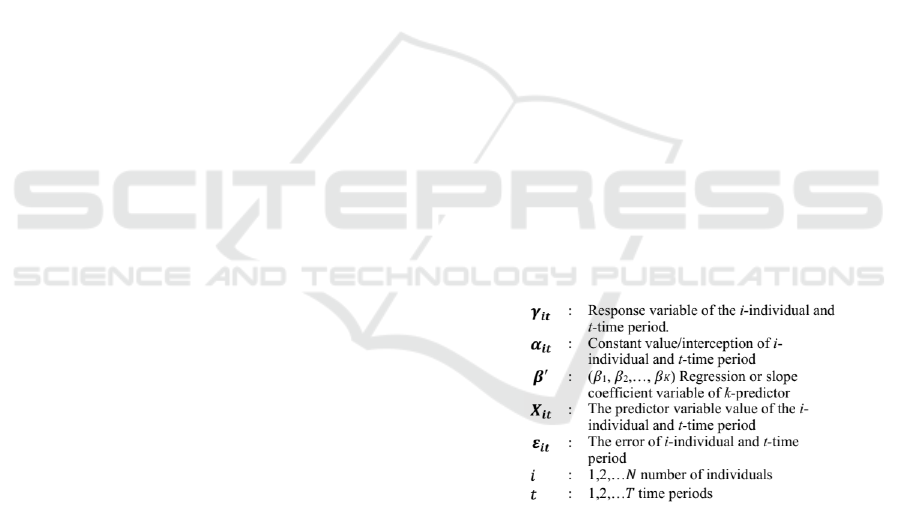
years is included in the high category (CFR ¿ 1%)
(Kemenkes, 2017).
The spread of DHF in Indonesia is influenced by
many factors such as climate, community behaviour,
environment, as well as demographic and socioeco-
nomic conditions (Arsin, 2013). The climate factors
include temperature, rainfall, rainy days, humidity,
and wind speed; behavioural factor is basically a per-
son’s response towards stimuli that is related to illness
and disease including knowledge, actions, and beliefs
associated with DHF, availability of health resources,
adequate and affordability of health facilities, com-
munity support, as well as the concern from govern-
ment and health workers; environmental factors are
the number of places or containers of DHF vector pro-
liferation; demographic factors consist of the popula-
tion density and population mobility; socio-economic
factors are the level of education, employment, and
the number of family members. Of the factors men-
tioned above, knowing which of the factors that have
a significant effect on DHF is very important so that
future efforts to prevent and control DHF can be done
more effectively and efficiently.
Modelling is a method that can be used to de-
termine the factors that have a significant effect on
DHF in Pasuruan Regency. This study aimed to find
a model that is able to explain the influencing fac-
tors of DHF in 21 sub-districts of Pasuruan within the
period of 2015 to 2018. Different regional character-
istics in each sub-district are thought to influence the
incidence of DHF. By that, this study used Panel Data
structure. There has never been a research which ex-
amined the factors of DHF in Pasuruan Regency so
that the results of this modelling are expected to be
used as early warnings or basis for the formulation of
strategic policies to prevent and eradicate DHF in the
future.
Several studies related to the analysis of DHF have
been done before. Research conducted by (Ariani,
2018) used the Negative Binomial regression model
to produce variables that significantly influence DHF,
namely population density, number of health workers
and rainfall. Subsequent research by (Rustiani, 2017)
using multiple linear regression resulted in R
2
values
of 67 percent, significant variables included popula-
tion density, rainfall and larva free index. Research
conducted by (Rasmanto, 2016) using Linear Regres-
sion resulted in R
2
values of 24.1 percent, variables
that had a significant effect consisted of air temper-
ature and relative humidity. The study by (Hasirun,
2016) used a Spatial regression model with spatial
error resulting in R
2
values of 43.34 percent, sig-
nificant variables covering rainfall, health facilities,
the percentage of PHBS houses, and the percentage
of healthy houses. Subsequent research by (Martha,
2015) used panel data regression with a fixed effect
model approach resulted in R
2
values of 72,76 per-
cent, significant variables included population den-
sity, population mobility, the average age of DHF pa-
tients, and the number of DHF patients in the previous
time period. Based on these studies we use several
variables that have been used previously namely pop-
ulation density, rainfall, rainy days, temperature, and
the number of health facilities. Then we add two new
variables namely the waste volume and the Number
of High-Educated Population.
2 LITERATURE REVIEW
2.1 Panel Data
Panel Data is a collection of data consisting of cross-
section data and time series data. Time series data
usually includes one individual observed in a certain
period of time while cross-section data is obtained
from several individuals observed in a certain period
of time. Therefore, it can be said that the Panel Data
presents larger and more informative data and gener-
ates a greater degree of freedom. The general form of
Panel Data regression models can be seen in Equation
1 (Hsiao, 2003; I. et al., 2017).
γ
it
= α
it
+ β
0
X
it
+ ε
it
(1)
2.2 Estimation of Panel Data
Regression Model
In making parameter estimation, the model depends
on the assumptions about intercepts and slope coef-
ficients. Using Panel Data allows different intercepts
and slope coefficients to occur in each individual and
each time period. In this concept, there are three ap-
proaches that can be used, namely CEM (Common
Effect Models), FEM (Fixed Effect Model) and REM
(Random Effect Models) (Widarjono, 2013; I. et al.,
2017)
Data Panel Modelling with Fixed Effect Model (FEM) Approach to Analyze the Influencing Factors of DHF in Pasuruan Regency
225