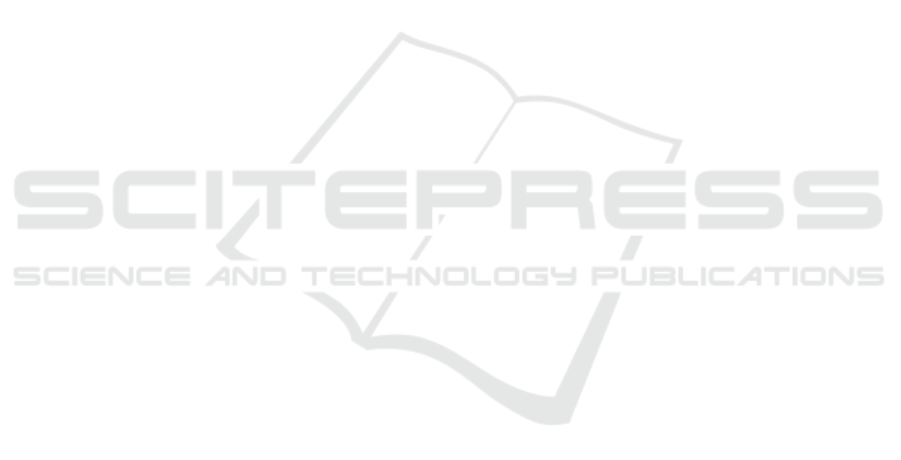
Krizhevsky, A., Sutskever, I., and Hinton, G. E. (2012). Im-
agenet classification with deep convolutional neural
networks. In Advances in neural information process-
ing systems, pages 1097–1105.
Li, Y., Qi, H., Dai, J., Ji, X., and Wei, Y. (2017). Fully
convolutional instance-aware semantic segmentation.
In Proceedings of the IEEE Conference on Computer
Vision and Pattern Recognition, pages 2359–2367.
Lin, D., Shen, D., Shen, S., Ji, Y., Lischinski, D., Cohen-
Or, D., and Huang, H. (2019). Zigzagnet: Fusing top-
down and bottom-up context for object segmentation.
In Proceedings of the IEEE Conference on Computer
Vision and Pattern Recognition, pages 7490–7499.
Lin, G., Milan, A., Shen, C., and Reid, I. (2017a). Re-
finenet: Multi-path refinement networks for high-
resolution semantic segmentation. In Proceedings of
the IEEE conference on computer vision and pattern
recognition, pages 1925–1934.
Lin, T.-Y., Dollár, P., Girshick, R., He, K., Hariharan, B.,
and Belongie, S. (2017b). Feature pyramid networks
for object detection. In Proceedings of the IEEE Con-
ference on Computer Vision and Pattern Recognition,
pages 2117–2125.
Lin, T.-Y., Maire, M., Belongie, S., Hays, J., Perona, P.,
Ramanan, D., Dollár, P., and Zitnick, C. L. (2014).
Microsoft coco: Common objects in context. In Euro-
pean conference on computer vision, pages 740–755.
Springer.
Liu, S., Qi, L., Qin, H., Shi, J., and Jia, J. (2018). Path ag-
gregation network for instance segmentation. In Pro-
ceedings of the IEEE Conference on Computer Vision
and Pattern Recognition, pages 8759–8768.
Liu, Y., Wang, Y., Wang, S., Liang, T., Zhao, Q., Tang, Z.,
and Ling, H. (2019). Cbnet: A novel composite back-
bone network architecture for object detection. arXiv
preprint arXiv:1909.03625.
Long, J., Shelhamer, E., and Darrell, T. (2015). Fully con-
volutional networks for semantic segmentation. In
Proceedings of the IEEE conference on computer vi-
sion and pattern recognition, pages 3431–3440.
Malamas, E. N., Petrakis, E. G., Zervakis, M., Petit, L., and
Legat, J.-D. (2003). A survey on industrial vision sys-
tems, applications and tools. Image and Vision Com-
puting, 21(2):171 – 188.
Massari, S. and Ruberti, M. (2013). Rare earth elements as
critical raw materials: Focus on international markets
and future strategies. Resources Policy, 38(1):36–43.
