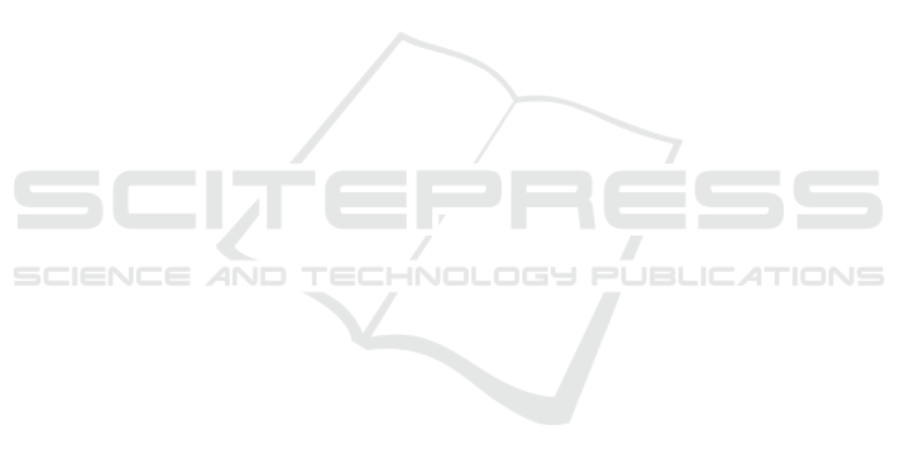
itemset and ensemble learning for SMS classification.
Expert Systems with Applications, 42(3):1065–1073.
Ahmed, I., Guan, D., and Chung, T. C. (2014). SMS clas-
sification based on na
¨
ıve bayes classifier and apriori
algorithm frequent itemset. International Journal of
Machine Learning and Computing, 4(2):183.
Almeida, T. A., Hidalgo, J. M. G., and Silva, T. P. (2013).
Towards SMS spam filtering: Results under a new
dataset. International Journal of Information Security
Science, 2(1):1–18.
Almeida, T. A., Hidalgo, J. M. G., and Yamakami, A.
(2011). Contributions to the study of SMS spam filter-
ing: New collection and results. In ACM Symposium
on Document Engineering, pages 259–262.
Chawla, N. V., Bowyer, K. W., Hall, L. O., and Kegelmeyer,
W. P. (2002). SMOTE: synthetic minority over-
sampling technique. Journal of Artificial Intelligence
Research, 16:321–357.
Chinchor, N. (1992). MUC-4 evaluation metrics. In 4th
Conference on Message Understanding (MUC), pages
22–29.
Choudhary, N. and Jain, A. K. (2017). Towards filtering
of SMS spam messages using machine learning based
technique. In International Conference on Advanced
Informatics for Computing Research (ICAICR), pages
18–30.
Cormack, G. V., Hidalgo, J. M. G., and Sanz, E. P. (2007).
Spam filtering for short messages. In 16th ACM Con-
ference on Information and Knowledge Management
(CIKM), pages 313–320.
Dong, Y., Oussalah, M., and Lov
´
en, L. (2017). A on
spam filtering classification: A majority voting like
approach. In KDIR, pages 293–301.
Gupta, V., Mehta, A., Goel, A., Dixit, U., and Pandey, A. C.
(2019). Spam detection using ensemble learning. In
Harmony Search and Nature Inspired Optimization
Algorithms, pages 661–668.
Healy, M., Delany, S. J., and Zamolotskikh, A. (2004). An
assessment of case base reasoning for short text mes-
sage classification. In 15th Irish Conference on Artifi-
cial Intelligence and Cognitive Sciences (AICS).
Hidalgo, J. M. G., Bringas, G. C., Sanz, E. P., and Garc
´
ıa,
F. C. (2006). Content based SMS spam filtering. In
ACM Symposium on Document Engineering, pages
107–114.
Ho, T. P., Kang, H., and Kim, S. (2013). Graph-based KNN
algorithm for spam SMS detection. Journal of Univer-
sal Computer Science (J. UCS), 19(16):2404–2419.
Junaid, M. B. and Farooq, M. (2011). Using evolutionary
learning classifiers to do mobile spam (SMS) filtering.
In 13th Annual Genetic and Evolutionary Computa-
tion Conference (GECCO), pages 1795–1802.
Karami, A. and Zhou, L. (2014). Improving static SMS
spam detection by using new content-based features.
In 20th Americas Conference on Information Systems
(AMCIS).
Kim, S.-E., Jo, J.-T., and Choi, S.-H. (2014). A spam mes-
sage filtering method: Focus on run time. Advanced
Science and Technology Letters, 76:29–33.
Lota, L. N. and Hossain, B. M. M. (2017). A systematic
literature review on sms spam detection techniques.
International Journal of Information Technology and
Computer Science, 9(7):42–50.
Mohasseb, A., Bader-El-Den, M., and Cocea, M. (2019). A
customised grammar framework for query classifica-
tion. Expert Systems with Applications, 135:164–180.
Mujtaba, G. and Yasin, M. (2014). SMS spam detection us-
ing simple message content features. Journal of Basic
and Applied Scientific Research, 4(4):275–279.
Popovac, M., Karanovic, M., Sladojevic, S., Arsenovic, M.,
and Anderla, A. (2018). Convolutional neural network
based SMS spam detection. In 26th Telecommunica-
tions Forum (TELFOR), pages 1–4.
Raj, H., Weihong, Y., Banbhrani, S. K., and Dino, S. P.
(2018). Lstm based short message service (SMS)
modeling for spam classification. In International
Conference on Machine Learning Technologies, pages
76–80.
Shirani-Mehr, H. (2013). SMS spam detection using ma-
chine learning approach.
Sun, Q., Qiao, H., and Luo, Z. (2008). The feature updating
algorithm for short message content filtering. Infor-
mation Technology Journal, 7(5):790–795.
Tekerek, A. (2019). Support vector machine based spam
SMS detection. Politeknik Dergisi, 22(3):779–784.
Uysal, A. K., Gunal, S., Ergin, S., and Gunal, E. S. (2013).
The impact of feature extraction and selection on
SMS spam filtering. Elektronika ir Elektrotechnika,
19(5):67–73.
Warade, S. J., Tijare, P. A., and Sawalkar, S. N. (2014).
An approach for SMS spam detection. International
Journal of Research in Advent Technology, 2(12):8–
11.
yan Zhang, H. and Wang, W. (2009). Application of
bayesian method to spam SMS filtering. In Inter-
national Conference on Information Engineering and
Computer Science, pages 1–3.
Zhang, L., Ma, J., and Wang, Y. (2013). Content based spam
text classification: An empirical comparison between
english and chinese. In 5th International Conference
on Intelligent Networking and Collaborative Systems,
pages 69–76.
DMMLACS 2020 - 1st International Special Session on Data Mining and Machine Learning Applications for Cyber Security
424