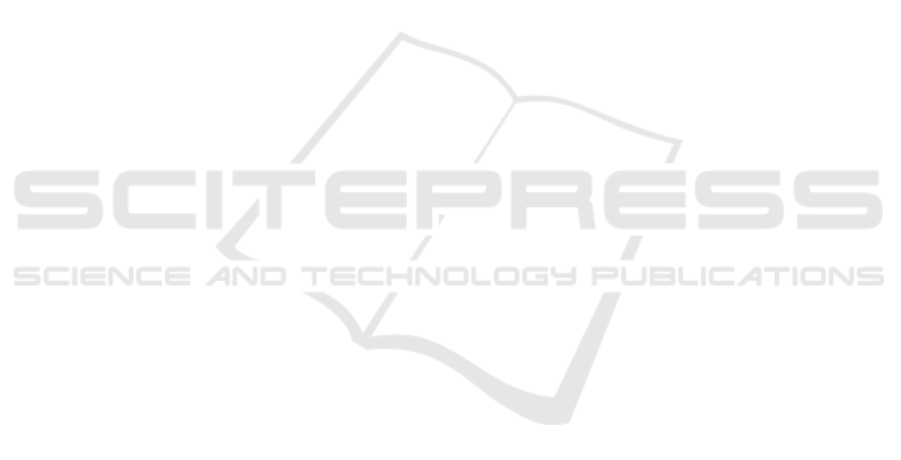
datasets for training to improve the cross-dataset per-
formance. Also a changed training process could be
executed. The network would be trained on imageNet
as a classifier. Before inference, the softmax, dropout
and avg. pooling layers would be stripped. This way
the amount of available training data is not the prob-
lem and the network could still work as feature ex-
tractor.
REFERENCES
Bak, S., Carr, P., and Lalonde, J.-F. (2018). Domain adap-
tation through synthesis for unsupervised person re-
identification. In Proceedings of the European Con-
ference on Computer Vision (ECCV), pages 189–205.
Bromley, J., Guyon, I., LeCun, Y., S
¨
ackinger, E., and Shah,
R. (1994). Signature verification using a” siamese”
time delay neural network. In Advances in neural in-
formation processing systems, pages 737–744.
Cao, Q., Shen, L., Xie, W., Parkhi, O. M., and Zisserman,
A. (2018). Vggface2: A dataset for recognising faces
across pose and age. In International Conference on
Automatic Face and Gesture Recognition.
Ge, Y., Li, Z., Zhao, H., Yin, G., Yi, S., Wang, X., et al.
(2018). Fd-gan: Pose-guided feature distilling gan for
robust person re-identification. In Advances in Neural
Information Processing Systems, pages 1222–1233.
Huang, G. B., Ramesh, M., Berg, T., and Learned-Miller,
E. (2007). Labeled faces in the wild: A database for
studying face recognition in unconstrained environ-
ments. Technical Report 07-49, University of Mas-
sachusetts, Amherst.
Liu, J., Ni, B., Yan, Y., Zhou, P., Cheng, S., and Hu, J.
(2018). Pose transferrable person re-identification. In
Proceedings of the IEEE Conference on Computer Vi-
sion and Pattern Recognition, pages 4099–4108.
Ma, L., Jia, X., Sun, Q., Schiele, B., Tuytelaars, T., and
Van Gool, L. (2017). Pose guided person image gener-
ation. In Advances in Neural Information Processing
Systems, pages 406–416.
Qian, X., Fu, Y., Xiang, T., Wang, W., Qiu, J., Wu, Y., Jiang,
Y.-G., and Xue, X. (2018). Pose-normalized image
generation for person re-identification. In Proceed-
ings of the European conference on computer vision
(ECCV), pages 650–667.
Ristani, E., Solera, F., Zou, R., Cucchiara, R., and Tomasi,
C. (2016). Performance measures and a data set
for multi-target, multi-camera tracking. In Euro-
pean Conference on Computer Vision, pages 17–35.
Springer.
Schroff, F., Kalenichenko, D., and Philbin, J. (2015).
Facenet: A unified embedding for face recognition
and clustering. In Proceedings of the IEEE conference
on computer vision and pattern recognition, pages
815–823.
Szegedy, C., Ioffe, S., Vanhoucke, V., and Alemi, A. A.
(2017). Inception-v4, inception-resnet and the impact
of residual connections on learning. In Thirty-First
AAAI Conference on Artificial Intelligence.
Weinberger, K. Q. and Saul, L. K. (2009). Distance met-
ric learning for large margin nearest neighbor clas-
sification. Journal of Machine Learning Research,
10(Feb):207–244.
Zheng, L., Shen, L., Tian, L., Wang, S., Wang, J., and Tian,
Q. (2015). Scalable person re-identification: A bench-
mark. In Proceedings of the IEEE international con-
ference on computer vision, pages 1116–1124.
Zheng, Z., Yang, X., Yu, Z., Zheng, L., Yang, Y., and Kautz,
J. (2019). Joint discriminative and generative learn-
ing for person re-identification. IEEE Conference on
Computer Vision and Pattern Recognition (CVPR).
Zheng, Z., Zheng, L., and Yang, Y. (2017). Unlabeled
samples generated by gan improve the person re-
identification baseline in vitro. In Proceedings of the
IEEE International Conference on Computer Vision,
pages 3754–3762.
Evaluating Person Re-identification Performance on GAN-enhanced Datasets
81