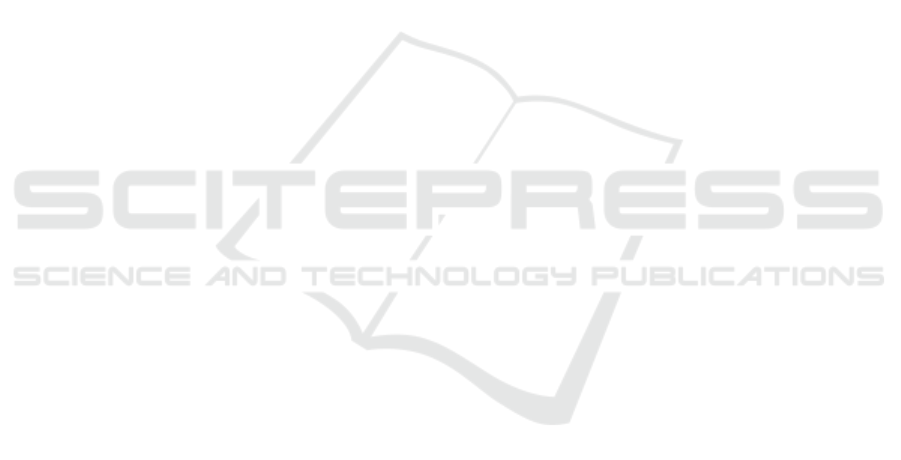
sion agriculture and plant phenotyping. Plant Disease,
100(2):241–251.
Mart
´
ınez-Plumed, F., Contreras-Ochando, L., Ferri, C.,
Orallo, J. H., Kull, M., Lachiche, N., Quintana, M.
J. R., and Flach, P. A. (2019). CRISP-DM twenty
years later: From data mining processes to data sci-
ence trajectories. IEEE Transactions on Knowledge
and Data Engineering.
Mohanty, S. P., Hughes, D. P., and Salath
´
e, M. (2016). Us-
ing deep learning for image-based plant disease detec-
tion. Frontiers in Plant Science, 7:1419.
Munn
´
e, R. and Del Hoyo, R. (2019). Grapevine-hiGh
performance comPuting sErvices for preVention and
https://grapevine-project.eu/. Accessed: 2020-07-15.
Najafabadi, M. M., Villanustre, F., Khoshgoftaar, T. M.,
Seliya, N., Wald, R., and Muharemagic, E. (2015).
Deep learning applications and challenges in big data
analytics. Journal of Big Data, 2(1):1.
Nguyen, L. H. and Henebry, G. M. (2019). Characteriz-
ing land use/land cover using multi-sensor time series
from the perspective of land surface phenology. Re-
mote Sensing, 11(14):1677.
Pham, X. and Stack, M. (2018). How data analytics is trans-
forming agriculture. Business Horizons, 61(1):125–
133.
Prusinkiewicz, P. and Runions, A. (2012). Computational
models of plant development and form. New Phytolo-
gist, 193(3):549–569.
Pytharoulis, I., Kotsopoulos, S., Tegoulias, I., Kartsios, S.,
Bampzelis, D., and Karacostas, T. (2016). Numeri-
cal modeling of an intense precipitation event and its
associated lightning activity over northern greece. At-
mospheric Research, 169:523–538.
Reaumur, R. A. F. (1735). Thermometric observations made
at Paris during the year 1735, compared to those made
below the equator on the isle of Mauritius, at Algiers
and on a few of our American islands. M
´
emoires de
l’Acad
´
emie royale des sciences (Paris).
Reed, B. C., Schwartz, M. D., and Xiao, X. (2009). Re-
mote sensing phenology. In Phenology of Ecosystem
Processes, pages 231–246. Springer.
Reynolds, M., Kropff, M., Crossa, J., Koo, J., Kruseman,
G., Molero Milan, A., Rutkoski, J., Schulthess, U.,
Sonder, K., Tonnang, H., et al. (2018). Role of mod-
elling in international crop research: overview and
some case studies. Agronomy, 8(12):291.
