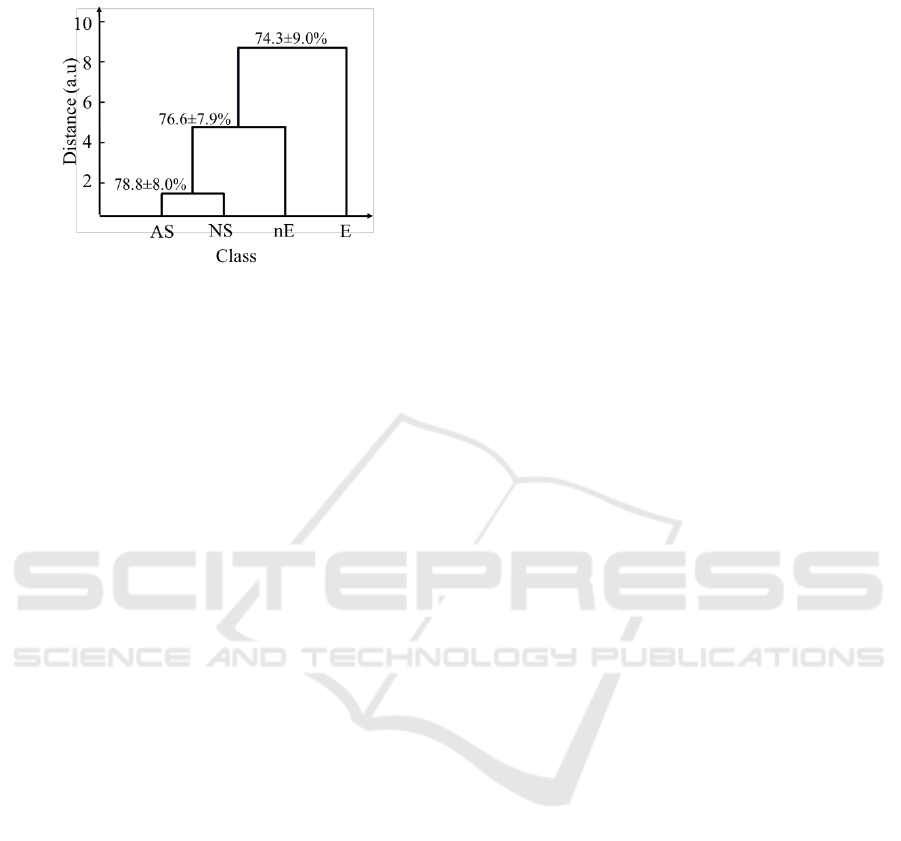
online scheme for decoding what humans hear upon
a set of training data.
Figure 13: Hierarchical clustering: Dendogram for 4-class
problem. The height (y-xis) indicates the distance
separating the feature space of the two super-categories; the
percentage numbers show the accuracy between those
super-categories.
4 CONCLUSIONS
This paper demonstrated the feasibility of our
proposed online method and discussed its
potentialities for processing in decoding brain
activity. This study used fNIRS signals evoked by
audio-stimuli from multiple sound categories. To
account for data processing in our online scheme, the
AR-IRLS algorithm as pre-processing, feature-
selection, and classifier performance were discussed.
Interestingly, the performance of online classification
was higher than the chance levels in almost subjects.
Finally, the authors conclude that the fNIRS signals
evoked by audio-stimuli from multiple sound
categories can be effectively utilized in an online
decoding scheme.
ACKNOWLEDGEMENTS
This research was supported by the University of
Pittsburgh Department of Radiology, USA and
Department of Electical Engineering, Universitas
Padjajaran Indonesia.
REFERENCES
Barker, J. W., et al., Autoregressive model based algorithm
for correcting motion and serially correlated errors in
fNIRS, Biomedical Optic Express 4, 1366-1379, 2013.
Barker, J. W., et al., Correction of motion artifacts and
serial correlations for real-time functional near-infrared
spectroscopy Neurophotonics 3, 031410, 2016.
Cope, M. et al., Methods of quantitating cerebral near
infrared spectroscopy data, Adv. Exp. Med. Biol. 222,
183-189, 1988.
Hong, K.-S. and Santosa, H., Decoding four different
sound-categories in the auditory cortex using functional
near-infrared spectroscopy, Hearing Research 333,
157-166, 2016.
Huppert, T. J. et al., A temporal comparison of BOLD,
ASL, and NIRS hemodynamic responses to motor
stimuli in adult humans, Neuroimage 29(2), 368-382
(2006).
Lawler, C. A., et al., The use of functional near-infrared
spectroscopy for measuring cortical reorganization in
cochlear implant users: A possible predictor of variable
speech outcomes? Cochlear Implantts Int. 16, S30-S32,
2015.
Pollonini, L., et al., Auditory cortex activation to natural
speech and simulated cochlear implant speech
measured with functional near-infrared spectroscopy,
Hearing Research 309, 84-93, 2014.
Plichta, M. M., et al., Auditory cortex activation is
modulated by emotion: A functional near-infrared
spectroscopy (fNIRS) study, Neuroimage 55, 1200-
1207, 2011.
Santosa, H., Fishburn, F., Zhai, X., Huppert, T. J.,
Investigation of sensitivity-specificity of canonical- and
deconvolution-based linear models in evoked
functional near-infrared spectroscopy, Neurophotonics
6(2), 025009 (2019).
Santosa, H., et al., Lateralization of music processing
auditory cortex: An fNIRS study, Frontier Behavioural
Neuroscience 8, 00418, 2014.
Santosa, H., et al., The NITS brain AnalyzIR toolbox,
Algorithms 11(5), 73, 2018.
Simbolon, A. I. et al. (2016) ‘An experiment of lie detection
based EEG-P300 classified by SVM algorithm’,
Proceedings of the 2015 International Conference on
Automation, Cognitive Science, Optics, Micro Electro-
Mechanical System, and Information Technology,
ICACOMIT 2015, pp. 68–71. doi:
10.1109/ICACOMIT.2015.7440177.
Turnip, A. et al. (2016) ‘EEG-based brain-controlled
wheelchair with four different stimuli frequencies’,
Internetworking Indonesia Journal, 8(1), pp. 65–69.
Turnip, A. and Simbolon, A. I., “Online Brain Activity
Extraction from EEG-P300 Signals with Nonlinear
Autoregressive Model” Internetworking Indonesian
Journal, vol. 8, no. 1, 2016.
ICHIMAT 2019 - International Conference on Health Informatics and Medical Application Technology
340