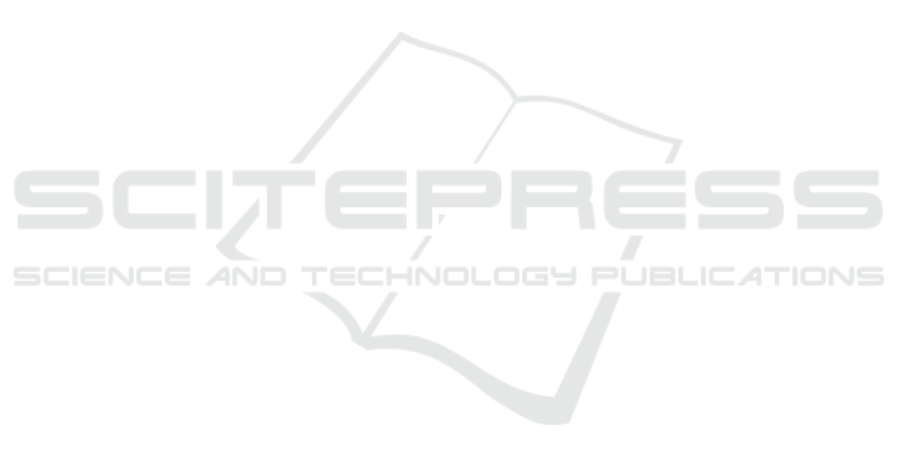
6 CONCLUSIONS AND FUTURE
WORK
We have studied how users behave when they en-
counter phishing email attacks. To the best of our
knowledge, this is the first comprehensive and quanti-
tative investigation of how users react in email check-
ing and reading that have become an integral part of
our daily life. We have designed two studies, on-
site study and online study. We have applied statis-
tical methods to analyze our on-site dataset and ex-
plore the answers to the questions on how interven-
tion, phishing types, and a monetary incentive af-
fect user behaviors when phishing attacks are encoun-
tered. Our analysis have showed that participants with
intervention and a monetary incentive perform better
than other cases. Phishing type 1, suspicious senders’
email addresses, tends to be more harmful to users
compared to other two phishing types. We have fur-
ther developed machine learning techniques with the
10-fold cross-validation to analyze the data collected
in the online study. We have analyzed the best at-
tributes used in our machine learning framework. By
choosing 16 attributes, we have achieved the user per-
formance prediction accuracies of 86.67%, 88.89%,
92.22%, and 96.67% for J48, Naive Bayes, SVM, and
Multilayer Perceptron, respectively.
In daily-life scenarios, we tend to deal with many
other things while checking our emails; thus, we plan
to investigate a multitasking experiment platform to
understand how multitasking will affect the behavior
of a user accordingly.
ACKNOWLEDGEMENT
We would like to thank NSF for partially spon-
soring the work under grants #1620868, #1620871,
#1620862, and #1651280. We also thank the JHU
team that provided the data used in this project.
REFERENCES
Brase, G. L. (2009). How different types of participant pay-
ments alter task performance. Judgment and Decision
Making, page 419.
Burns, M. B., Durcikova, A., and Jenkins, J. L. (2013).
What kind of interventions can help users from falling
for phishing attempts: a research proposal for exam-
ining stage-appropriate interventions. In HICSS.
Chin, T. J., Xiong, K., and Hu, C. (2018). Phishlimiter:
A phishing detection and mitigation approach using
software-defined networking. In IEEE Access.
Database, P. B. (Accessed Sept. 2018). [online]. Available:
https://it.cornell.edu/phish-bowl.
Goel, S., Williams, K., and Dincelli, E. (2017). Got
phished? Internet security and human vulnerability.
Journal of the Association for Information Systems,
18(1):22.
Gupta, S., Singhal, A., and Kapoor, A. (2016). A literature
survey on social engineering attacks: Phishing attack.
In ICCCA, pages 537–540.
Harrison, B., Svetieva, E., and Vishwanath, A. (2016). In-
dividual processing of phishing emails: How attention
and elaboration protect against phishing. Online Infor-
mation Review, 40(2):265–281.
Liang, H. and Xue, Y. (2010). Understanding security be-
haviors in personal computer usage: A threat avoid-
ance perspective. JAIS.
MTurk, A. M. T. W. (Accessed Sept, 2018). [Online].
Available: https:// www.mturk.com/ mturk/welcome.
Muthal, S., Li, S., Huang, Y., Li, X., Dahbura, A., Bos, N.,
and Molinaro, K. (2017). A phishing study of user
behavior with incentive and informed intervention. In
Proceedings of the National Cyber Summit.
Pande, D. N. and Voditel, P. S. (2017). Spear phishing: Di-
agnosing attack paradigm. In WiSPNET, pages 2720–
2724. IEEE.
Parsons, K., Butavicius, M., Pattinson, M., Calic, D., Mc-
cormac, A., and Jerram, C. (2016). Do users focus on
the correct cues to differentiate between phishing and
genuine emails? arXiv preprint:1605.04717.
Rakhra, M. and Kaur, D. (2018). Studying user’s com-
puter security behaviour in developing an effective an-
tiphishing educational framework. In ICISC. IEEE.
Smadi, S., Aslam, N., Zhang, L., Alasem, R., and Hossain,
M. (2015). Detection of phishing emails using data
mining algorithms. In SKIMA, pages 1–8. IEEE.
Vishwanath, A., Harrison, B., and Ng, Y. J. (2016). Sus-
picion, cognition, and automaticity model of phishing
susceptibility. Communication Research.
Vishwanath, A., Herath, T., Chen, R., Wang, J., and Rao,
H. R. (2011). Why do people get phished? testing
individual differences in phishing vulnerability within
an integrated, information processing model. Decision
Support Systems, 51(3).
Williams, N. and Li, S. (2017). Simulating human detection
of phishing websites: An investigation into the appli-
cability of the act-r cognitive behaviour architecture
model. In CYBCONF, pages 1–8. IEEE.
Wu, M., Miller, R. C., and Garfinkel, S. L. (2006). Do
security toolbars actually prevent phishing attacks?
In Proceedings of the SIGCHI conference on Human
Factors in computing systems. ACM.
Yang, W., Chen, J., Xiong, A., Proctor, R. W., and Li, N.
(2015). Effectiveness of a phishing warning in field
settings. In Proceedings of the Symposium and Boot-
camp on the Science of Security, page 14. ACM.
SECRYPT 2019 - 16th International Conference on Security and Cryptography
534