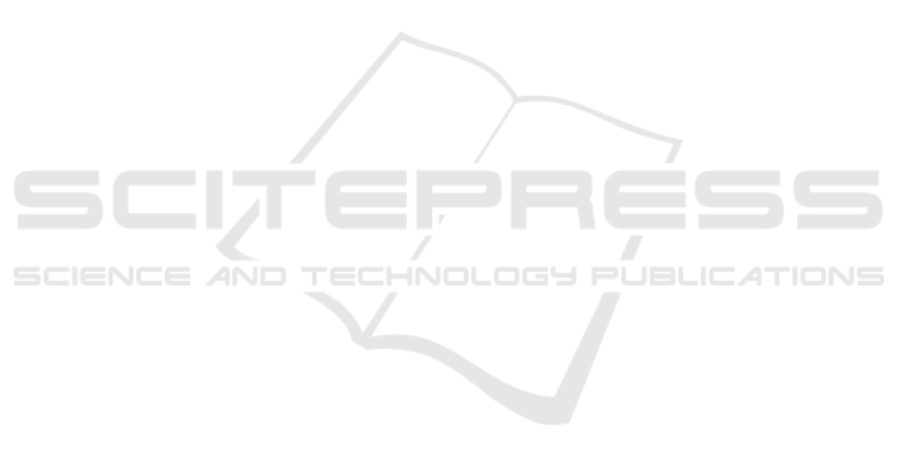
tection: A gru-based gaussian mixture variational au-
toencoder approach. In Asian Conference on Machine
Learning, pages 97–112.
High-Level Expert Group on Artificial Intelligence
(2019). Ethics guidelines for trustworthy ai.
https://ec.europa.eu/digital-single-market/en/news/
ethics-guidelines-trustworthy-ai. (Accessed on
09/28/2020).
Hwang, Y., Tong, A., and Choi, J. (2016). Automatic con-
struction of nonparametric relational regression mod-
els for multiple time series. In Balcan, M. F. and Wein-
berger, K. Q., editors, ICML 2016: Proceedings of the
33rd International Conference on Machine Learning,
volume 48 of Proceedings of Machine Learning Re-
search, pages 3030–3039. PLMR.
Ioannou, C., Vassiliou, V., and Sergiou, C. (2017). An intru-
sion detection system for wireless sensor networks. In
2017 24th International Conference on Telecommuni-
cations (ICT), pages 1–5. IEEE.
Kawachi, Y., Koizumi, Y., and Harada, N. (2018). Com-
plementary set variational autoencoder for supervised
anomaly detection. In 2018 IEEE International Con-
ference on Acoustics, Speech and Signal Processing
(ICASSP), pages 2366–2370. IEEE.
Kim, H. and Teh, Y. W. (2018). Scaling up the Automatic
Statistician: Scalable structure discovery using Gaus-
sian processes. In Proceedings of the 21st Interna-
tional Conference on Artificial Intelligence and Statis-
tics, volume 84.
Kingma, D. P. and Welling, M. (2014). Auto-encoding vari-
ational bayes. In ICLR.
Kwon, D., Kim, H., Kim, J., Suh, S. C., Kim, I., and Kim,
K. J. (2019). A survey of deep learning-based network
anomaly detection. Cluster Computing, pages 1–13.
Lange-Hegermann, M. (2018). Algorithmic linearly con-
strained gaussian processes. In NeurIPS, pages 2141–
2152.
Lange-Hegermann, M. (2020). Linearly constrained gaus-
sian processes with boundary conditions. CoRR,
abs/2002.00818.
Li, D., Chen, D., Jin, B., Shi, L., Goh, J., and Ng, S.-K.
(2019). Mad-gan: Multivariate anomaly detection for
time series data with generative adversarial networks.
In International Conference on Artificial Neural Net-
works, pages 703–716. Springer.
Liu, F. T., Ting, K. M., and Zhou, Z.-H. (2008). Isolation
forest. In Giannotti, F., editor, Eighth IEEE Interna-
tional Conference on Data Mining, 2008, pages 413–
422, Piscataway, NJ. IEEE.
