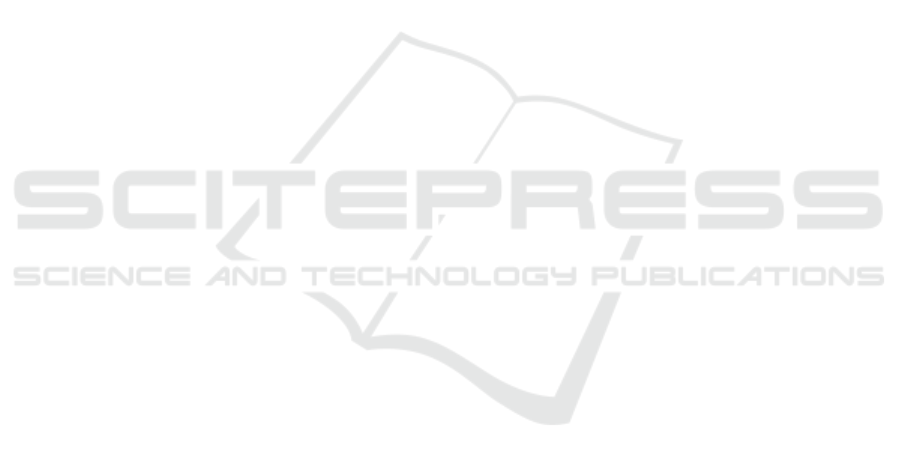
Fessahaye, F., P
´
erez, L., Zhan, T., Zhang, R., Fossier, C.,
Markarian, R., Chiu, C., Zhan, J., Gewali, L., and Oh,
P. (2019). T-recsys: A novel music recommendation
system using deep learning. In 2019 IEEE Interna-
tional Conference on Consumer Electronics (ICCE),
pages 1–6.
Germain, A. and Chakareski, J. (2013). Spotify me:
Facebook-assisted automatic playlist generation. In
2013 IEEE 15th International Workshop on Multime-
dia Signal Processing (MMSP), pages 25–28.
Han, B.-j., Rho, S., Jun, S., and Hwang, E. (2010). Music
emotion classification and context-based music rec-
ommendation. Multimedia Tools and Applications,
47(3):433–460.
Kaminskas, M. and Ricci, F. (2011). Location-adapted
music recommendation using tags. In Proceedings
of the 19th International Conference on User Model-
ing, Adaption, and Personalization, UMAP’11, pages
183–194, Berlin, Heidelberg. Springer-Verlag.
Knees, P., Schedl, M., Ferwerda, B., and Laplante, A.
(2019). User awareness in music recommender sys-
tems. In Personalized Human-Computer Interaction.
Lee, S. K., Cho, Y. H., and Kim, S. H. (2010). Collabora-
tive filtering with ordinal scale-based implicit ratings
for mobile music recommendations. Information Sci-
ences, 180(11):2142–2155.
Levitin, D., D James Mcgill, P., Daniel, D., and Levitin, J.
(2007). Life soundtracks: The uses of music in every-
day life’.
Madathil, M. (2017). Music recommendation system spo-
tify - collaborative filtering. Reports in Computer Mu-
sic. Aachen University, Germany.
Mahmud, R., Kotagiri, R., and Buyya, R. (2018). Fog Com-
puting: A Taxonomy, Survey and Future Directions,
pages 103–130. Springer.
North, A. C., Hargreaves, D. J., and Hargreaves, J. J. (2004).
Uses of music in everyday life. Music Perception: An
Interdisciplinary Journal, 22(1):41–77.
Oliver, N. and Kreger-Stickles, L. (2006). Papa: Physiology
and purpose-aware automatic playlist generation. In
ISMIR, volume 2006, page 7th.
Pampalk, E., Pohle, T., and Widmer, G. (2005). Dynamic
playlist generation based on skipping behavior. In IS-
MIR, volume 5, pages 634–637.
Pichl, M., Zangerle, E., and Specht, G. (2015). Combin-
ing spotify and twitter data for generating a recent and
public dataset for music recommendation. In Proceed-
ings of the 26th GI-Workshop Grundlagen von Daten-
banken (GvDB 2014), Ritten, Italy.
Pivotal Software (2017). Spring framework. https://spring.
io/projects/spring-framework.
Russell, J. (1980). A circumplex model of affect. Journal of
personality and social psychology, 39(6):1161–1178.
Su, J., Yeh, H., Yu, P. S., and Tseng, V. S. (2010). Music
recommendation using content and context informa-
tion mining. IEEE Intelligent Systems, 25(1):16–26.
Terry, P. (2006). Psychophysical effects of music in sport
and exercise: an update on theory, research and appli-
cation. pages 415–419.
Vall, A., Dorfer, M., Eghbal-zadeh, H., Schedl, M., Burjor-
jee, K., and Widmer, G. (2019a). Feature-combination
hybrid recommender systems for automated music
playlist continuation. User Modeling and User-
Adapted Interaction, 29:527–572.
Vall, A., Quadrana, M., Schedl, M., and Widmer, G.
(2019b). Order, context and popularity bias in next-
song recommendations. International Journal of Mul-
timedia Information Retrieval, 8(2):101–113.
Wang, X., Rosenblum, D., and Wang, Y. (2012). Context-
aware mobile music recommendation for daily activ-
ities. In Proceedings of the 20th ACM international
conference on Multimedia, pages 99–108. ACM.
Yang, X., Dong, Y., and Li, J. (2018). Review of data
features-based music emotion recognition methods.
Multimedia Syst., 24(4):365–389.
Zheng, H.-T., Chen, J.-Y., Liang, N., Sangaiah, A. K., Jiang,
Y., and Zhao, C.-Z. (2019). A deep temporal neu-
ral music recommendation model utilizing music and
user metadata. Applied Sciences, 9(4).
DJ-Running: An Emotion-based System for Recommending Spotify Songs to Runners
63