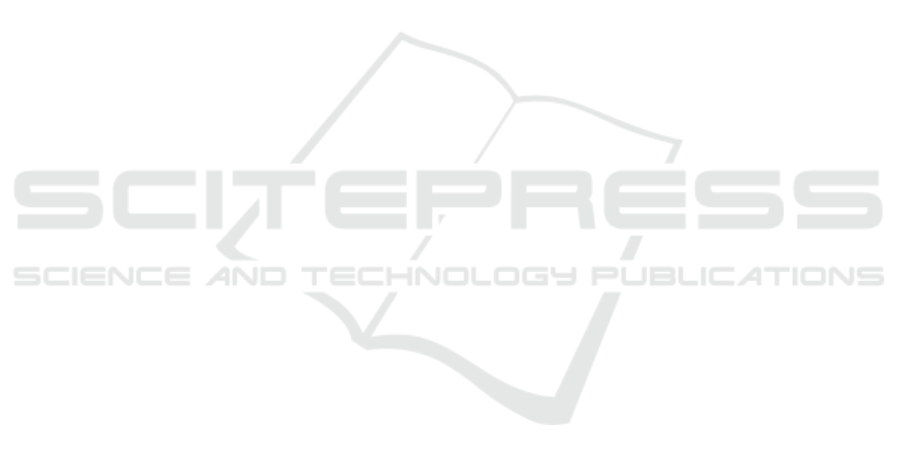
ing step. Regarding the FSI approach, which is still in
its preliminary stage, many directions are looking for-
ward. We expect to provide more performative meth-
ods in (i) identifying scenes with further algorithms,
(ii) selecting scene frames, and (iii) ranking candidate
frames.
ACKNOWLEDGEMENTS
This research has been partially supported by
the ”Bando Aiuti per progetti di Ricerca e
Sviluppo”—POR FESR6832014-2020—Asse 1,
Azione 1.1.3. Project VideoBrain- Intelligent Video
Optmization.
REFERENCES
Ames, M. and Naaman, M. (2007). Why We Tag: Moti-
vations for Annotation in Mobile and Online Media.
CHI ’07. Association for Computing Machinery, New
York, NY, USA.
Gao, Y., Zhang, T., and Xiao, J. (2009). Thematic video
thumbnail selection. In Proc. of the 16th IEEE Int.
Conf. on Image Processing, ICIP’09, pages 4277–
4280, Piscataway, NJ, USA. IEEE Press.
Hasler, D. and S
¨
usstrunk, S. (2003). Measuring colourful-
ness in natural images. Human Vision and Electronic
Imaging.
Kang, H.-W. and Hua, X.-S. (2005). To learn representa-
tiveness of video frames. In Proceedings of the 13th
Annual ACM International Conference on Multime-
dia, MULTIMEDIA ’05, page 423–426, New York,
NY, USA. Association for Computing Machinery.
Lee, Y. J., Ghosh, J., and Grauman, K. (2012). Discover-
ing important people and objects for egocentric video
summarization. In 2012 IEEE Conference on Com-
puter Vision and Pattern Recognition, pages 1346–
1353.
Li, H., Yi, L., Liu, B., and Wang, Y. (2014). Localizing rel-
evant frames in web videos using topic model and rel-
evance filtering. Mach. Vis. Appl., pages 1661–1670.
Lin, T.-Y., Maire, M., Belongie, S., Bourdev, L., Girshick,
R., Hays, J., Perona, P., Ramanan, D., Zitnick, C. L.,
and Doll
´
ar, P. (2014). Microsoft coco: Common ob-
jects in context.
Liu, C., Huang, Q., and Jiang, S. (2011). Query sensitive
dynamic web video thumbnail generation. In 2011
18th IEEE International Conference on Image Pro-
cessing, pages 2449–2452.
Liu, W., Mei, T., Zhang, Y., Che, C., and Luo, J. (2015).
Multi-task deep visual-semantic embedding for video
thumbnail selection. In CVPR, pages 3707–3715.
IEEE Computer Society.
Pearson, K. (1895). Note on regression and inheritance in
the case of two parents. Proceedings of the Royal So-
ciety of London, 58:240–242.
Potapov, D., Douze, M., Harchaoui, Z., and Schmid, C.
(2014). Category-specific video summarization. In
Fleet, D., Pajdla, T., Schiele, B., and Tuytelaars,
T., editors, ECCV - European Conference on Com-
puter Vision, volume 8694 of Lecture Notes in Com-
puter Science, pages 540–555, Zurich, Switzerland.
Springer.
Rav-Acha, A., Pritch, Y., and Peleg, S. (2006). Making a
long video short: Dynamic video synopsis. In 2006
IEEE Computer Society Conference on Computer Vi-
sion and Pattern Recognition (CVPR’06), volume 1,
pages 435–441.
Redmon, J., Divvala, S., Girshick, R., and Farhadi, A.
(2015). You only look once: Unified, real-time ob-
ject detection. cite arxiv:1506.02640.
Song, Y., Redi, M., Vallmitjana, J., and Jaimes, A. (2016).
To click or not to click: Automatic selection of beau-
tiful thumbnails from videos. In Mukhopadhyay, S.,
Zhai, C., Bertino, E., Crestani, F., Mostafa, J., Tang,
J., Si, L., Zhou, X., Chang, Y., Li, Y., and Sondhi, P.,
editors, CIKM, pages 659–668. ACM.
Wang, Y., Han, B., Li, D., and Thambiratnam, K. (2018).
Compact web video summarization via supervised
learning. In 2018 IEEE International Conference on
Multimedia Expo Workshops (ICMEW), pages 1–4.
Zhang, K., Chao, W., Sha, F., and Grauman, K. (2016).
Summary transfer: Exemplar-based subset selection
for video summarization. CoRR, abs/1603.03369.
WEBIST 2020 - 16th International Conference on Web Information Systems and Technologies
216