Lloyd, J. R., Duvenaud, D., Grosse, R. B., Tenenbaum,
J. B., and Ghahramani, Z. (2014). Automatic con-
struction and natural-language description of nonpara-
metric regression models. In AAAI, pages 1242–1250.
AAAI Press.
M
¨
uller, A., Lange-Hegermann, M., and von Birgelen,
A. (2020). Automatisches training eines varia-
tional autoencoder f
¨
ur anomalieerkennung in zeitrei-
hen. In VDI Kongress Automation 2020, volume VDI-
Berichte 2375, pages 687–698, Baden-Baden. VDI
Wissensforum GmbH, VDI Verlag GmbH.
Niggemann, O. and Lohweg, V. (2015). On the diagnosis of
cyber-physical production systems. In Twenty-Ninth
AAAI Conference on Artificial Intelligence.
Pedregosa, F., Varoquaux, G., Gramfort, A., Michel, V.,
Thirion, B., Grisel, O., Blondel, M., Prettenhofer, P.,
Weiss, R., Dubourg, V., Vanderplas, J., Passos, A.,
Cournapeau, D., Brucher, M., Perrot, M., and Duch-
esnay, E. (2011). Scikit-learn: Machine learning in
python. J. Mach. Learn. Res., 12:2825–2830.
Phua, C., Lee, V. C. S., Smith-Miles, K., and Gayler, R. W.
(2010). A comprehensive survey of data mining-based
fraud detection research. CoRR, abs/1009.6119.
Rajpurkar, P., Hannun, A. Y., Haghpanahi, M., Bourn, C.,
and Ng, A. Y. (2017). Cardiologist-level arrhythmia
detection with convolutional neural networks. CoRR,
abs/1707.01836.
Rasmussen, C. E. and Williams, C. K. I. (2006). Gaussian
Processes for Machine Learning (Adaptive Computa-
tion And Machine Learning). The MIT Press.
Renaudie, D., Zuluaga, M. A., and Acuna-Agost, R. (2018).
Benchmarking anomaly detection algorithms in an in-
dustrial context: Dealing with scarce labels and multi-
ple positive types. In IEEE International Conference
on Big Data, pages 1227–1236.
Rezende, D. J. and Mohamed, S. (2015). Variational infer-
ence with normalizing flows. In ICML, volume 37 of
JMLR Workshop and Conference Proceedings, pages
1530–1538. JMLR.org.
Rousseeuw, P. J. (1984). Least median of squares regres-
sion. Journal of the American statistical association,
79(388):871–880.
Sabokrou, M., Khalooei, M., Fathy, M., and Adeli, E.
(2018). Adversarially learned one-class classifier for
novelty detection. In Proceedings of the IEEE Con-
ference on Computer Vision and Pattern Recognition,
pages 3379–3388.
Sch
¨
olkopf, B., Platt, J. C., Shawe-Taylor, J. C., Smola, A. J.,
and Williamson, R. C. (2001). Estimating the support
of a high-dimensional distribution. Neural Comput.,
13(7):1443–1471.
Snelson, E. and Ghahramani, Z. (2007). Local and global
sparse gaussian process approximations. In AIS-
TATS, volume 2 of JMLR Proceedings, pages 524–
531. JMLR.org.
Sorournejad, S., Zojaji, Z., Atani, R. E., and Monadjemi,
A. H. (2016). A survey of credit card fraud detection
techniques: Data and technique oriented perspective.
CoRR, abs/1611.06439.
Steinruecken, C., Smith, E., Janz, D., Lloyd, J. R., and
Ghahramani, Z. (2019). The automatic statistician.
In Automated Machine Learning, The Springer Series
on Challenges in Machine Learning, pages 161–173.
Springer.
Stojanovic, L., Dinic, M., Stojanovic, N., and Stojadi-
novic, A. (2016). Big-data-driven anomaly detection
in industry (4.0): An approach and a case study. In
2016 IEEE International Conference on Big Data (Big
Data), pages 1647–1652.
Suh, S., Chae, D. H., Kang, H.-G., and Choi, S. (2016).
Echo-state conditional variational autoencoder for
Towards Gaussian Processes for Automatic and Interpretable Anomaly Detection in Industry 4.0
91