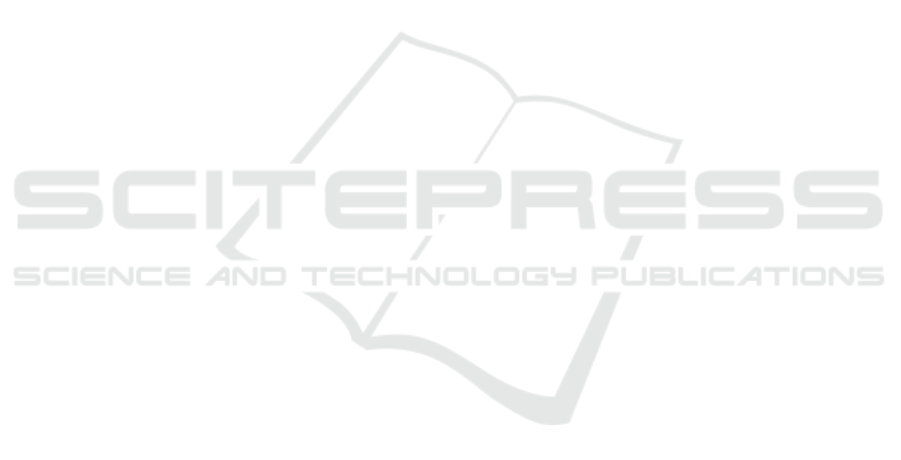
initial proposition, it would be plausible to consider
simultaneous, localized HiAlpha suppression
together with HiBeta reductions as a sign of neural
efficiency. The reasoning behind this thought builds
on the proposition of HiAlpha reflecting task-specific
information processing (Klimesch, 1999), and Beta
reflecting increased local communication (Buzsáki
and Draguhn, 2004). Therefore, HiAlpha suppression
could be indicative of a cortical region being recruited
to process a particular task, while at the same time a
reduction in local Beta would reflect a reduction in
communication among local neuron populations. In a
similar manner as frontal Theta and widespread Beta
increases are considered as coping mechanisms
during (too) hard tasks (Sauseng et al., 2005), a
reduction of local Beta might be indicative of the fact
that local regions are coping well and only recruit
absolutely required neuron groups.
Besides these theoretical potentials, the remaining
present findings show an alternative practical
potential to differentiate levels of perceived difficulty
(and thereby indirectly flow) through feature
combination. In this regard, it should be pointed out
first, that barely any significant changes were
detected in the Theta band. This was an unexpected
finding, as a large amount of literature is available
documenting frontal Theta increases with increasing
task demands (Klimesch, 1999; Borghini et al., 2014).
As to why such a pattern is here only reflected in trend
level temporal Theta power changes, several
explanations are possible. Theta changes have been
documented to occur strongly with prolonged task
exposure (e.g. in studies with airplane simulators –
Borghini et al., 2014). By adding the first task round
(the task introduction using the EASY treatment), to
a one-way repeated-measures ANOVA we do find a
difference for frontal Theta at F3 & F4 (F-M) (F(4,
104) = 4.33, p < .01,
2
G
= .05), with the lowest Theta
power in the introduction phase. This points to the
fact that time might have acted as a confounding
effect on Theta power changes. Further, frontal Theta
effects are typically identified in midline positions
(e.g. Fz – see Ewing et al., 2016). Thus, as these
electrodes are missing for the Epoc+ headset, it might
not be possible to find Theta effects in some EEG
devices. This is a limitation that could easily occur in
real-world measurement scenarios using portable
EEG systems with few electrodes.
However, employing the frequency separation
approach, an interesting pattern emerged in that some
narrow frequency features showed significant
reaction to changes in perceived difficulty, with some
indicating stepwise, monotonous increases, some
indicating increases with moderate level plateaus for
OPTIMAL conditions and some indicating maxima
for either the EASY or the HARD condition when
compared to all other conditions. The functional
explanation of these patterns should be the subject of
future work as would be the development of sensitive
and robust compound indices, ideally based on more
than one task type (see e.g. Berka et al., 2007).
Presently, it is primarily argued that these patterns
allow to discuss flow related changes in a refined
manner and that they pose interesting alternatives for
the detection of situations of optimal difficulty,
especially in scenarios where less information might
be available than typically is in laboratory setups (e.g.
fewer and unevenly distributed electrodes). When
considering how for example neuro-adaptive systems
employ thresholds to inform adaptation rules (cf. e.g.
Ewing et al., 2016), features indicating maxima
during EASY or HARD conditions could be valuable
indicators, given that they would be subject to lower
variation except in the boundary cases. In this regard
they could firstly be employed to robustly identify
when difficulty is unbalanced and flow unlikely.
In conclusion, this study posits that flow research
could benefit from nuanced frequency power
analyses, in general by identifying Alpha and Beta
power changes that could relate to neural efficiency
(in a local form), and in particular when (portable)
EEG systems are used that lack midline electrodes. In
line with previous research (Klimesch, 1999), the
herein presented initial analyses support the
understanding that a personalized and narrow
frequency power analysis helps to avoid to miss
frequency specific effects. Specifically, this research
contributes to the literature on flow by highlighting
that frontal medial HiAlpha decreases in increased
task difficulty (and HiBeta decreases during
optimally balanced task difficulties) as well as
widespread HiBeta increases in very hard task
conditions provide additional avenues to
automatically and unobtrusively detect boundary
situations to flow. Thus, these metrics, indirectly
allow to improve the identification of situations with
optimal preconditions for flow to emerge.
Importantly, the frequency segmentation approach
would appear to be a valuable alternative when
portable EEG systems are used that don’t include
midline electrodes. As an additional effect, Alpha and
Beta bands appear unaffected by influences from task
exposure durations providing an interesting
alternative to the more established metric of frontal
midline Theta power increases with higher task
demands (e.g. Ewing et al., 2016). Ideally by taking
narrow frequency power analysis into account, future
flow research will thus move closer to identifying
Flow and Optimal Difficulty in the Portable EEG: On the Potentiality of using Personalized Frequency Ranges for State Detection
189