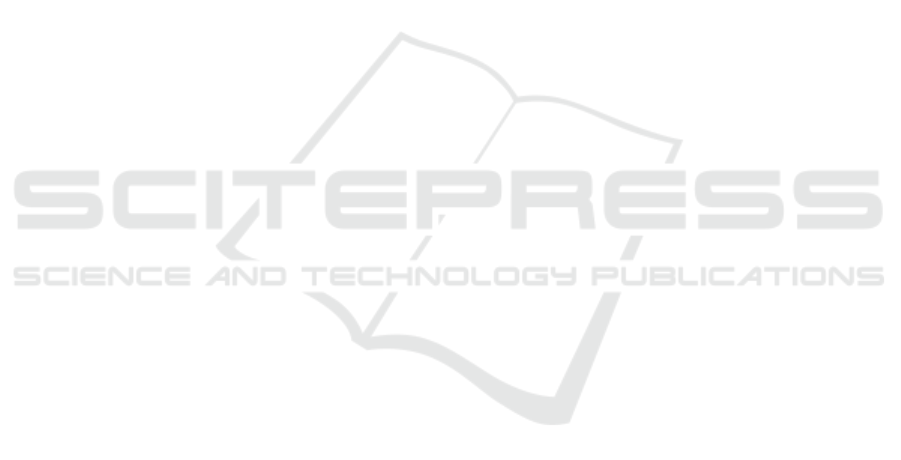
separate simulations, dividing the course into two sec-
tions with clear difference in wind conditions. The
model does not consider braking and cornering, and
this can lead to an underestimation of simulated time
for courses with fast downhill sections and sharp turns.
The other set of limitations is the data input to the
model. The wind tunnel data were measured for the
head down position that a rider normally uses during
straight sections of the course, where much attention
to the road ahead is not needed. In the validation tests
as well as in a race, this will not always be possible, as
curves and uneven road surfaces forces the rider to raise
their head to scan the road ahead. This will cause the
simulated time to be lower than the real time at certain
points. However, the time spent in the aero-position
increases with the level of the cyclist and is large com-
pared to the time spent in deviating positions for most
races. It is shown by (Olds et al., 1995) that riders of
a higher level also have a higher discipline of pacing
and positioning while riding. As none of the test rid-
ers hold international level as cyclists, this effect of
this will be greater in the performed tests than for a
World Tour rider. Wind tunnel tests were conducted
for no lower than 8m/s and no greater than 20m/s. The
drag area was assumed constant for any velocities be-
low 8m/s and over 20m/s in the simulations. The va-
lidity of the assumption of a constant drag area is de-
pendant on equipment and position, but the drag area
is more likely to increase slightly for lower velocities
based on the trend in wind tunnel test results in the Ap-
pendix. Velocities below 8m/s were measured at some
points during climbs and this leads to an underestima-
tion of drag in these hills, thus slightly overestimating
the velocity in the simulation. Also a significant uncer-
tainty is related to the instantaneous power produced
by the test rider. Even though the average power was
fairly consistent between test runs, a random variation
around the predefined power curve must be expected.
The MAE
P
row in Table 2 shows that the mean average
error of power input ranged from 19.2 to 43 watts.
Also, the power-slope function developed for this
simulation was based on a small data set. Ideally, the
power functions should be based on personalized his-
torical performance data for similar race conditions and
duration. Another limiting factor of the power curve is
that it does not account for a third dimension, namely
the length of the race. The average power of a short
time trial is significantly higher than that of a long time
trial, and the power curve should be scaled accordingly.
It is shown in Figure 4 that the simulated velocity
deviates the most from the measured velocity in sec-
tions where there is a transition between high and low
velocity, typically where the gradient changes quickly,
as over a crest and in the transition into a hill. A de-
lay was observed in the gradients recorded by the GPS
computer. Due to this delay the simulation will pro-
duce unrealistic values in such sections of terrain tran-
sition. This results in the simulated velocity curve hav-
ing close to the same shape as the measured velocity
curve, but being shifted slightly to the right. The effect
is visible from the 4000m mark and onward in Figure
4. More accurate altitude data of the course should lead
to more accurate simulations.
Because the wind conditions varied in between the
test runs, it is hard to determine with certainty that one
equipment setup is indeed faster than the other. The
trends observed in the measurements do however in-
dicate that Skinsuit 1 is superior to Skinsuit 2 while
riding the course in both directions. When simulating
both setups against each other with equal conditions,
Skinsuit 1 is predicted to be the faster one of the two.
Additionally, the wind tunnel tests results in the Ap-
pendix show that Skinsuit 1 gives a lower drag coeffi-
cient than Skinsuit 2. This indicates that the model is
able to predict which of the setups is the fastest.
In Table 2, results from simulations with a con-
stant drag area are included. Based on the wind tun-
nel tests results shown in the Appendix, the drag area
will vary with velocity (and Reynolds number). The
general tendency in these tests was that the predicted
time became slightly higher with a constant drag area.
It is believed that time trials spanning a greater velocity
interval will lead to greater differences between simu-
lations with or without a changing drag area. This will
also depend largely on the velocity profile and shape
of the C
D
-velocity curve. For the two skinsuits tested
here, the shape of the C
D
-velocity curves do not inter-
sect and consequently Skinsuit 1 will give a higher ve-
locity throughout the course in both directions.
From the total of six tests that were conducted, the
mean absolute error of 3.22% was within the frame
of the objectives. Because of the low sample size, in
which every test was unique in form of a different rider,
equipment setup or course, there is not much basis to
name the statistical uncertainty of the simulations. Nor
are there enough empirical results to claim that this
model of prediction is any more accurate than previous
models. However, despite the difficulty of performing
accurate field tests involving human test subjects, the
test results indicate that the fastest setup identified from
the wind tunnel tests, is also faster on the road. Also,
given accurate input data, the model should be able to
predict the fastest setup for otherwise similar race con-
ditions.
5 CONCLUSIONS
Based on the experiments conducted in this paper, the
expanded model for predicting road cycling perfor-
mance may be used to identify the best equipment setup
for any given time trial and rider. Simulations of the
conducted field tests showed a mean absolute differ-
ence of 3.22%, which was within the range of previous
icSPORTS 2019 - 7th International Conference on Sport Sciences Research and Technology Support
82