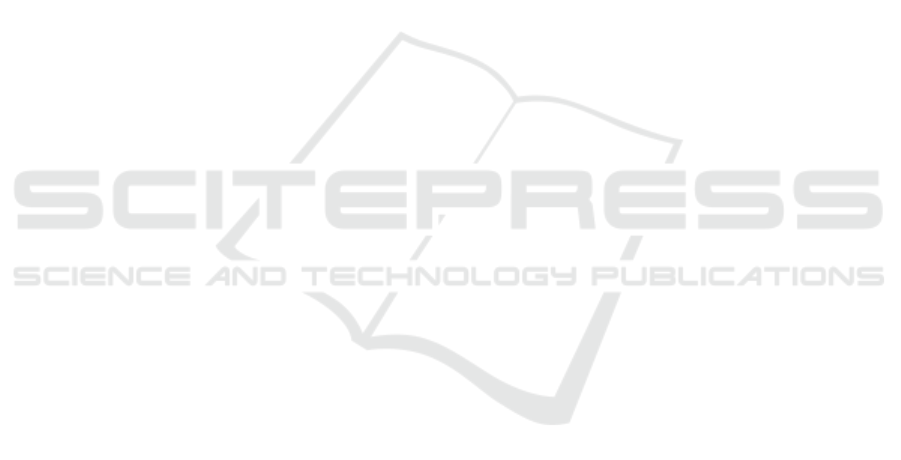
ACKNOWLEDGEMENTS
This research was supported by the Science and
Technology Projects of Test & Maintenance Center
of CSG EHV Transmission Company (Grant No.
CGYKJXM20160025).
REFERENCES
Bolin, P., and Koch, H.. 2005. Introduction and
applications of gas insulated substation (GIS), IEEE
Power Engineering Society General Meeting.
Кучинский, Г. С., 1984. Partial discharge of high voltage
electrical equipment, China Water Conservancy and
Electric Power Press.
Kang, H. , Qi, Y. , Liu, G., et al., 2012. Application of the
ARMA Model in Non-stationary Vibration Signals.
International Conference on Quality, IEEE..
Li. J., Sun, C.X., Du, L., et al., 2002. Study on Fractal
Dimension of PD Gray Intensity Image. Proceedings
of the CSEE, 22, p. 123-127,.
Liu, Y.P., Lv, F.C., and Li, C.R., 2004. Study on pattern
spectrum of patial. Proceedings of the CSEE, 24,
p.179-183.
Liu, Y. P., Wang, Z. J., Li, Y. S., 2013. Study on the
Insulating Spacers Surface Discharge of GIS. Applied
Mechanics and Materials, 385-386, p. 1209-1212.
Martin, Y., Li, Z., Tsutsumi, T., et al.,2012. Detection of
SF6 Decomposition Products Generated by DC
Corona Discharge Using a Carbon Nanotube Gas
Sensor. IEEE Transactions on Dielectrics and
Electrical Insulation, 19, p. 671-676.
Okubo, H., Kato, T., Hayakawa, N., et al., 1998. Temporal
Development of Partial Discharge and Its Application
to Breakdown Prediction in SF/sub 6/ gas. IEEE
Transactions on Power Delivery, 13, p. 440-445.
Pharmatrisanti, A., Meijer, S., and Smit, J. J., 2004.
Probability of Partial Discharge Detection in Aged
GIS due to Void in Epoxy. Gaseous Dielectrics X.
Springer US.
Qiu, Y.C., 1994. GIS device and its insulation technology,
China Water Resources and Electric Power Press.
Qi, B., Li, C.R., Hao, Z., et al., 2011. Evolution
phenomena and features of surface partial discharge
initiated by immobilized metal particles on GIS
insulators, Proceedings of the CSEE, 31(1), p. 101-108.
Qi, B., Li, C.R., Xing, Z.L., et al., 2014. Partial Discharge
Initiated by Free Moving Metallic Particles on GIS
Insulator Surface: Severity Diagnosis and Assessment.
IEEE Transactions on Dielectrics and Electrical
Insulation, 21, p. 766-774.
Ren, M., Dong, M., and Qiu A.C., 2014. Partial
Discharges in SF6 Gas Filled Void under Standard
Aperiodic and Oscillating Switching Impulses. IEEE
Transactions on Dielectrics and Electrical Insulation,
21, p. 262-272.
Strachan, S.M., McArthur, S.D.J., Judd, M.D., et al, 2005.
Incremental Knowledge-based Partial Discharge
Diagnosis in Oil-filled Power Transformers.
Intelligent Systems Application to Power Systems, 130,
p.181-186.
Tang, Z.G., Tang, M.Z., Li, J.Z., et al, 2017. Review on
Partial Discharge Pattern Recognition of Electrical
Equipment. High Voltage Engineering, 7, p.173-187.
Yang, Q., Chen, S.Z., Shen, S.M., et al., 2018.
Adaptability of LSTM Network and ARMA Modeling
to Random Error Prediction of Inertial Devices.
Electronics Optics & Control, 25, p. 68-72.
Zhou, Q., Tang, J., Tang, M., et al, 2006. Mathematical
Model of Four Typical Defects for UHF Partial
Discharge in GIS. Proceedings of the CSEE, 26, p. 99-
105.
Application of ARMA Model in Prediction of Development Trend of Partial Discharge
397