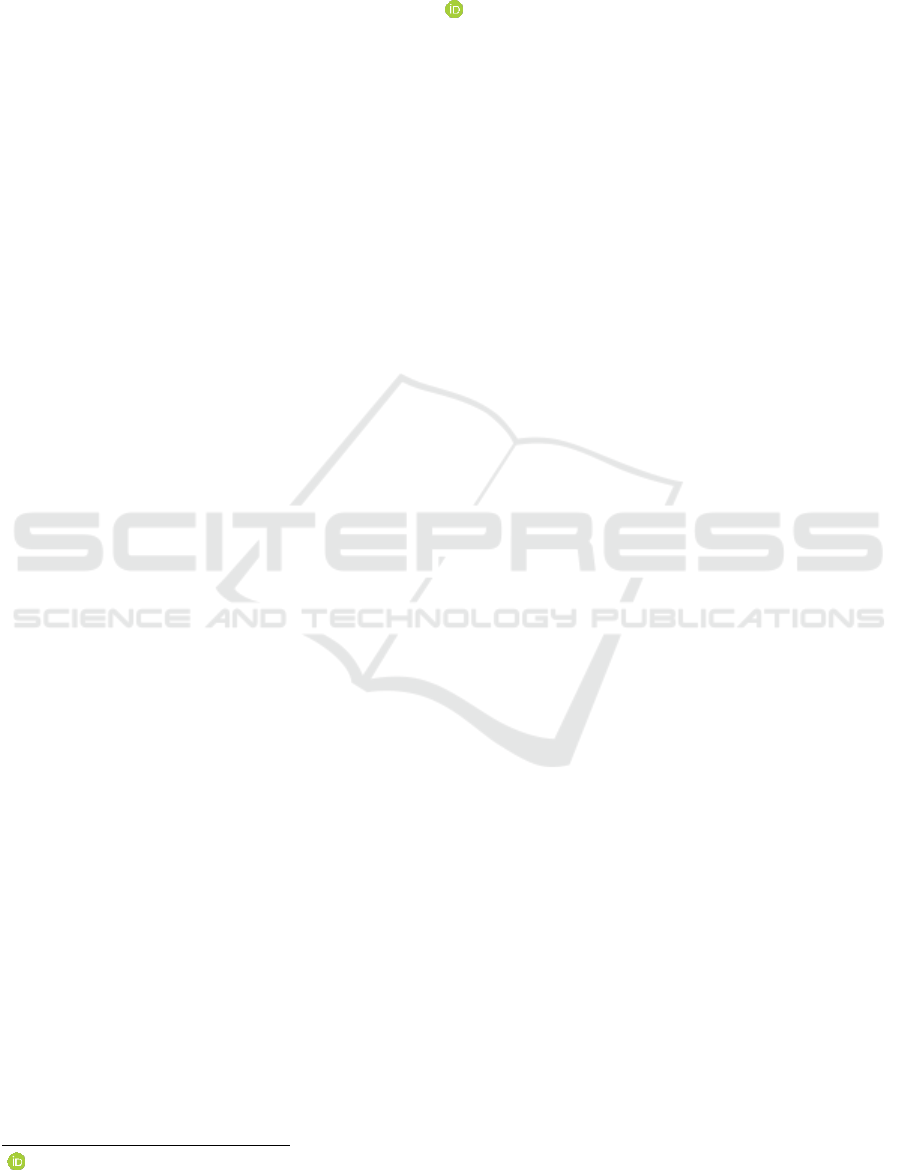
Fast and Streaming Analytics in ATM Cash Management
Terpsichori-Helen Velivassaki
a
and Panagiotis Athanasoulis
SingularLogic, Achaias 3 & Trizinias st., Kifisia, Attica, Greece
Keywords: Cash Management, Fast Analytics, Stream Analytics, Operational Database.
Abstract: Cash management across a network of ATMs can be greatly improved, exploiting a multitude of information
sources, which however generate huge datasets, not possible to be analysed via traditional methods. Business
Intelligence in the Banking, Financial Services and Insurance (BFSI) sector can be even more challenging
when exploitation of real-time information streams is desired. This paper presents the uCash ATM cash
management system, running on top of CloudDBAppliance, supporting fast analytics over historical data, as
well as streaming analytics functionalities over real-time data, served by its Operational Database. The paper
discussed integration points with the platform and provides integration hints, thus constituting a real use case
example of the CloudDBAppliance platform, allowing for its further exploitation in various application
domains.
1 INTRODUCTION
Even in the digital age, cash remains an essential
component of the payment system, being the largest
retail payment category by number of transaction for
transactions under $10 (Reserve Bank of Australia,
2017). During the last few years, in their attempt to
achieve mobility to better serve their customers,
banks across the globe are investing on next-
generation ATMs, featuring advanced capabilities
and offering customers the ability to perform more
types of financial transactions than the ones are
currently handled by customer service
representatives. This will create a whole new
perspective of the user ATM experience, also
increasing the spatiotemporal heterogeneity of the
cash demand and the data produced by the ATMs,
derived from the new business value propositions of
these new ATMs (ATM Industry Association, 2018).
Additionally, as user mobility increases, so does the
complexity of predicting the expected user
withdrawal and deposit transactions volumes.
Banks and financial institutes nowadays face
significant challenges in managing effectively ATM
replenishment, based primarily on static prediction
algorithms. This results in high cash-out rates in
certain ATMs, while having excess leftover cash in
other ATMs, returned to banks. Accordingly,
a
https://orcid.org/0000-0002-0362-4607
ineffective cash management in ATMs leads to
considerable customer dissatisfaction due to delays
until reloading empty ATMs, as well as complex and
expensive cash logistics to reload the network.
Ideally, smart cash management should be able to
catch cash demand, affected by external factors and
not easily predictable, as e.g. the monthly
salaries/pensions. For example, an ATM, located on
the same level of a large shopping Mall as a store with
special discounts for a single day only, could have
increased cash flow. Also, cash demand in ATMs
close to social events is expected to be higher than
usual. Moreover, ATM traffic could be affected by
combined factors, such as weather and location.
Indicatively, ATMs close to beaches/seaside might
realize increased demand during warm and sunny
days or cash demand could decreased for any ATM
during cold days. Another dependence can be found
at seasonality, e.g. ATM cash demand is expected to
grow during the days before bank holidays.
In this context, appropriate cash management is
bound to ultra-fast analytics over huge datasets,
produced by large interconnected ATM channel
networks. Business objectives mandate for the
capability of processing complex queries over
thousands of columns of operational data, created by
thousands of ATMs in the order of seconds.
On the other hand, strong data privacy and
454
Velivassaki, T. and Athanasoulis, P.
Fast and Streaming Analytics in ATM Cash Management.
DOI: 10.5220/0008318504540458
In Proceedings of the 8th International Conference on Data Science, Technology and Applications (DATA 2019), pages 454-458
ISBN: 978-989-758-377-3
Copyright
c
2019 by SCITEPRESS – Science and Technology Publications, Lda. All rights reser ved