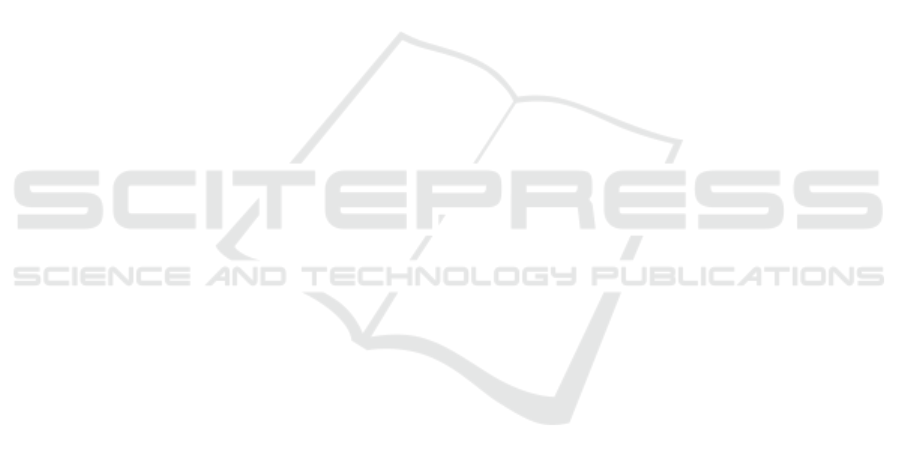
increasing the SN connectivity, where this increased
connectivity was also found to be negatively
correlated with mind-wandering task and resting
alpha rhythm (Ros et al., 2013), two conditions which
activate the DMN (Neuner et al., 2014; Simon &
Engström, 2015).
Our connectivity results also show different
connectivity patterns during baseline in different
groups. This difference might reflect the possibility
of using resting state connectivity to predict gaming
performance, where the similar idea has been
proposed in predicting the neurofeedback training
response in individuals with anxiety (Scheinost et al.,
2014).
In order to obtain more detailed connectivity
information, especially regarding the pattern changes
between the three major networks, more specific and
larger number of electrodes should be chosen for
connectivity analysis. Larger number of subjects and
different range of frequency bands are also required
to explore the different impact of interactive BCI
gaming in different frequency bands. An equal
number of electrodes for both players should be used
for the last gaming session to avoid bias by the
more/less dominant players.
5 CONCLUSIONS
Our study introduced a multi-user competitive BCI
game that is based on alpha operant conditioning. We
reported the preliminary results of the cortical
changes and connectivity from the most successful
gaming. Further development of this study will
include more participants and other social interaction
settings (i.e., collaborative), in order to explore the
brain activity and connectivity during the different
interaction tasks.
ACKNOWLEDGEMENTS
This study is funded by Indonesian Endowment Fund
for Education by the Ministry of Finance, Republic of
Indonesia.
REFERENCES
Baccalá, L. A., & Sameshima, K. J. B. c. (2001). Partial
directed coherence: a new concept in neural structure
determination. 84(6), 463-474.
Bazanova, O., & Vernon, D. (2014). Interpreting EEG
alpha activity. Neuroscience & Biobehavioral Reviews,
44, 94-110.
Bonnet, L., Lotte, F., & Lécuyer, A. (2013). Two brains,
one game: design and evaluation of a multiuser BCI
video game based on motor imagery. IEEE
Transactions on Computational Intelligence, 5(2), 185-
198.
Coan, J. A., & Allen, J. J. (2004). Frontal EEG asymmetry
as a moderator and mediator of emotion. Biological
psychology, 67(1-2), 7-50.
Cruz, I., Moreira, C., Poel, M., Ferreira, H., & Nijholt, A.
(2017). Kessel Run-A Cooperative Multiplayer SSVEP
BCI Game. Paper presented at the International
Conference on Intelligent Technologies for Interactive
Entertainment.
Davidson, R. J., Ekman, P., Saron, C. D., Senulis, J. A., &
Friesen, W. V. (1990). Approach-withdrawal and
cerebral asymmetry: emotional expression and brain
physiology: I. Journal of Personality Social
Psychology, 58(2), 330.
Goulden, N., Khusnulina, A., Davis, N. J., Bracewell, R.
M., Bokde, A. L., McNulty, J. P., & Mullins, P. G.
(2014). The salience network is responsible for
switching between the default mode network and the
central executive network: replication from DCM.
Neuroimage, 99, 180-190.
Greicius, M. D., Krasnow, B., Reiss, A. L., & Menon, V.
(2003). Functional connectivity in the resting brain: a
network analysis of the default mode hypothesis.
Proceedings of the National Academy of Sciences,
100(1), 253-258.
Gürkök, H., Nijholt, A., Poel, M., & Obbink, M. (2013).
Evaluating a multi-player brain–computer interface
game: Challenge versus co-experience. Entertainment
Computing, 4(3), 195-203.
Hecht, D. (2014). Cerebral lateralization of pro-and anti-
social tendencies. Experimental Neurobiology, 23(1),
1-27.
Homan, R. W., Herman, J., & Purdy, P. (1987). Cerebral
location of international 10-20 system electrode
placement. Electroencephalogr Clin Neurophysiol,
66(4), 376-382.
Konvalinka, I., Bauer, M., Stahlhut, C., Hansen, L. K.,
Roepstorff, A., & Frith, C. D. (2014). Frontal alpha
oscillations distinguish leaders from followers:
multivariate decoding of mutually interacting brains.
Neuroimage, 94, 79-88.
Korczowski, L., Barachant, A., Andreev, A., Jutten, C., &
Congedo, M. (2016). " Brain Invaders 2": an open
source Plug & Play multi-user BCI videogame. Paper
presented at the 6th International Brain-Computer
Interface Meeting (BCI Meeting 2016).
Korczowski, L., Congedo, M., & Jutten, C. (2015). Single-
trial classification of multi-user P300-based Brain-
Computer Interface using riemannian geometry. Paper
presented at the 2015 37th annual international
conference of the IEEE engineering in medicine and
biology society (EMBC).
CHIRA 2019 - 3rd International Conference on Computer-Human Interaction Research and Applications
64