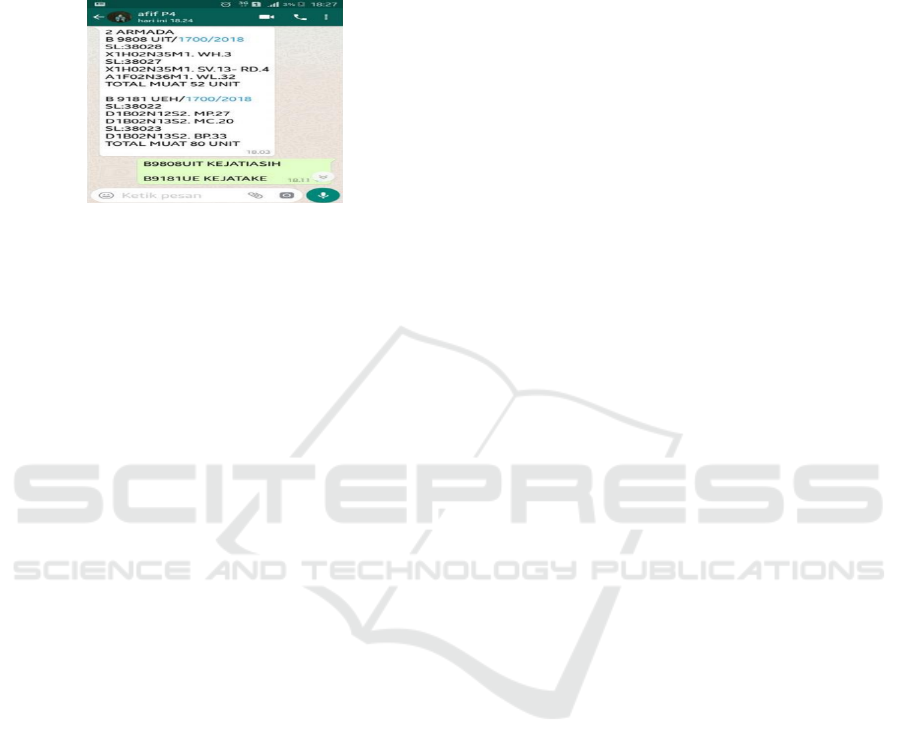
speed of the process of distributing goods. This will
ultimately have an impact on the company’s business
processes, so companies need a system that can sup-
port the process of analysing the allocation of ware-
house destinations quickly and precisely.
Figure 3: Allocation process using WhatsApp
This is where the role of decision support sys-
tems is expected to help the organization. With the
adoption of a decision support system, the data analy-
sis process to determine the destination warehouse is
scheduled to run more quickly and systematically and
can reduce the burden of analysis on analyst distribu-
tion. With this author raised the idea to Analysis and
Develop Information Systems Supporting Warehouse
Allocation Decisions using AHP (Mu and Pereyra-
Rojas, 2016)(Saaty, 2008). AHP methods related to
DSS have been widely used and proven to provide
benefits for companies (Susanto et al., 2017).
2 LITERATURE REVIEW
2.1 Decision Support System
Decision Support System (DSS) is a computer-based
decision support tool to assist decision-makers by pre-
senting information and interpretations for various al-
ternative decisions (Pal and Palmer, 2000). The aspect
of the scope system in DSS is automation which helps
decision-makers with different levels of intelligence.
From a theoretical point of view, decisions relate to
cognitive concepts, especially those related to ideas
that support humans in decision making. SPK can
help decision-makers in building strategic decisions.
The use of DSS has shown satisfactory results, by
minimising costs, accelerating the decision-making
process, and significant achievements in competitive
advantage.
2.2 Analytic Hierarchy Process
The Analytic Hierarchy Process (AHP) developed by
Professor Thomas Saaty in 1980 made it possible to
arrange decisions hierarchically (to reduce their com-
plexity) and show the relationship between goals (or
criteria) and potential alternatives (Mu and Pereyra-
Rojas, 2016), the stages carried out refer to the ana-
lytic hierarchy process:
1. Develop a model for decisions: Break the decision
into a hierarchy of goals, criteria, and alternatives
2. Lower the priority (weight) for the criteria
3. Consistency
4. Lower local priorities (preferences) for alterna-
tives
5. Decrease Overall Priority (Synthesis Model)
6. Do a sensitivity analysis
7. Make Final Decisions.
2.3 Previous Research
The Yager Fuzzy MADM model chosen as the Yager
analysis model to determine the most prospective cus-
tomers with better feasibility through the highest giv-
ing(Susanto et al., 2017). By using the vector D value
that has been calculated from the sum of each cri-
terion value {0.904; 0.794; 0.914; 0.794}, the most
prospective customers are customers C (D3) with a
value of 0.914.
The customer is chosen as the most prospective
customer to be given Murabahah financing. Each cri-
terion is given a weight value according to the desired
priority. The system that was built streamlined the
decision-making process time to be more efficient.
DSS generates valuation calculations based on sub-
jective and objective assessment criteria for using 5C:
Character, Capacity, Capital, Guarantee and Condi-
tions. The system can present an assessment based on
the calculation of the value of each criterion such as
very good with a value of 1, 0.8, 0.6, 0.4 and 0.2 to
improve the weight vector results obtained from the
input value of the comparison criteria to produce the
most recommended customer data sequence. The suc-
cess of AHP on DSS was also carried out by other
researchers with different achievements according to
their individual needs (Dweiri et al., 2016) (Sari et al.,
2017)(Narabin and Boonjing, 2016).
Another researcher is by (Sinesi et al., 2017) gen-
erating DSS with A multivariate logic to monitor in
real-time for one week using GPS data from Marine-
Trafficwebsite and validating the proposed model
by comparing the results of the model with real
data which counts four performance indicators (ME,
MAD, MAPE, MSE), the accuracy results are better
than the proposed model in evaluating the probability
of a maritime company choice.
Decision Support System of Warehouse Allocation using Analytical Hierarchy Process Method
295