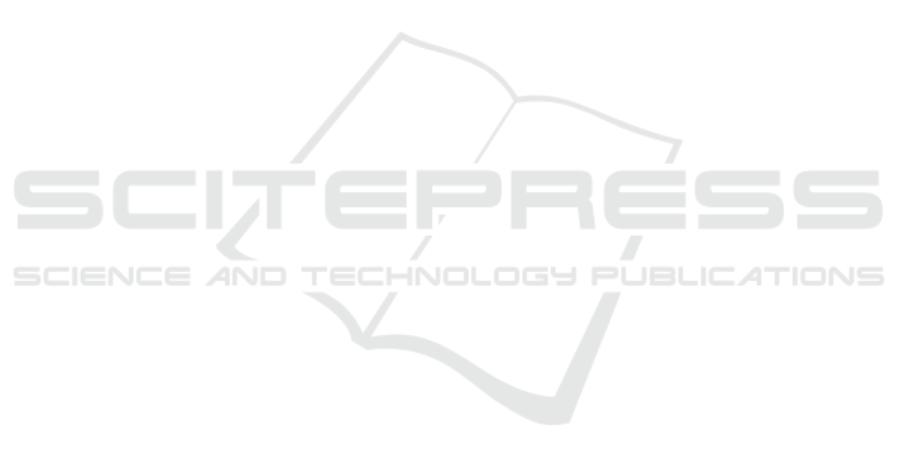
Their models significantly improved the fitting
performance of the postseismic time series and the
prediction performance of the evolution of
postseismic deformation. (F.L. Ren, et al, 2018)
adopted the optimized multiple linear regression
model and grey GM(1,1)model to forecast the power
demands of Shaanxi Province in recent years and
obvioused that the prediction model has the high
accuracy. To improve the prediction accuracy of
bridge structure deformation. (J.Z. Xin, et al, 2018)
based on data mining and to accurately evaluate the
time-varying characteristics of bridge structure
performance evolution, this paper proposes a new
method for bridge structure deformation prediction,
which integrates the Kalman filter, Autoregressive
Integrated Moving Average Model (ARIMA), and
Generalized Autoregressive Conditional
Heteroskedasticity (GARCH). Finally, this paper
provides a new way for structural behavior
prediction based on data processing, which can lay a
foundation for the early warning of bridge health
monitoring system based on sensor data using
sensing technology. (Y. Han, 2016) used grey
prediction theory to construct the grey model GM (1,
1) and linear regression weighted combination
model, used to predict the settlement of subgrade.
The studies show that the model has a certain
practical application value and provides a new way
for the study of subgrade settlement prediction. (D.
Liang, et.al, 2014) verified the feasibility of this
combination model is verified through the example.
It can obtain higher prediction accuracy by
collecting relative little data. The combination model
has a lot of advantages in the machine tool sales
forecast. (K.P. Bi, et al, 2016) verified the gray-linear
regression combined model is proved to be valid and
more accurate forecasting method compare with
single forecasting model through practical example.
(B. Zeng, et al, 2013) used the inverse accumulation
generator of the original sequence, the non-
homogeneous exponential incremental sequence is
transformed into homogeneous exponential
incremental sequence, and then a DGM (1, 1) model
is established using the new sequence. Finally, two
examples are given to illustrate the simplicity,
practicability and operability of the model. (N. Tian,
Y. Wei. 2018) Based on the direct modeling of the
approximate non-homogeneous exponential discrete
grey model, this paper constructs a new grey
forecasting model, which not only improves the
modeling accuracy of approximate non-
homogeneous exponential sequences, but also
extends the application scope to such cases as the
combination of the increasing-decreasing sequence.
Finally, examples are employed to verify the
effectiveness and feasibility of the proposed method.
(Y.X. Jiang, Q.S. Zhang, 2015) Based on the recursive
solution to unbiased GM (1, 1) model, proposed the
method of time series piecewise representation. The
results show that the recursive model has higher
fitting precision, and also verify the effectiveness
and the practicability of the representation method of
time series based on the grey forecasting model.
(H.R.Zhao, X.Y.Han, S.Guo, 2018) presented a hybrid
annual peak load forecasting model (MVO-DGM (1,
1). The model uses the latest optimization algorithm
MVO (multi verse optimizer) to determine the two
parameters of DGM (1, 1) model, and then uses the
optimized DGM (1, 1) model to predict the annual
peak load. Taking the annual peak load of Shandong
Province from 2005 to 2014 as an example, the
analysis results show that the DGM (1, 1) model
parameter determination method based on MVO
algorithm has significant advantages over the least
square estimation method, particle swarm
optimization and Drosophila optimization algorithm.
In this paper, the CSI 5200 Three Vector Oil
Analyzer is used to detect and analyze the oil of
passenger cars of different grades, and the number of
wear particles in the oil is obtained, and then the
degree of deterioration of engine oil quality and the
wear condition of the engine are judged according to
the cleanliness grade. Because the analysis cost of
deterioration degree of passenger locomotive oil is
higher, the analysis period is longer, and the data
obtained is relatively less, the grey model is selected
to predict the deterioration degree of passenger
locomotive oil. The grey model is suitable for data
prediction in exponential form, which is not
consistent with the linear rule of actual data.
Therefore, this paper chooses the combination of
grey model and linear regression model to form a
new combination model for forecasting and analysis,
which can not only make up for the lack of linear
law in grey model, but also give full play to the
advantages of grey model, thus improving the
limitations of single model and improving the
accuracy of forecasting (J.F. Jing, K.C. Li, F.K. Deng,
et al, 2015). Due to the different temperatures, loads
and rotational speeds, the chemical composition of
the wear particles is likely to be not the same, which
also has an impact on the number of wear particles.
In this paper, the prediction of wear particles is
carried out under the same wear mechanism.
Application of Grey Linear Regression Combined Model in Predicting the Motor Oil Wear Particles for Passenger Cars
39