Otsu, N. (1979). A threshold selection method from gray-
level histograms. IEEE transactions on systems, man,
and cybernetics, 9(1):62–66.
Pinheiro, P. O., Lin, T.-Y., Collobert, R., and Dollár, P.
(2016). Learning to refine object segments. In Eu-
ropean Conference on Computer Vision, pages 75–91.
Springer.
Pomares, J., Puente, S., Torres, F., Candelas, F., and Gil,
P. (2004). Virtual disassembly of products based on
geometric models. Computers in Industry, 55(1):1–
14.
Ren, S., He, K., Girshick, R., and Sun, J. (2015). Faster
r-cnn: Towards real-time object detection with region
proposal networks. In Advances in neural information
processing systems, pages 91–99.
Ronneberger, O., Fischer, P., and Brox, T. (2015). U-net:
Convolutional networks for biomedical image seg-
mentation. In International Conference on Medical
image computing and computer-assisted intervention,
pages 234–241. Springer.
Rusu, R. B., Marton, Z. C., Blodow, N., Dolha, M., and
Beetz, M. (2008). Towards 3D Point Cloud Based
Object Maps for Household Environments. Robotics
and Autonomous Systems Journal (Special Issue on
Semantic Knowledge in Robotics), 56(11):927–941.
Sandler, M., Howard, A., Zhu, M., Zhmoginov, A., and
Chen, L.-C. (2018). Mobilenetv2: Inverted residu-
als and linear bottlenecks. In Proceedings of the IEEE
conference on computer vision and pattern recogni-
tion, pages 4510–4520.
Szegedy, C., Vanhoucke, V., Ioffe, S., Shlens, J., and Wo-
jna, Z. (2016). Rethinking the inception architecture
for computer vision. In Proceedings of the IEEE con-
ference on computer vision and pattern recognition,
pages 2818–2826.
Tabuchi, H. (2010). Japan recycles minerals from used elec-
tronics. New York Times, 4.
Tonko, M. and Nagel, H.-H. (2000). Model-based stereo-
tracking of non-polyhedral objects for automatic dis-
assembly experiments. International Journal of Com-
puter Vision, 37(1):99–118.
Torres, F., Puente, S., and Aracil, R. (2003). Disassembly
planning based on precedence relations among assem-
blies. International Journal of Advanced Manufactur-
ing Technology, 21.
Ukida, H. (2007). Visual defect inspection of rotating screw
heads. In SICE Annual Conference 2007, pages 1478–
1483. IEEE.
Wegener, K., Chen, W. H., Dietrich, F., Dröder, K., and
Kara, S. (2015). Robot assisted disassembly for the
recycling of electric vehicle batteries. Procedia CIRP,
29:716–721.
Weigl-Seitz, A., Hohm, K., Seitz, M., and Tolle, H. (2006).
On strategies and solutions for automated disassem-
bly of electronic devices. International Journal of Ad-
vanced Manufacturing Technology, 30(5-6):561–573.
Xie, S., Girshick, R., Dollár, P., Tu, Z., and He, K. (2017).
Aggregated residual transformations for deep neural
networks. In Proceedings of the IEEE conference on
computer vision and pattern recognition, pages 1492–
1500.
Xie, S. Q., Cheng, D., Wong, S., and Haemmerle, E. (2008).
Three-dimensional object recognition system for en-
hancing the intelligence of a KUKA robot. Interna-
tional Journal of Advanced Manufacturing Technol-
ogy, 38(7-8):822–839.
Yildiz, E. and Wörgötter, F. (2019). DCNN-Based
Screw Detection for Automated Disassembly Pro-
cesses. Proceedings of the 15th International Con-
ference on Signal Image Technology & Internet based
Systems.
Zhang, M., Zhou, Y., Zhao, J., Man, Y., Liu, B., and Yao, R.
(2019). A survey of semi- and weakly supervised se-
mantic segmentation of images. Artificial Intelligence
Review.
A Visual Intelligence Scheme for Hard Drive Disassembly in Automated Recycling Routines
27