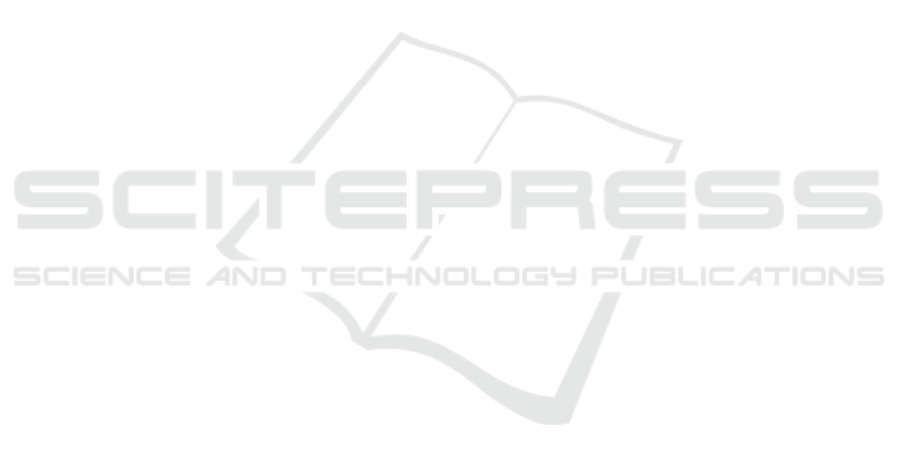
REFERENCES
Bontcheva, K., Derczynski, L., Funk, A., Greenwood,
M. A., Maynard, D., and Aswani, N. (2013). TwitIE:
An Open-Source Information Extraction Pipeline for
Microblog Text. In Proceedings of the International
Conference Recent Advances in Natural Language
Processing, pages 83–90.
Cai, H., Yang, Y., Li, X., and Huang, Z. (2015). What are
Popular : Exploring Twitter Features for Event Detec-
tion , Tracking and Visualization. In Proceedings of
the 23rd ACM international conference on Multime-
dia, pages 89–98.
Cunningham, H., Maynard, D., and Bontcheva, K. (2011).
Text processing with gate. Gateway Press CA,
Sheffield, UK.
Firas Odeh (2018). Event detection in heterogeneous data
streams. Technical report, Lyon.
Gaillard, M. and Egyed-Zsigmond, E. (2017). Large scale
reverse image search-A method comparison for al-
most identical image retrieval. In INFORSID, pages
127–142.
Gobbel Dr, G. T., Garvin, J., Reeves, R., Cronin, R. M.,
Heavirland, J., Williams, J., Weaver, A., Jayarama-
raja, S., Giuse, D., Speroff, T., Brown, S. H., Xu, H.,
and Matheny, M. E. (2014). Assisted annotation of
medical free text using RapTAT. Journal of the Ameri-
can Medical Informatics Association, 21(5):833–841.
Guille, A. and Favre, C. (2014). Mention-anomaly-based
Event Detection and tracking in Twitter. In ASONAM
2014 - Proceedings of the 2014 IEEE/ACM Interna-
tional Conference on Advances in Social Networks
Analysis and Mining, pages 375–382.
Hardeniya, N., Perkins, J., Chopra, D., Joshi, N., and
Mathur, I. (2016). Natural Language Processing:
Python and NLTK. Packt Publishing Ltd, Sebastopol,
CA.
Honnibal, M. and Montani, I. (2017). spacy 2: Natural lan-
guage understanding with bloom embeddings, convo-
lutional neural networks and incremental parsing.
Hu, X., Tang, J., Gao, H., and Liu, H. (2013). ActNeT: Ac-
tive Learning for Networked Texts in Microblogging.
Katragadda, S., Virani, S., Benton, R., and Raghavan, V.
(2016). Detection of event onset using Twitter. In
Proceedings of the International Joint Conference on
Neural Networks, pages 1539–1546.
Lieberman, H., Patern
`
o, F., Klann, M., and Wulf, V. (2006).
End-User Development: An Emerging Paradigm. In
Lieberman, H., Patern
`
o, F., and Wulf, V., editors, End
User Development, chapter 1, pages 1–8. Springer
Netherlands, Dordrecht.
Makki, R., Carvalho, E., Soto, A. J., Brooks, S., Oliveira,
M. C. F. D., Milios, E., and Minghim, R. (2018). ATR-
Vis: Visual and Interactive Information Retrieval for
Parliamentary Discussions in Twitter. ACM Transac-
tions on Knowledge Discovery from Data, 12(1):33.
McCallum, A. (2002). MALLET: A Machine Learning for
Language Toolkit. http://mallet.cs.umass.edu.
Miller, B., Linder, F., and Mebane Jr, W. R. (2018). Active
Learning Approaches for Labeling Text. Technical re-
port, University of Michigan, Ann Arbor, MI.
ˇ
Reh
˚
u
ˇ
rek, R. and Sojka, P. (2011). Gensim - Statistical Se-
mantics in Python. In EuroScipy.
Settles, B. (2009). Active learning literature survey. Techni-
cal report, University of Wisconsin-Madison Depart-
ment of Computer Sciences.
Spina, D., Peetz, M.-H., and de Rijke, M. (2015). Active
Learning for Entity Filtering in Microblog Streams.
pages 975–978. ACM New York.
Trivedi, G., Pham, P., Chapman, W. W., Hwa, R., Wiebe,
J., and Hochheiser, H. (2018). NLPReViz: An inter-
active tool for natural language processing on clinical
text. Journal of the American Medical Informatics As-
sociation, 25(1):81–87.
WEBIST 2019 - 15th International Conference on Web Information Systems and Technologies
160