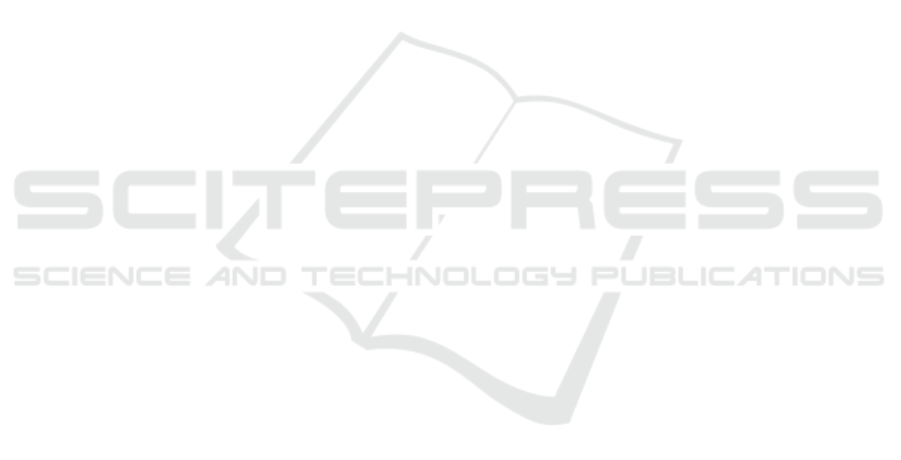
The EMG signals were obtained using Myo
Armband. Features such as root mean square were
extracted and fed to the classifier and the classifiers
were then trained, tested and validated on different
features. It was found that using root mean square as
a feature and KNN as a classifier gave maximum
accuracy. An offline accuracy of 98.75% and an
online accuracy of 90% was achieved(Anil and
Sreeletha, 2019).
However, the prosthesis can be made more
natural-like by mimicking the human gait that can be
done by increasing the number of output classes on
the basis of the angle of the ankle joint.
Moreover, the classifier performance can be
improved by increasing the number of training data
set. The combination of different features can also be
implemented to increase the accuracy. The artificial
neural networks or other deep learning techniques
may be applied to improve the accuracy.
Although, the prime objective of controlling the
prosthetic was achieved, closed loop control must be
introduced for precise and robust control of the joint.
More work needs to be done to make the
prosthesis portable by the introduction of single board
computer like Beaglebone, Raspberry Pi and UDOO.
REFERENCES
Alam, M. S. and Arefin, A. S. (2018) ‘Real-Time
Classification of Multi-Channel Forearm EMG to
Recognize Hand Movements using Effective Feature
Combination and LDA Classifier’, Bangladesh Journal
of Medical Physics, 10(1), pp. 25–39. doi:
10.3329/bjmp.v10i1.39148.
Alkan, A. and Günay, M. (2012) ‘Identification of EMG
signals using discriminant analysis and SVM
classifier’, Expert Systems with Applications. Elsevier
Ltd, 39(1), pp. 44–47. doi:
10.1016/j.eswa.2011.06.043.
Altın, C. and Er, O. (2016) ‘Comparison of Different Time
and Frequency Domain Feature Extraction Methods on
Elbow Gesture’s EMG’, European Journal of
Interdisciplinary Studies, 5(1), p. 35. doi:
10.26417/ejis.v5i1.p35-44.
Anil, N. and Sreeletha, S. H. (2019) ‘EMG Based Gesture
Recognition Using Machine Learning’, Proceedings of
the 2nd International Conference on Intelligent
Computing and Control Systems, ICICCS 2018. IEEE,
(Iciccs), pp. 1560–1564. doi:
10.1109/ICCONS.2018.8662987.
Bright, D. et al. (2016) ‘EEG-based brain controlled
prosthetic arm’, Conference on Advances in Signal
Processing, CASP 2016, pp. 479–483. doi:
10.1109/CASP.2016.7746219.
Cheesborough, J. E. et al. (2015) ‘Targeted muscle
reinnervation and advanced prosthetic arms’, Seminars
in Plastic Surgery, 29(1), pp. 62–72. doi: 10.1055/s-
0035-1544166.
Cho, E. et al. (2016) ‘Force myography to control robotic
upper extremity prostheses: A feasibility study’,
Frontiers in Bioengineering and Biotechnology,
4(MAR), pp. 1–12. doi: 10.3389/fbioe.2016.00018.
Christov, I., Raikova, R. and Angelova, S. (2018)
‘Separation of electrocardiographic from
electromyographic signals using dynamic filtration’,
Medical Engineering and Physics. Elsevier Ltd, 57, pp.
1–10. doi: 10.1016/j.medengphy.2018.04.007.
González, D. S. and Castellini, C. (2013) ‘A realistic
implementation of ultrasound imaging as a human-
machine interface for upper-limb amputees’, Frontiers
in Neurorobotics, 7(OCT), pp. 1–11. doi:
10.3389/fnbot.2013.00017.
Hong, K. S., Khan, M. J. and Hong, M. J. (2018) ‘Feature
Extraction and Classification Methods for Hybrid
fNIRS-EEG Brain-Computer Interfaces’, Frontiers in
Human Neuroscience, 12(June), pp. 1–25. doi:
10.3389/fnhum.2018.00246.
Khan, R. A. et al. (2018) ‘FNIRS-based Neurorobotic
Interface for gait rehabilitation’, Journal of
NeuroEngineering and Rehabilitation. Journal of
NeuroEngineering and Rehabilitation, 15(1), pp. 1–17.
doi: 10.1186/s12984-018-0346-2.
Maat, B. et al. (2018) ‘Passive prosthetic hands and tools:
A literature review’, Prosthetics and Orthotics
International, 42(1), pp. 66–74. doi:
10.1177/0309364617691622.
Naseer, N. et al. (2016) ‘Determining optimal feature-
combination for LDA classification of functional near-
infrared spectroscopy signals in brain-computer
interface application’, Frontiers in Human
Neuroscience, 10(MAY2016), pp. 1–10. doi:
10.3389/fnhum.2016.00237.
Pasquina, P. F. et al. (2015) ‘First-in-man demonstration of
a fully implanted myoelectric sensors system to control
an advanced electromechanical prosthetic hand’,
Journal of Neuroscience Methods. Elsevier B.V., 244,
pp. 85–93. doi: 10.1016/j.jneumeth.2014.07.016.
Phinyomark, A., Khushaba, R. N. and Scheme, E. (2018)
‘Feature extraction and selection for myoelectric
control based on wearable EMG sensors’, Sensors
(Switzerland), 18(5), pp. 1–17. doi:
10.3390/s18051615.
Qureshi, N. K. et al. (2016) ‘Comparison of classification
performance for fNIRS-BCI system’, 2016 2nd
International Conference on Robotics and Artificial
Intelligence, ICRAI 2016, pp. 54–57. doi:
10.1109/ICRAI.2016.7791228.
Reaz, M. B. I., Hussain, M. S. and Mohd-Yasin, F. (2006)
‘Techniques of EMG signal analysis: Detection,
processing, classification and applications’, Biological
Procedures Online, 8(1), pp. 11–35. doi:
10.1251/bpo115.
Simbolon, A. I. et al. (2016) ‘An experiment of lie detection
based EEG-P300 classified by SVM algorithm’,
Proceedings of the 2015 International Conference on
Automation, Cognitive Science, Optics, Micro Electro-
ICHIMAT 2019 - International Conference on Health Informatics and Medical Application Technology
80