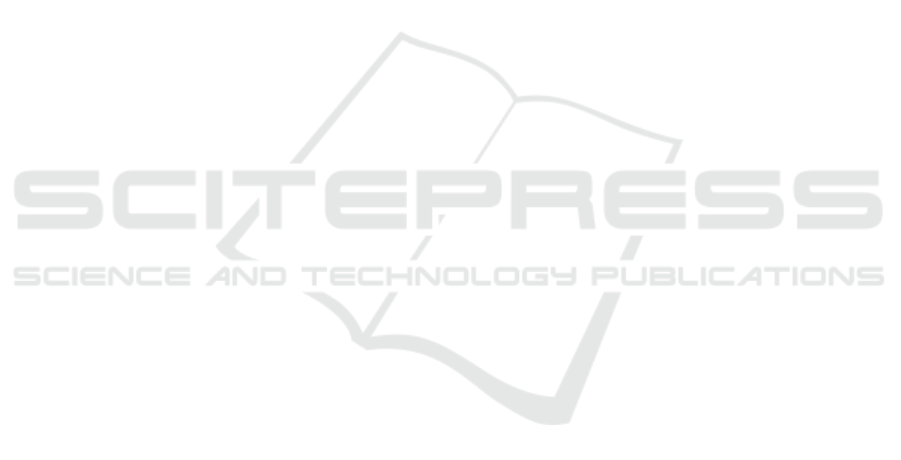
REFERENCES
Belsis, P., Chalaris, I., Chalaris, M., Skourlas, C., and Tso-
lakidis, A. (2014). The analysis of the length of stud-
ies in higher education based on clustering and the
extraction of association rules. Procedia-Social and
Behavioral Sciences, 147:567–575.
Berland, M., Baker, R. S., and Blikstein, P. (2014). Edu-
cational data mining and learning analytics: Applica-
tions to constructionist research. Technology, Knowl-
edge and Learning, 19(1-2):205–220.
Bhatia, P. (2019). Data Mining and Data Warehousing:
Principles and Practical Techniques. Cambridge Uni-
versity Press.
Campagni, R., Merlini, D., Sprugnoli, R., and Verri, M. C.
(2015). Data mining models for student careers. Ex-
pert Systems with Applications, 42(13):5508–5521.
Chalaris, M., Gritzalis, S., Maragoudakis, M.,
Sgouropoulou, C., and Tsolakidis, A. (2014).
Improving quality of educational processes provid-
ing new knowledge using data mining techniques.
Procedia-Social and Behavioral Sciences, 147:390–
397.
Dutt, A., Aghabozrgi, S., Ismail, M. A. B., and Mahroeian,
H. (2015). Clustering algorithms applied in educa-
tional data mining. International Journal of Informa-
tion and Electronics Engineering, 5(2):112.
Farid, D. M., Zhang, L., Rahman, C. M., Hossain, M. A.,
and Strachan, R. (2014). Hybrid decision tree and
na
¨
ıve bayes classifiers for multi-class classification
tasks. Expert systems with applications, 41(4):1937–
1946.
Kaur, P., Singh, M., and Josan, G. S. (2015). Classification
and prediction based data mining algorithms to predict
slow learners in education sector. Procedia Computer
Science, 57:500–508.
Lefebvre, W., Vurpillot, F., and Sauvage, X. (2016). Atom
Probe Tomography: Put Theory into Practice. Aca-
demic Press.
Manjarres, A. V., Sandoval, L. G. M., and Su
´
arez, M. S.
(2018). Data mining techniques applied in educational
environments: Literature review. Digital Education
Review, (33):235–266.
Mayilvaganan, M. and Kalpanadevi, D. (2015). Cognitive
skill analysis for students through problem solving
based on data mining techniques. Procedia Computer
Science, 47:62–75.
Mishra, T., Kumar, D., and Gupta, S. (2014). Mining
students’ data for prediction performance. In 2014
Fourth International Conference on Advanced Com-
puting & Communication Technologies, pages 255–
262. IEEE.
Patarapichayatham, C., Kamata, A., and Kanjanawasee,
S. (2012). Evaluation of model selection strategies
for cross-level two-way differential item functioning
analysis. Educational and Psychological Measure-
ment, 72(1):44–51.
Pradeep, A., Das, S., and Kizhekkethottam, J. J. (2015).
Students dropout factor prediction using edm tech-
niques. In 2015 International Conference on Soft-
Computing and Networks Security (ICSNS), pages 1–
7. IEEE.
Renaningtias, N., Suseno, J. E., and Gernowo, R. Hybrid
decision tree and na
¨
ıve bayes classifier for predict-
ing study period and predicate of student’s gradua-
tion. International Journal of Computer Applications,
975:8887.
Shahiri, A. M., Husain, W., et al. (2015). A review on pre-
dicting student’s performance using data mining tech-
niques. Procedia Computer Science, 72:414–422.
Shukor, N., Tasir, Z., and van der Meijden, H. (2015).
An examination of online learning effectiveness using
data mining.
Sowmya, R. and Suneetha, K. (2017). Data mining with
big data. In 2017 11th International Conference on
Intelligent Systems and Control (ISCO), pages 246–
250. IEEE.
Sundar, P. P. (2013). A comparative study for predicting stu-
dents academic performance using bayesian network
classifiers. IOSR Journal of Engineering (IOSRJEN)
e-ISSN, pages 2250–3021.
Thai-Nghe, N., Drumond, L., Krohn-Grimberghe, A., and
Schmidt-Thieme, L. (2010). Recommender system
for predicting student performance. Procedia Com-
puter Science, 1(2):2811–2819.
Educational Data Mining in Graduation Rate and Grade Predictions Utilizing Hybrid Decision Tree and Naïve Bayes Classifier
157