Ribeiro, H., Pi
˜
na-Rey, A., Abreu, I., Rodr
´
ıguez-Rajo, F. J.,
et al. (2020). Integrating phenological, aerobiological
and weather data to study the local and regional flow-
ering dynamics of four grapevine cultivars. Agron-
omy, 10(2):185.
Rodr
´
ıguez Galiano, V. F., S
´
anchez Castillo, M., Dash, J.,
Atkinson, P., and Ojeda Z
´
ujar, J. (2016). Modelling
interannual variation in the spring and autumn land
surface phenology of the European forest. Biogeo-
sciences, 13:3305–3317.
R
¨
otzer, T. and Chmielewski, F.-M. (2001). Phenological
maps of Europe. Climate Research, 18(3):249–257.
Schwartz, M. D. et al. (2003). Phenology: an integrative
environmental science. Springer.
Sirsat, M. S., Mendes-Moreira, J., Ferreira, C., and Cunha,
M. (2019). Machine learning predictive model of
grapevine yield based on agroclimatic patterns. En-
gineering in Agriculture, Environment and Food,
12(4):443–450.
Skawsang, S., Nagai, M., K Tripathi, N., and Soni, P.
(2019). Predicting rice pest population occurrence
with satellite-derived crop phenology, ground mete-
orological observation, and machine learning: A case
study for the central plain of Thailand. Applied Sci-
ences, 9(22):4846.
St
¨
ockli, R., Rutishauser, T., Dragoni, D., O’keefe, J., Thorn-
ton, P., Jolly, M., Lu, L., and Denning, A. (2008). Re-
mote sensing data assimilation for a prognostic phe-
nology model. Journal of Geophysical Research: Bio-
geosciences, 113(G4).
Tang, J., K
¨
orner, C., Muraoka, H., Piao, S., Shen, M.,
Thackeray, S. J., and Yang, X. (2016). Emerging
opportunities and challenges in phenology: a review.
Ecosphere, 7(8).
Taylor, S. D. and White, E. P. (2020). Automated data-
intensive forecasting of plant phenology through-
out the United States. Ecological Applications,
30(1):e02025.
Tonnang, H. E., Makumbi, D., and Craufurd, P. (2018).
Methodological approach for predicting and mapping
the phenological adaptation of tropical maize (Zea
mays L.) using multi-environment trials. Plant Meth-
ods, 14(1):108.
Wang, H. (2017). Crop assessment and monitoring using
optical sensors. PhD thesis, Kansas State University.
Wang, Y., Case, B., Rossi, S., Dawadi, B., Liang, E., and El-
lison, A. M. (2019). Frost controls spring phenology
of juvenile smith fir along elevational gradients on the
southeastern tibetan plateau. International Journal of
Biometeorology, 63(7):963–972.
Wirth, R. and Hipp, J. (2000). CRISP-DM: Towards a stan-
dard process model for data mining. In Fourth Inter-
national Conference on the Practical Applications of
Knowledge Discovery and Data Mining, pages 29–39.
Springer.
Wolfert, S., Ge, L., Verdouw, C., and Bogaardt, M.-J.
(2017). Big data in smart farming–a review. Agri-
cultural Systems, 153:69–80.
Zeng, L., Wardlow, B. D., Xiang, D., Hu, S., and Li, D.
(2020). A review of vegetation phenological metrics
extraction using time-series, multispectral satellite
data. Remote Sensing of Environment, 237:111511.
Zhang, N., Wang, M., and Wang, N. (2002). Precision agri-
culture—a worldwide overview. Computers and Elec-
tronics in Agriculture, 36(2-3):113–132.
Zhao, M., Peng, C., Xiang, W., Deng, X., Tian, D., Zhou,
X., Yu, G., He, H., and Zhao, Z. (2013). Plant pheno-
logical modeling and its application in global climate
change research: overview and future challenges. En-
vironmental Reviews, 21(1):1–14.
WEBIST 2020 - 16th International Conference on Web Information Systems and Technologies
82