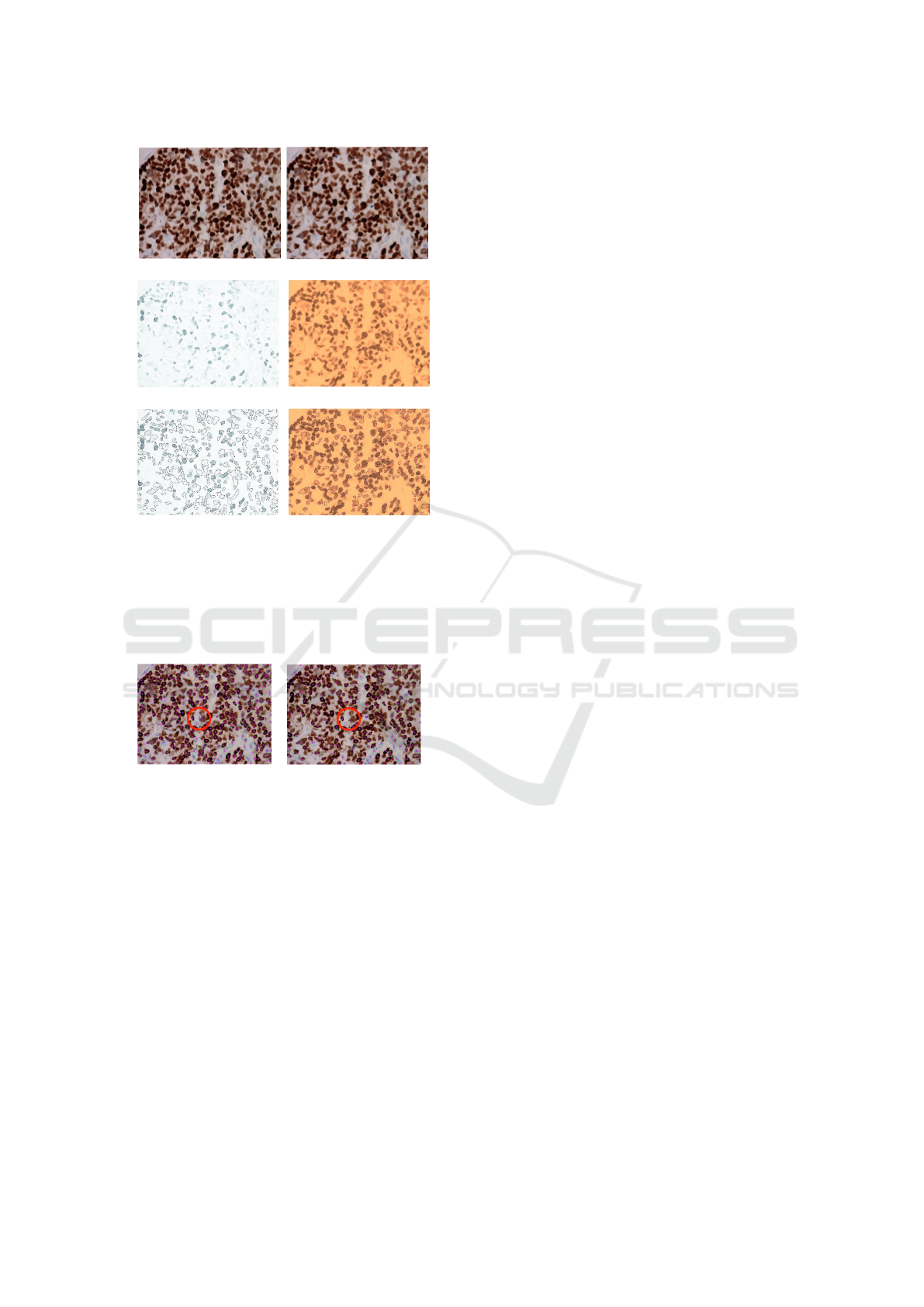
(a)
(b)
(c)
(d)
(e)
(f)
Figure 4: The Stage of IHC Nucleus Image Segmenta-
tion Stage of Breast Cancer (a) Image of estrogen recep-
tor (b) Image Resulted by Median filtering (c) Image H Re-
sulted by colour Deconvolution (d) Image DAB Resulted by
Colour Deconvolution (e) Image H Resulted by Watershed
(f) Image DAB Resulted by Watershed
(a) (b)
Figure 5: Image of the result of using (a) 3x3 Kernel Size
(b) 1x1 kernel size
Figure 5 is the result of cell segmentation in connec-
tive tissue using a 3x3 and 1x1 disk kernel. It is seen
that cells in the connective tissue are still counted as
many cancer cells while using a 1x1-sized kernel seen
in cells in the connective tissue there are not many
counts.
Based on the results seen in figure 5, the use of
3x3 and 1x1 kernels has indeed succeeded in remov-
ing cells in the connective tissue, but not all cells in
the connective tissue can be identified. This research
will be continued by using other methods to remove
cells in connective tissue. If this step has been com-
pleted, then the next process until cell count can be
done.
4 CONCLUSIONS
From the steps that have been done above, some re-
sults are obtained, namely stages of digital image pro-
cessing to read IHC breast cancer images to obtain
H cell counts and positive DAB cell numbers begin-
ning with the pre-processing process using Median
Filtering, then proceed with colour segmentation us-
ing Colour Deconvolution to obtain IHC H images
and positive DAB IHC images and followed by cell
segmentation using Watershed Markers. The use of
3x3 and 1x1 kernels has indeed succeeded in remov-
ing cells in the connective tissue, but not all cells in
the connective tissue can be identified. This research
will be continued by using other methods to remove
cells in connective tissue. If this step has been com-
pleted, then the next process until cell count can be
done.
ACKNOWLEDGEMENTS
High appreciation should be given to Universitas Is-
lam Riau (UIR) and Universiti Teknologi Petronas
(UTP) for their support in matching grant of this re-
search work.
REFERENCES
Akbari, H., Uto, K., Kosugi, Y., Kojima, K., and Tanaka, N.
(2011). Cancer detection using infrared hyperspectral
imaging. Cancer science, 102(4):852–857.
Calhoun, M. E., Chowdhury, S., and Goldberg, I. (2019).
Medical analytics system. US Patent 10,255,997.
Estrogen, S. C. I. R. Kanker payudara menggunakan marker
watershed.
Gonzalez, R. C., Woods, R. E., et al. (2002). Digital image
processing.
Kadir, A. (2017). Teori dan aplikasi pengolahan citra.
Kostopoulos, S., Cavouras, D., Daskalakis, A., Bougioukos,
P., Georgiadis, P., Kagadis, G. C., Kalatzis, I., Rava-
zoula, P., and Nikiforidis, G. (2007). Colour-texture
based image analysis method for assessing the hor-
mone receptors status in breast tissue sections. In 2007
29th Annual International Conference of the IEEE
Engineering in Medicine and Biology Society, pages
4985–4988. IEEE.
Labellapansa, A., Muhimmah, I., and Indrayanti (2016).
Segmentation of breast cancer cells positive 1+ and 3+
immunohistochemistry. In AIP Conference Proceed-
ings, volume 1718, page 110002. AIP Publishing.
Limsiroratana, S. and Boonyaphiphat, P. (2009). Computer-
aided system for microscopic images: application
to breast cancer nuclei counting. INTERNATIONAL
JOURNAL OF APPLIED, 2(1):69.
ICoSET 2019 - The Second International Conference on Science, Engineering and Technology
66