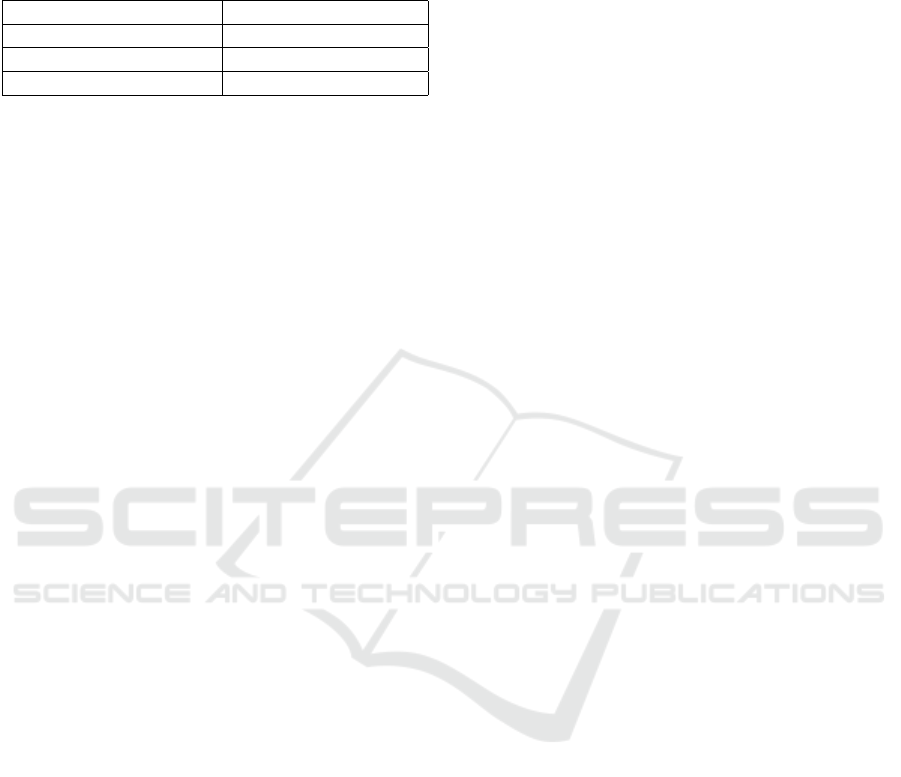
racy level by 89%. However, the KL method is able
to reduce the features to 18 which indicates that the
KL method is more efficient.
Table 3: Word of stop consonants classification result.
Feature Acc. classification (%)
WPT (25 features) 95
WPT + SVD2 95,67
WPT + KL (18 features) 89
4 CONCLUSIONS
In this paper, a dimensionality reduction method
based on SVD combined with time-frequency fea-
tures was performed for classifying the Indonesian
stop consonants in the context of CV syllable as well
as the word of stop consonants. Based on the ex-
perimental result presented in this paper, it can be
concluded that the SVD gives improved the clas-
sification scores as shown by average classification
rate of 68.7%, and 67.58 for WPSDS+SVD1 and
WRPSDS+SVD10, respectively which are 3.34% and
3.69% increase than WPSDS and WRPSDS without
dimensionality reduction by using SVD. The appli-
cation of the SVD method in the dimension of word
sound features, at a certain level of the reduction in-
dex (index-2) can increase the classification results,
however an increase in the reduction index that is
too high tends to reduce the results of the classifica-
tion. Classification of stop consonants is more diffi-
cult when compared to words of stop consonant. The
highest classification result for the words is 95.67%.
ACKNOWLEDGEMENTS
This work has been supported by Directorate Gen-
eral of Research, Technology, and Higher Educa-
tion (RISTEKDIKTI) of Indonesia under “Peneli-
tian Dosen Pemula” scheme with contract number
B/1435.28/L5/RA.00/2019.
REFERENCES
Boccaletti, S., Giaquinta, A., and Arecchi, F. (1997). Adap-
tive recognition and filtering of noise using wavelets.
Physical review E, 55(5):5393.
Chakroborty, S. and Saha, G. (2010). Feature selection us-
ing singular value decomposition and qr factorization
with column pivoting for text-independent speaker
identification. Speech Communication, 52(9):693–
709.
Fachrie, M. and Harjoko, A. (2015). Robust indonesian
digit speech recognition using elman recurrent neu-
ral network. Konferensi Nasional Informatika (KNIF),
2015:49–54.
Ferdiansyah, V. and Purwarianti, A. (2011). Indonesian
automatic speech recognition system using english-
based acoustic model. In Proceedings of the 2011 In-
ternational Conference on Electrical Engineering and
Informatics, pages 1–4. IEEE.
Fugal, D. L. (2009). Conceptual wavelets in digital signal
processing: an in-depth, practical approach for the
non-mathematician. Space & Signals Technical Pub.
Gamallo, P. and Bordag, S. (2011). Is singular value decom-
position useful for word similarity extraction? Lan-
guage resources and evaluation, 45(2):95–119.
Hariharan, M., Paulraj, M. P., and Yaacob, S. (2009). Iden-
tification of vocal fold pathology based on mel fre-
quency band energy coefficients and singular value
decomposition. In 2009 IEEE International Confer-
ence on Signal and Image Processing Applications,
pages 514–517. IEEE.
Kristomo, D., Hidayat, R., and Soesanti, I. (2018). Fea-
ture selection using singular value decomposition for
stop consonant classification. In 2018 Joint 7th Inter-
national Conference on Informatics, Electronics & Vi-
sion (ICIEV) and 2018 2nd International Conference
on Imaging, Vision & Pattern Recognition (icIVPR),
pages 432–435. IEEE.
Kristomo, D., Hidayat, R., Soesanti, I., and Kusjani, A.
(2016). Heart sound feature extraction and classifica-
tion using autoregressive power spectral density (ar-
psd) and statistics features. In AIP Conference Pro-
ceedings, volume 1755, page 090007. AIP Publishing
LLC.
Lukasik, E. (2000). Wavelet packets based features
selection for voiceless plosives classification. In
2000 IEEE International Conference on Acoustics,
Speech, and Signal Processing. Proceedings (Cat. No.
00CH37100), volume 2, pages II689–II692. IEEE.
Nafisah, S., Wahyunggoro, O., and Nugroho, L. E. (2016).
An optimum database for isolated word in speech
recognition system. Telkomnika, 14(2).
Sarac¸O
˘
gLu, R. (2012). Hidden markov model-based classi-
fication of heart valve disease with pca for dimension
reduction. Engineering Applications of Artificial In-
telligence, 25(7):1523–1528.
Theodoridis, S. and Koutroumbas, K. (2009). Pattern recog-
nition, edition.
Yazdanpanah, M., Allard, L., Durand, L., and Guardo, R.
(1999). Evaluation of karhunen-loeve expansion for
feature selection in computer-assisted classification of
bioprosthetic heart-valve status. Medical & biological
engineering & computing, 37(4):504–510.
Yessivirna, R. and Marji, D. E. R. (2013). Klasifikasi suara
berdasarkan gender (jenis kelamin) dengan metode k-
nearest neighbor (knn). Jurnal Doro, 2(3).
CONRIST 2019 - International Conferences on Information System and Technology
84