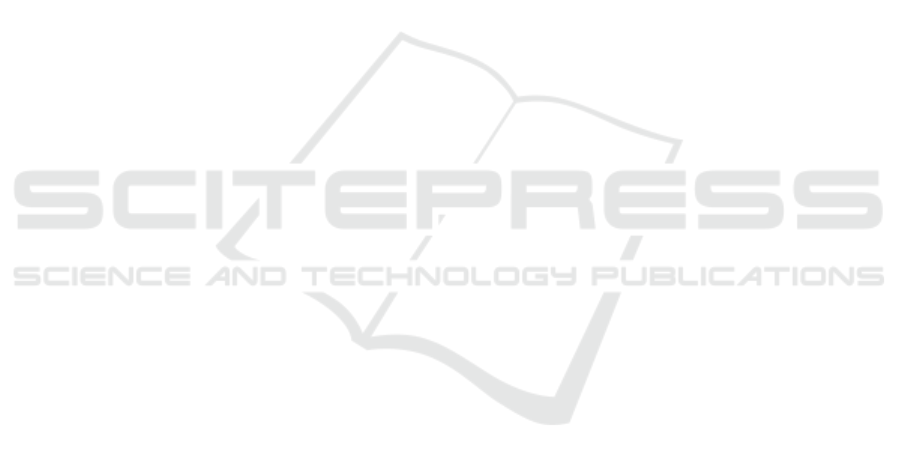
research in neural network engineering has shown
that neural networks have the properties needed for
relevant applications, such as nonlinearity and fine
interpolation, the ability to learn nonlinear complex
mapping, and self-adaptation for different statistical
distributions.
However, neural networks cannot be used to
explain the causal relationship between input and
output variables. This is because the black box is
like the natural of most neural network algorithms.
Neural networks cannot be named with the underlying
knowledge. Networks must learn from the beginning,
while the learning process itself does not guarantee
success.
On the other hand, the expert system’s fuzzy
approach has been applied to the forecasting
of different problem (Bolloju, 1996), (Kaneko,
1996), (Shaout and Al-Shammari, 1998), where the
operator’s knoeledge to predict results. Although
forecasting is based on Fuzzy logic, the results
show that the process for constructing fuzzy- logic
system is subjective and depends on the heuristic
process. The choice of membership function and
basic rules must be developed heuristically for each
case. Rules in this way do not always produce
the best predictions, and the choice of membership
function still depends on trial and error. The
strengths and weaknesses of Neuro-fuzzy and fuzzy
logic, have combined the ability to learn from neural
networks and the functions of fuzzy expert systems.
Application can be found in (Dash et al., 1995),
(Studer and Masulli, 1997), and (Padmakumari et al.,
1999). For example the hybrid model is expected to
provide understanding to humans about the meaning
of ’Fuzzy’ through the various advantages can be
used as a concept of knowledge by studying neural
networks.
Some researchers such as (Jacobs and Levy,
1989), have claimed that the stock market is not a
system that can be explained by simple rules, nor
is it a random system that is impossible to predict.
In fact, they claim that the market is a complex
system, where the behaviour of the system can be
only be explained and predicted by a complex set of
relationships between variables.
Recognizing the complex characteristics of the
stock market to invites the researchers to further
investigate whether index variations can be improved
to predict nonlinear models using the neuro-fuzzy
approach. (Lin et al., 2002) in the ”Can the
neuro-fuzzy models predict stock indexes better than
its rivals?” Develop a model based on a trading
system by using a neuro fuzzy model to predict
stock indexes better. Thirty well-known stock indexes
were analyzed with the help of the developed model.
Empirically shows the corresponding non-linear
results in stock indexes using the KD technical index.
Analysis of trading points and analysis of trade costs
indicate endurance and opportunities for profit, it is
recommended to use nonlinear neuro fuzzy systems.
The analysis also shows that the recommended neuro
fuzzy is consistent over time.
In 2003, (
¨
Ozkan et al., 2004) in the ”Currency
Crises Analyzed By Type-I Fuzzy System
Modelling”, implementing softcomputing in the
analysis of a currency crisis, with test data time series
of data is the currency of Turkey. The method used
is the approach of macroeconomic time series data,
the Rule-based Fuzzy System Modelling (RBFSM)
become the focus of research and compared to
GARCH /ARMAX and ANFIS. The results show
that the GARCH approach / ARMAX and ANFIS no
better than predicted RBFSM.
Achsani, (2003) using a fuzzy cluster algorithm
to model the demand for Indonesia with the data in
1993: 4 to 2002: 3 even though the results are not
as good as the econometric model approach because
it does not consider the effect of autocorrelation and
seasonality of data, however, can explain in more
detail the grouping of economic periods. In the
same year, Achsani, (2003) back to do research using
Fuzzy-Clustering in data ASEAN + 3 as the ratio of
debt / GDP, exchange rate stability, inflation rate, and
the long term interest rate to determine the relative
position of Indonesia in the constellation of Asian
economies East.
In previous years some researchers report that
their concern for the problems of economic crisis.
They are concerned about theadverse consequences
of the policies needed to maintain economic variables
(Agenor et al., 1992; Flood and Marion, 1998;
Flood and Marion, 1998). While the traditional
approach emphasizes the role played by a decline in
foreign reserves in triggering the collapse of the fixed
exchange rate, some of the latest models suggest that
the decision to abandon the parity may occur based
on concerns about the evolution of the economic
authorities. On the other hand, variables indicate that
groups of other variables can be useful for predicting
the currency crisis (Ozkan and Sutherland, 1995) and
(Velasco, 1987). In addition, the latest model also
suggested that the crisis can develop in the absence
of fundamental changes in the real economy. This
model emphasizes that the nature of the contingency
of economic policy may pose some equlibria and
produce that meets its own crisis (Obstfeld, 1983).
Some recent research has focused on the effects of the
balance of payments crisis (Eichengreen et al., 1995).
ICoSEEH 2019 - The Second International Conference on Social, Economy, Education, and Humanity
84