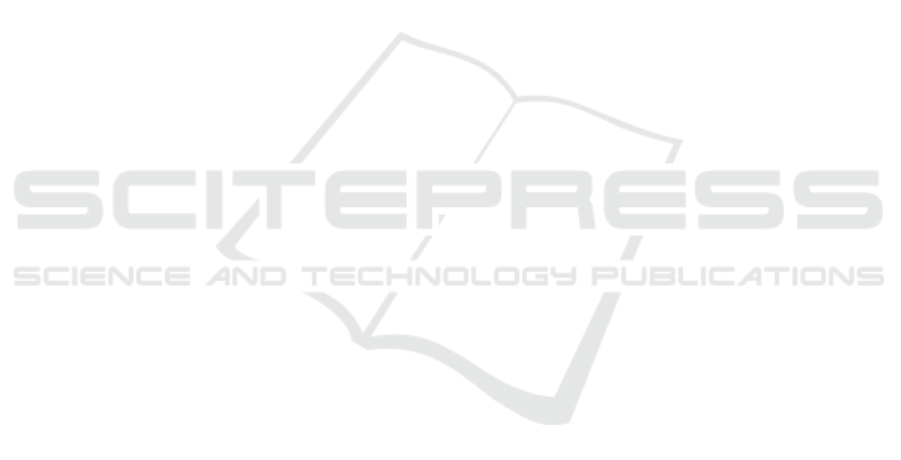
cause of the many factors that support customers to
be loyal to the e-commerce industry. Customers and
companies can enjoy the benefits of a loyalty program
(Magatef and Tomalieh, 2015) and customer loyalty
is categorized into four types of categories, namely
no loyalty, loyalty, latent loyalty, and spurious loy-
alty, this category is seen based on two dimensions,
namely attitude and preferential treatment (Dick and
Basu, 1994), A typical approach uses Platinum, Gold,
and Silver tiers, typically based on purchase volumes.
The collaborated Na
¨
ıve Bayes as a data mining tech-
nique and RFM as analytical, and positioning in this
research between e-commerce business actors in busi-
ness competition is essential as a basis for taking ap-
propriate decision making for stakeholders. Based on
their duties, mining data is grouped into description,
estimation, prediction, classification, clustering, asso-
ciation (Larose, 2015), meanwhile, data mining is the
process of discovering interesting patterns and knowl-
edge from large amounts of data (Han et al., 2012).
This study uses the supervised learning for classifica-
tion method by using the Na
¨
ıve classifier statistically
(Bhosale and Ade, 2014). Another explanation about
the Na
¨
ıve Bayes classifier in determining the classifi-
cation of the right test data is finding the highest prob-
ability (Feldman et al., 2007). Then RFM as a clas-
sification analysis method, and to increase company
profits in the short term RFM can be used in predict-
ing responses (Baecke and Van den Poel, 2011). In di-
rect marketing and long history, practically the RFM
model is widely applied (Wei et al., 2010). Indeed
the results of data mining by combining customer de-
mographic variables can be done, but it is better to
use RFM analysis because it can improve customer
relations better (Nimbalkar and Shah, 2013). So the
objective in this research to experiment with the al-
gorithm of Na
¨
ıve Bayes classifier and RFM then use
customer loyalty data as decision making for stake-
holders.
2 PREVIOUS RESEARCH
Some of the previous research was explained in this
section to support the research conducted, for the first
research (Da et al., 2011) In this study based on the
quantitative combined value of the RFM model and
the Na
¨
ıve Bayesian algorithm that functions for the
classification of students and offers more decisions
and methods as feedback for learning resources by
presenting new construction methods. Second re-
search (Zu et al., 2012) to improve the quality of
the Na
¨
ıve Bayes classification method, it is neces-
sary to change the attribute group with the new at-
tribute group in presenting the extended Bayes model.
Third research (Nimbalkar and Shah, 2013) In RFM
analysis to manage customer relations in segmenta-
tion, a grouping of values is needed and is classified
into each customer segment that has been grouped.
Then the fourth research (Cheng and Chen, 2009)
Other studies use rough sets or LEM2 algorithms for
extracting classifications in CRM, and the resulting
RFM model becomes quantitative values as its at-
tributes and other K-means algorithms in grouping
customer values. Based on previous research that has
been explained, the differentiator in this study uses
Naive Bayes as a classification method in detail to the
attributes that are tested and the customer is classi-
fied by the RFM method and in previous studies that
have been conducted by other researchers are still lit-
tle discussing the combining of these two methods
between Naive Bayes and RFM then positioning in
this research between e-commerce business actors in
business competition is very important as a basis for
taking appropriate decision making for stakeholders.
3 MATERIAL AND METHOD
3.1 Research Stages
The knowledge discovery process is shown in as an
iterative sequence of the following steps (Han et al.,
2012):
1. Data Cleaning: Data cleaning is a process of dis-
appearing noise and inconsistent data or irrelevant
data
2. Data Integration: Data integration is the merg-
ing of data from various databases into one new
database.
3. Data Selection: Data that is in the database is of-
ten not all used, therefore only data suitable for
analysis to be taken from the database.
4. Data Transformation: Data is converted or com-
bined into a format suitable for processing in Data
Mining
5. Data Mining: It is a major process when methods
are applied to find knowledge valuable and hidden
from data. Several methods can be used based on
Data Mining grouping
6. Pattern Evaluation: To identify interesting pat-
terns into knowledge based found.
7. Knowledge Presentation: Is a visualization and
presentation of knowledge about the methods
used to obtain knowledge obtained by users.
ICCETIM 2019 - International Conference on Creative Economics, Tourism Information Management
148