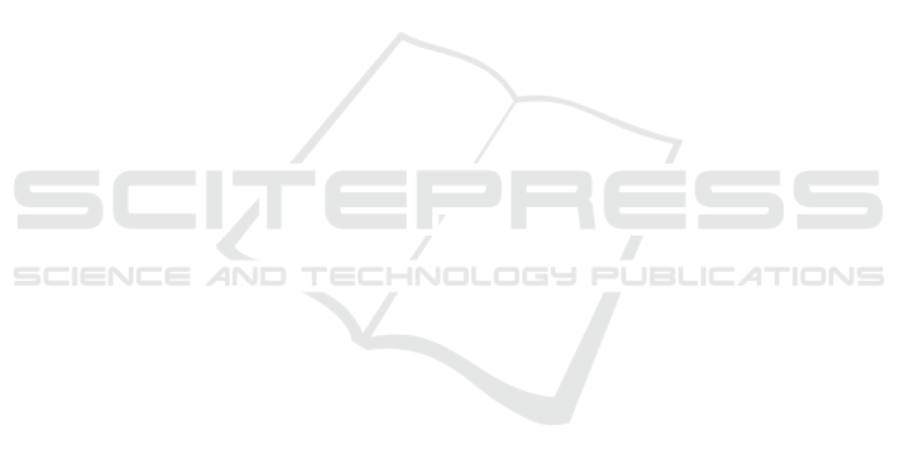
5 CONCLUSIONS
The paper presents the forecasting analysis of airline
passenger demand for the long-haul routes at Garuda
Indonesia, the legacy airline of Indonesia. We
compare two forecasting methods, which are suitable
for the purpose, namely an adjusted regression model
from Carreira et al. (2017) and Winter’s model with
optimal smoothing constants.
The results show that the regression model is
more suitable for Saudi Arabia route, while Winter’s
model is more suitable for China route as indicated
by the forecast error values.
The results may be due to the fact that China
demand shows a seasonal pattern in each year, while
for Saudi Arabia route, the passenger demand is
mainly depending on the Hajj season and Ramadan
season which are moving forward in approximately
10 days every year.
Forecast results for 2019-2028 show that the
demand for Saudi Arabia and China grow at the rate
of 6% and 9% and reach 900.000 and 1.6 million
passengers in 2028 respectively. The results imply
that Garuda Indonesia must anticipate the growth by
increasing the seating capacity by increasing the
flight frequency and/or using larger aircraft.
This study has limitations. Even though demand
data from Garuda Indonesia is monthly data,
however, the population data in annual data, thus,
forecasting is conducted using annual data, that may
not accurately reflect the actual situation.
Future research may include using the resulting
forecast for strategic fleet planning, to maximize the
profitability of the airline.
ACKNOWLEDGEMENTS
We would like to thank Garuda Indonesia, especially
the Market Research Department for their support in
this study.
REFERENCES
Airbus. (2013) Future Journeys. Global Market Forecast
2013. Blagnac Cedex, France.
Boeing (2013) ‘Current Market Outlook 2013-2032’,
Boeing-Website, pp. 2013–2014. Available at:
http://www.boeing.com/boeing/commercial/cmo/.
Carreira, J. S., Lulli, G. and Antunes, A. P. (2017) ‘The
airline long-haul fleet planning problem: The case of
TAP service to/from Brazil’, European Journal of
Operational Research. Elsevier B.V., 263(2), pp. 639–
651. doi: 10.1016/j.ejor.2017.05.015.
Chen, Z., Tzeremes, P., Tzeremes, N.G. (2018)
Convergence in the Chinese airline industry: A
Malmquist productivity analysis. Journal of Air
Transport Management, pp. 77-86.
Chopra, S., Meindl, P. (2016) Supply Chain Management
Strategy Planning and Operation, 6
th
Ed. Essex:
Pearson.
Flyvbjerg, B., Skamris, M. K., Buhl, S. L. (2005) How (in)
accurate are demand forecasts in public works projects?
The case of transportation, 131-146.
Gelhausen, M. C., Berster, P. and Wilken, D. (2018) ‘A new
direct demand model of long-term forecasting air
passengers and air transport movements at German
airports’, Journal of Air Transport Management.
Elsevier Ltd, 71(April), pp. 140–152. doi:
10.1016/j.jairtraman.2018.04.001.
Heizer, J., Render, B., Munson, C. (2017). Operation
Management Sustainability and Supply Chain
Management, I2th Ed, Boston: Pearson.
Hsu, C. I. et al. (2011) ‘Aircraft replacement scheduling: A
dynamic programming approach’, Transportation
Research Part E: Logistics and Transportation Review.
Elsevier Ltd, 47(1), pp. 41–60. doi:
10.1016/j.tre.2010.07.006
Jakarta Post. (2018) Chinese tourists' favorite places in
Indonesia. [Online] Available at:
https://www.thejakartapost.com/travel/2018/03/02/chi
nese-tourists-favorite-places-in-indonesia.html
[Accessed 19th January 2019].
Klker, K., Bießlich, P. and Ltjens, K. (2016) ‘From
passenger growth to aircraft movements’, Journal of
Air Transport Management, 56(Part B), pp. 99–106.
doi: 10.1016/j.jairtraman.2016.04.021.
Pyke, D. and Sibdari, S. (2018) ‘Risk Management in the
Airline Industry’, Finance and Risk Management for
International Logistics and the Supply Chain, pp. 293–
315. doi: 10.1016/b978-0-12-813830-4.00012-5.
Susanty, F., Ramadhani, N. F. (2017) Indonesians love of
Mecca boosts lucrative ‘umrah’ business. [Online]
Available at: https://www.thejakartapost.com/ news/
2017/01/21/indonesians-love-of-mecca-boosts-lucrative
-umrah-business.html [Accessed 18th January 2019].
Wiesner, J. D., Choon, K., Leong, G. K. (2019). Principles
of Supply Chain Management, 5
th
Ed, Mason: Cengage
Learning.
Worldometers, 2019. http://www.worldometers.info.
[Online]Available at: http://www.worldometers.info
/world-population/china-population/ [Accessed 15
january 2019].
Worldometers, 2019. http://www.worldometers.info.
[Online] Available at: http://www.world
ICIB 2019 - The 2nd International Conference on Inclusive Business in the Changing World
536