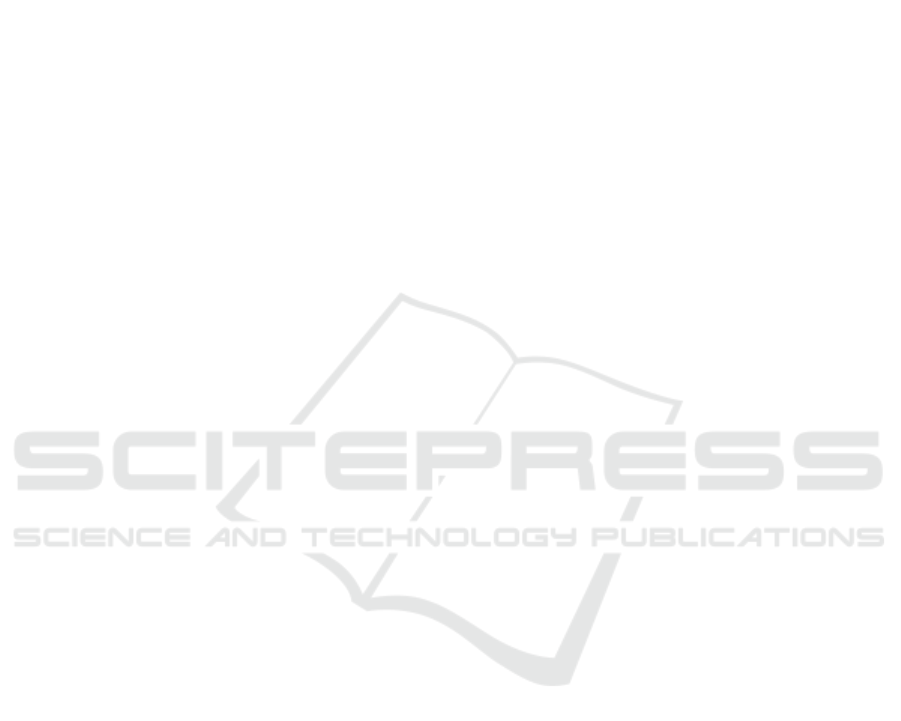
Prediction and Analysis of the Factors That Influence Volume of Air
Passenger at Banyuwangi Airport using Econometric
Suwander Husada
1
, Endroyono
1
and Yoyon K. Suprapto
1
1
Department of Electrical Engineering, Institute of Institute Teknologi Sepuluh Nopember, Surabaya, Indonesia
Keywords:
Econometric, Passenger Prediction, Airport Masterplan.
Abstract:
The volume of passengers at the airport in the future needs to be predicted. From several air passenger
prediction methods, one of them is the econometric method. This method has the excellence of long-term
predictions and gaining knowledge of economic factors that affect the volume of air passengers. In this study,
several economic factors, such as hotel occupancy, population, rupiah exchange rate, tourism visitor, inflation,
and the Banyuwangi Consumer Price Index (CPI), were analyzed. Pre-analysis data needed before building
the model, including data selection, data description, data pattern recognition, and data completeness. The best
econometric model is obtained from the results of the combination test of each variable. The best econometric
model is used to predict the volume of air passengers in Banyuwangi airport for the next 20 years. From the
results, three factors significantly effect the volume of air passengers, that is, hotel occupancy affect 0.301%,
population affect 0.132%, and the rupiah exchange rate affect -0.481%. To evaluate the accuracy of predictions
using the Mean Absolute Percentage Error (MAPE) with a value of 15,208 %.
1 INTRODUCTION
Indonesia Civil Avition Law No.1 2009, it is stated
that the airport, one of the transportation network
nodes. It encourages and supports industrial and
trade activities. It encourages and supports indus-
trial and trade activities. The economic stability of
Banyuwangi Regency has an impact on the growth of
human and goods mobilization from origin to desti-
nation through air transportation modes. Referring to
the Banyuwangi Airport Master Plan, phase II is es-
timated to serve aircraft passengers around 272,500
passengers per year, but in 2017 volume of air pas-
sengers at Banyuwangi Airport is 188,949 thousand,
and by the end of 2018 air passengers volume is
366,155 people. There is a significant difference be-
tween the master plan projections and existing pas-
senger. Banyuwangi Airport has the potential to ex-
perience density and result in a decrease in the quality
of services for users of air transportation services. In
this condition, there is a high need to evaluate and
projections air volume passengers in the future.
The purpose of this study is to predict the vol-
ume of air passengers for the next 20 years and an-
alyze the factors that effect the volume of passengers
at Banyuwangi Airport. According result of analysis,
it was obtaining knowledge about the factors that in-
fluence the volume of air passengers at Banyuwangi
Airport. The study result can be used as a reference
for policy development.
2 LITERATURE REVIEW
Economic factors affect the volume of passengers at
certain airports, regions or cities. The most com-
mon economic indicators are Gross Domestic Product
(GDP), Per capita GDP, Population (Pop), Income,
and Per Capita Income (Guo and Zhong, 2017). There
are many studies that discuss the relationship between
air passenger volume and economic variables.
Husada, S., Endroyono, . and Suprapto, Y.
Prediction and Analysis of the Factors That Influence Volume of Air Passenger at Banyuwangi Airport using Econometric.
DOI: 10.5220/0009880301730179
In Proceedings of the 2nd International Conference on Applied Science, Engineering and Social Sciences (ICASESS 2019), pages 173-179
ISBN: 978-989-758-452-7
Copyright
c
2020 by SCITEPRESS – Science and Technology Publications, Lda. All rights reserved
173