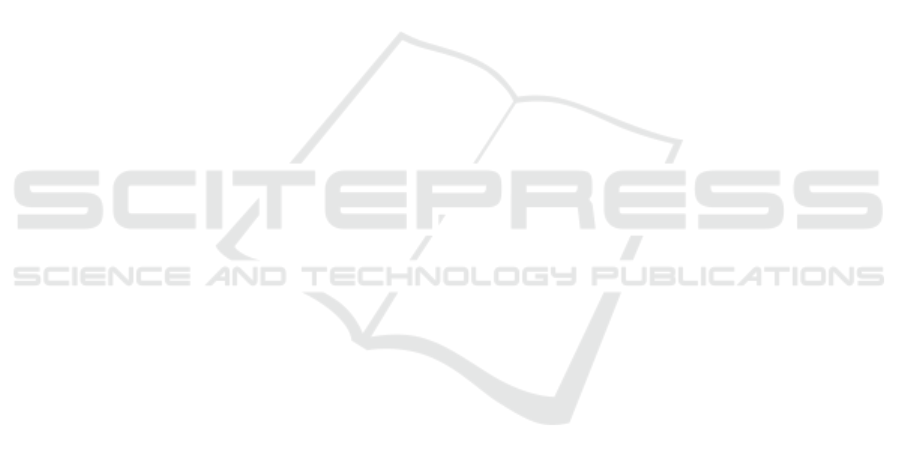
volved. Based on the usage of RDF and the 3-RMM
compliance, the agent system AJAN is able to au-
tonomously understand new software components in
the architecture. Adapting the architecture to new
domain-specific applications is then reduced to mod-
elling fitting SPARQL-BTs that operate on the new
data. Our approach has been applied to an aerospace
industry use case in an air plane assembly line.
ACKNOWLEDGEMENTS
The work described in this paper has been partially
funded by the German Federal Ministry of Education
and Research (BMBF) through the projects Hybr-iT
under the grant 01IS16026A, and REACT under the
grant 01/W17003.
REFERENCES
Antakli, A., Hermann, E., Zinnikus, I., Du, H., and Fischer,
K. (2018). Intelligent distributed human motion simu-
lation in human-robot collaboration environments. In
Proceedings of the 18th International Conference on
Intelligent Virtual Agents, IVA ’18, pages 319–324,
New York, NY, USA. ACM.
Awad, R., Fechter, M., and van Heerden, J. (2017). Inte-
grated risk assessment and safety consideration dur-
ing design of HRC workplaces. In 2017 22nd IEEE
International Conference on Emerging Technologies
and Factory Automation (ETFA), pages 1–10.
Bosse, S. (2016). Mobile Multi-agent Systems for the
Internet-of-Things and Clouds Using the JavaScript
Agent Machine Platform and Machine Learning as a
Service. In 2016 IEEE 4th International Conference
on Future Internet of Things and Cloud (FiCloud),
pages 244–253.
Diaconescu, I. M. and Wagner, G. (2015). Modeling and
Simulation of Web-of-Things Systems as Multi-Agent
Systems. In Mueller, J. P., Ketter, W., Kaminka,
G., Wagner, G., and Bulling, N., editors, Multiagent
System Technologies, volume 9433, pages 137–153.
Springer International Publishing, Cham.
Fielding, R. T. and Taylor, R. N. (2002). Principled design
of the modern web architecture. ACM Transactions on
Internet Technology (TOIT), 2(2):115–150.
Fritzsche, L., Sch
¨
onherr, R., and Illmann, B. (2014). Inter-
active simulation and ergonomics assessment of man-
ual work with EMA–applications in product develop-
ment and production planning. In Advances in Applied
Digital Human Modeling. AHFE, pages 49–58.
Garcia-Sanchez, F., Fern
´
andez-Breis, J. T., Valencia-
Garcia, R., G
´
omez, J. M., and Martinez-B
´
ejar, R.
(2008). Combining Semantic Web technologies with
Multi-Agent Systems for integrated access to biolog-
ical resources. Journal of Biomedical Informatics,
41(5):848–859.
Gelfond, M. and Lifschitz, V. (1998). Action languages.
Electronic Transactions on AI, 3.
Gopinath, V. and Johansen, K. (2016). Risk assessment pro-
cess for collaborative assembly–a job safety analysis
approach. Procedia CIRP, 44:199–203.
Guinard, D. and Trifa, V. (2009). Towards the web of things:
Web mashups for embedded devices. In Workshop on
Mashups, Enterprise Mashups and Lightweight Com-
position on the Web (MEM 2009), in proceedings of
WWW (International World Wide Web Conferences),
Madrid, Spain, volume 15.
Heath, T. and Bizer, C. (2011). Linked data: Evolving the
web into a global data space. Synthesis lectures on the
semantic web: theory and technology, 1(1):1–136.
Herrmann, E., Manns, M., Du, H., Hosseini, S., and Fischer,
K. (2017). Accelerating statistical human motion syn-
thesis using space partitioning data structures. Com-
puter Animation and Virtual Worlds, 28(3-4):e1780.
Holden, D., Komura, T., and Saito, J. (2017). Phase-
functioned neural networks for character control.
ACM Transactions on Graphics (TOG), 36(4):42.
Kashevnik, A., Teslya, N., Yablochnikov, E., Arckhipov, V.,
and Kipriianov, K. (2016). Development of a proto-
type cyber physical production system with help of
smart-m3. In IECON 2016 - 42nd Annual Confer-
ence of the IEEE Industrial Electronics Society, pages
4890–4895.
Khriyenko, O. and Nagy, M. (2011). Semantic web-driven
agent-based ecosystem for linked data and services.
In Proceedings of the Third International Conferences
on Advanced Service Computing, pages 25–30.
Koenig, N. and Howard, A. (2004). Design and use
paradigms for gazebo, an open-source multi-robot
simulator. In 2004 IEEE/RSJ International Confer-
ence on Intelligent Robots and Systems (IROS) (IEEE
Cat. No.04CH37566), volume 3, pages 2149–2154.
Marzinotto, A., Colledanchise, M., Smith, C., and
¨
Ogren, P.
(2014). Towards a unified behavior trees framework
for robot control. In 2014 IEEE International Con-
ference on Robotics and Automation (ICRA), pages
5420–5427.
Meneguzzi, F. R., Zorzo, A. F., and da Costa M
´
ora, M.
(2004). Propositional planning in BDI agents. In
Proceedings of the 2004 ACM Symposium on Applied
Computing, SAC ’04, pages 58–63, New York, NY,
USA. ACM.
Min, J. and Chai, J. (2012). Motion graphs++: a com-
pact generative model for semantic motion analysis
and synthesis. ACM Transactions on Graphics (TOG),
31(6):153.
Mourtzis, D., Papakostas, N., Mavrikios, D., Makris, S.,
and Alexopoulos, K. (2015). The role of simulation in
digital manufacturing: applications and outlook. In-
ternational Journal of Computer Integrated Manufac-
turing, 28(1):3–24.
Nguyen, H., Ciocarlie, M. T., Hsiao, K., and Kemp, C. C.
(2013). ROS commander (ROSCo): Behavior cre-
ation for home robots. 2013 IEEE International Con-
ference on Robotics and Automation, pages 467–474.
WEBIST 2019 - 15th International Conference on Web Information Systems and Technologies
98