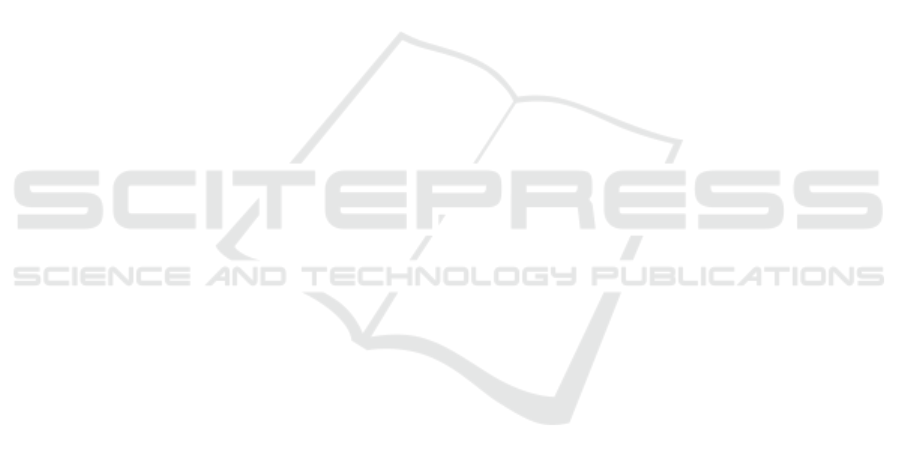
Birmingham, B., Muscat, A., and Belz, A. (2018). Adding
the third dimension to spatial relation detection in 2d
images. In Proceedings of the 11th International Con-
ference on Natural Language Generation, pages 146–
151.
Carlson-Radvansky, L. A. and Logan, G. D. (1997). The
influence of reference frame selection on spatial tem-
plate construction. JOURNAL OF MEMORY AND
LANGUAGE, 37:411–437.
Carlson-Radvansky, L. A. and Radvansky, G. A. (1996).
The influence of functional relations on spatial term
selection. Psychological Science, 7(1):56–60.
Coventry, K. R., Prat-Sala, M., and Richards, L. (2001). The
interplay between geometry and function in the com-
prehension of over, under, above, and below. Journal
of Memory and Language, 44(3):376 – 398.
Dai, B., Zhang, Y., and Lin, D. (2017). Detecting visual
relationships with deep relational networks. In Com-
puter Vision and Pattern Recognition (CVPR), 2017
IEEE Conference on, pages 3298–3308. IEEE.
Dobnik, S. and Kelleher, J. (2014). Exploration of func-
tional semantics of prepositions from corpora of de-
scriptions of visual scenes. In Proceedings of the
Third Workshop on Vision and Language, pages 33–
37. Dublin City University and the Association for
Computational Linguistics.
Jain, A. K., Murty, M. N., and Flynn, P. J. (1999). Data
clustering: a review. ACM computing surveys (CSUR),
31(3):264–323.
Kelleher, J. D. and Kruijff, G.-J. (2005). A context-
dependent algorithm for generating locative expres-
sions in physically situated environments. In Proceed-
ings of the Tenth European Workshop on Natural Lan-
guage Generation (ENLG-05).
Kelleher, J. D., Ross, R. J., Sloan, C., and Namee, B. M.
(2011). The effect of occlusion on the semantics of
projective spatial terms: a case study in grounding lan-
guage in perception. Cognitive Processing, 12(1):95–
108.
Logan, G. D. and Sadler, D. D. (1996). A computa-
tional analysis of the apprehension of spatial rela-
tions, pages 493–529. The MIT Press, Cambridge,
MA, US.
Lu, C., Krishna, R., Bernstein, M., and Fei-Fei, L. (2016).
Visual relationship detection with language priors.
In European Conference on Computer Vision, pages
852–869. Springer.
Mikolov, T., Sutskever, I., Chen, K., Corrado, G. S., and
Dean, J. (2013). Distributed representations of words
and phrases and their compositionality. In Advances in
neural information processing systems, pages 3111–
3119.
Muscat, A. and Belz, A. (2017). Learning to generate
descriptions of visual data anchored in spatial rela-
tions. IEEE Computational Intelligence Magazine,
12(3):29–42.
Pedregosa, F., Varoquaux, G., Gramfort, A., Michel, V.,
Thirion, B., Grisel, O., Blondel, M., Prettenhofer,
P., Weiss, R., Dubourg, V., Vanderplas, J., Passos,
A., Cournapeau, D., Brucher, M., Perrot, M., and
Duchesnay,
´
E. (2011). Scikit-learn: Machine learning
in Python. Journal of Machine Learning Research,
12:2825–2830.
Pennington, J., Socher, R., and Manning, C. (2014). Glove:
Global vectors for word representation. In Proceed-
ings of the 2014 conference on empirical methods in
natural language processing (EMNLP), pages 1532–
1543.
Ramisa, A., Wang, J., Lu, Y., Dellandrea, E., Moreno-
Noguer, F., and Gaizauskas, R. (2015). Combining
geometric, textual and visual features for predicting
prepositions in image descriptions. In Proc. 20th
Conf. on Empirical Methods in Natural Language
Processing (EMNLP), pages 214–220, Lisbon, Portu-
gal.
Regier, T. and Carlson, L. A. (2001). Grounding spatial
language in perception: an empirical and computa-
tional investigation. Journal of Experimental Psychol-
ogy General, 130(2):273–298.
Sadeghi, M. A. and Farhadi, A. (2011). Recognition us-
ing visual phrases. In Computer Vision and Pat-
tern Recognition (CVPR), 2011 IEEE Conference on,
pages 1745–1752. IEEE.
Tsoumakas, G. and Katakis, I. (2007). Multi-label classi-
fication: An overview. Int J Data Warehousing and
Mining, 2007:1–13.
Yu, R., Li, A., Morariu, V. I., and Davis, L. S. (2017). Visual
relationship detection with internal and external lin-
guistic knowledge distillation. In IEEE International
Conference on Computer Vision (ICCV).
Zhang, M. and Zhou, Z. (2014). A review on multi-label
learning algorithms. IEEE Transactions on Knowl-
edge and Data Engineering, 26(8):1819–1837.
ICINCO 2019 - 16th International Conference on Informatics in Control, Automation and Robotics
156