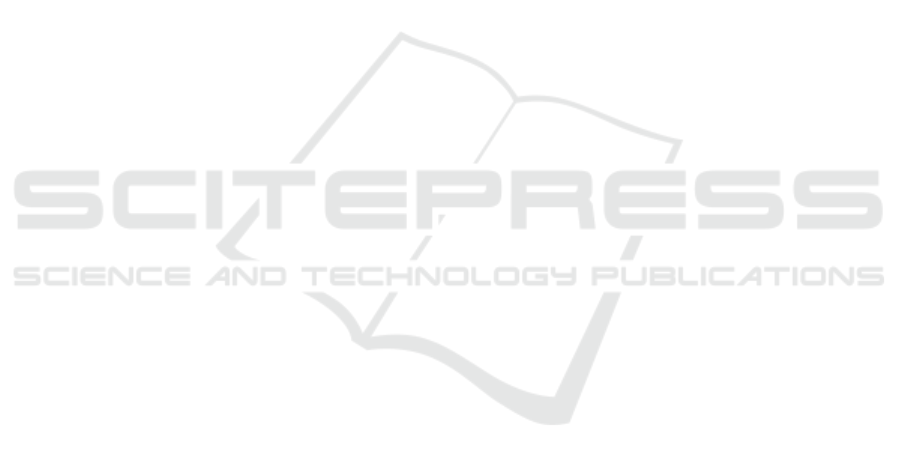
Fortin, M. and Chaib-draa, B. (2019). Multimodal multi-
task emotion recognition using images, texts and tags.
pages 3–10.
French, J. H. (2017). Image-based memes as sentiment pre-
dictors. In 2017 International Conference on Infor-
mation Society (i-Society), pages 80–85. IEEE.
Geman, D., Geman, S., Hallonquist, N., and Younes, L.
(2015). Visual turing test for computer vision systems.
Proceedings of the National Academy of Sciences of
the United States of America, 112.
Gurari, D., Zhao, Y., Zhang, M., and Bhattacharya, N.
(2020). Captioning images taken by people who are
blind.
He, K., Zhang, X., Ren, S., and Sun, J. (2015). Deep
residual learning for image recognition. CoRR,
abs/1512.03385.
Hudson, D. A. and Manning, C. D. (2019). Gqa: A new
dataset for real-world visual reasoning and composi-
tional question answering.
Klein, B., Lev, G., Sadeh, G., and Wolf, L. (2015). Associ-
ating neural word embeddings with deep image repre-
sentations using fisher vectors. pages 4437–4446.
Krishna, R., Zhu, Y., Groth, O., Johnson, J., Hata, K.,
Kravitz, J., Chen, S., Kalantidis, Y., Li, L., Shamma,
D. A., Bernstein, M. S., and Li, F. (2016). Vi-
sual genome: Connecting language and vision us-
ing crowdsourced dense image annotations. CoRR,
abs/1602.07332.
Krizhevsky, A., Sutskever, I., and Hinton, G. E. (2012).
Imagenet classification with deep convolutional neu-
ral networks. In Pereira, F., Burges, C. J. C., Bottou,
L., and Weinberger, K. Q., editors, Advances in Neu-
ral Information Processing Systems 25, pages 1097–
1105. Curran Associates, Inc.
Mohammad, S. M. (2018). Word affect intensities. In
Proceedings of the 11th Edition of the Language
Resources and Evaluation Conference (LREC-2018),
Miyazaki, Japan.
Mu, Y., Yan, S., Liu, Y., Huang, T., and Zhou, B. (2008).
Discriminative local binary patterns for human detec-
tion in personal album.
Nagda, M. and Eswaran, P. (2019). Image classification
using a hybrid lstm-cnn deep neural network. 8.
Peirson, V., Abel, L., and Tolunay, E. M. (2018). Dank
learning: Generating memes using deep neural net-
works. arXiv preprint arXiv:1806.04510.
Pennington, J., Socher, R., and Manning, C. (2014). Glove:
Global vectors for word representation. volume 14,
pages 1532–1543.
Porwik, P. and Lisowska, A. (2004). The haar-wavelet
transform in digital image processing: its status and
achievements. 13.
Russakovsky, O., Deng, J., Su, H., Krause, J., Satheesh, S.,
Ma, S., Huang, Z., Karpathy, A., Khosla, A., Bern-
stein, M. S., Berg, A. C., and Li, F. (2014). Ima-
genet large scale visual recognition challenge. CoRR,
abs/1409.0575.
Savva, M., Kadian, A., Maksymets, O., Zhao, Y., Wijmans,
E., Jain, B., Straub, J., Liu, J., Koltun, V., Malik, J.,
Parikh, D., and Batra, D. (2019). Habitat: A platform
for embodied ai research.
Sidorov, O., Hu, R., Rohrbach, M., and Singh, A. (2020).
Textcaps: a dataset for image captioning with reading
comprehension.
Simonyan, K. and Zisserman, A. (2014). Very deep con-
volutional networks for large-scale image recognition.
arXiv 1409.1556.
Singh, A., Natarajan, V., Shah, M., Jiang, Y., Chen, X., Ba-
tra, D., Parikh, D., and Rohrbach, M. (2019). Towards
vqa models that can read.
Singh, N., Singh, K., and Sinha, A. (2012). A novel ap-
proach for content based image retrieval. Procedia
Technology, 4:245–250.
Sonnad, N. (2018). The world’s biggest meme is the word
“meme” itself.
Sorokin, A. and Forsyth, D. (2008). Utility data annotation
with amazon mechanical turk. In 2008 IEEE Com-
puter Society Conference on Computer Vision and
Pattern Recognition Workshops, pages 1–8.
Thomee, B., Shamma, D. A., Friedland, G., Elizalde, B.,
Ni, K., Poland, D., Borth, D., and Li, L. (2015). The
new data and new challenges in multimedia research.
CoRR, abs/1503.01817.
Vinyals, O., Toshev, A., Bengio, S., and Erhan, D. (2015).
Show and tell: A neural image caption generator.
pages 3156–3164.
Wang, Y. and Liu, Q. (2017). Visual and textual sentiment
analysis using deep fusion convolutional neural net-
works.
Xuelin, Z., Cao, B., Xu, S., Liu, B., and Cao, J. (2019). Joint
Visual-Textual Sentiment Analysis Based on Cross-
Modality Attention Mechanism: MMM 2019, pages
264–276.
Young, P., Lai, A., Hodosh, M., and Hockenmaier, J.
(2014). From image descriptions to visual denota-
tions: New similarity metrics for semantic inference
over event descriptions. Transactions of ACL, 2.
Yuvaraju, M., Sheela, K., and Sobana Rani, S. (2015). Fea-
ture extraction of real-time image using sift algorithm.
IJEEE, 3:1–7.
WEBIST 2020 - 16th International Conference on Web Information Systems and Technologies
338