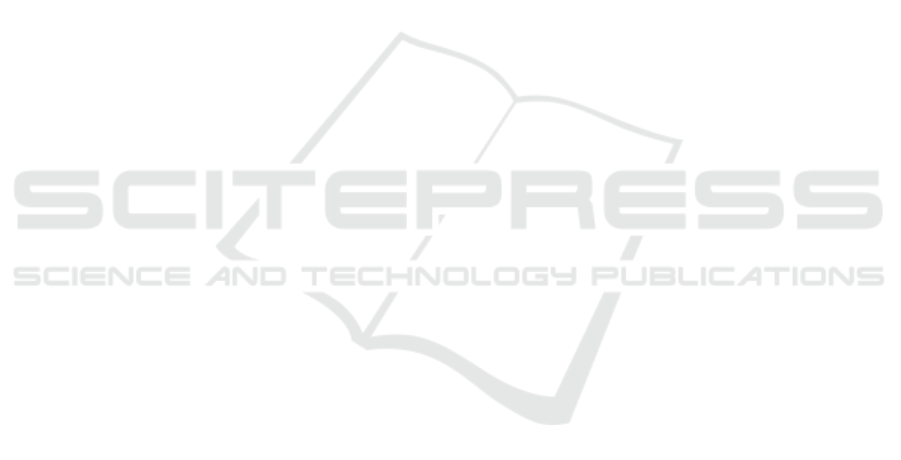
based on standards such as OWL-S and WSMO. The
semantic services are enriched with inputs, outputs,
preconditions, and results with postconditions. Addi-
tionally, we have integrated our approach in a cyber-
physical business process management architectural
model. In our feasibility test, we have exemplary
demonstrated that the developed SOA is suitable to
be used in state-of-the-art workflow management sys-
tems to build valid cyber-physical production work-
flows. By using semantic web services as pre- and
postconditions of other semantic services, a near real-
time verification for executing cyber-physical work-
flows is ensured. Additionally, the developed services
provide the foundation to support low-code applica-
tions (Sanchis et al., 2020).
In future work, we investigate the described
use cases further to enhance workflow flexibility in
cyber-physical production systems. We assume that
Process-Oriented Case-Based Reasoning (POCBR),
for example, could be used for this purpose because it
has already shown great potential in various other do-
mains. Additionally, automated planning techniques
can be used to further increase workflow flexibility in
Industry 4.0. Therefore, the pre- and postconditions
of the semantic web services can be transferred into a
planning model and directly used during planning. In
this context, we plan to conduct an comprehensive ex-
perimental evaluation that assess the appropriateness
of the presented approach in more detail. With the fu-
ture application, the semantic web services are contin-
uously improved according to their environment and
requirements (see Application & Evolution phase).
ACKNOWLEDGEMENTS
This work is funded by the German Research Founda-
tion (DFG) under grant No. BE 1373/3-3. The basic
components of the semantic web services were devel-
oped in a student research project by Felix Reither and
Julian Sawatzki and revised by Marcel Mischo.
REFERENCES
Abele, E., Chryssolouris, G., Sihn, W., Metternich, J., El-
Maraghy, H., Seliger, G., Sivard, G., ElMaraghy, W.,
Hummel, V., Tisch, M., and Seifermann, S. (2017).
Learning factories for future oriented research and ed-
ucation in manufacturing. CIRP Ann., 66(2):803–826.
Bordel Sánchez, B., Alcarria, R., Sánchez de Rivera, D.,
and Robles, T. (2018). Process execution in Cyber-
Physical Systems using cloud and Cyber-Physical In-
ternet services. J. Supercomput., 74(8):4127–4169.
Boschert, S. and Rosen, R. (2016). Digital Twin—The Sim-
ulation Aspect. In Mechatron. Futur., pages 59–74.
Springer.
Broy, M., Cengarle, M. V., and Geisberger, E. (2012).
Cyber-Physical Systems: Imminent Challenges. In
Large-Scale Complex IT Syst. Dev., Operat. and
Manag. - 17th Monterey Workshop, volume 7539 of
LNCS, pages 1–28. Springer.
Burns, T., Cosgrove, J., and Doyle, F. (2019). A Review of
Interoperability Standards for Industry 4.0. Procedia
Manuf., 38:646–653.
Calà, A., Ryashentseva, D., and Lüder, A. (2016). Modeling
approach for a flexible manufacturing control system.
In 21st Int. Conf. on Emerg. Technol. and Factory Au-
tomat., pages 1–4. IEEE.
Chen, L. and Yang, X. (2005). Applying AI Planning to Se-
mantic Web Services for Workflow Generation. In Int.
Conf. on Semant., Knowl. and Grid, page 65. IEEE.
Cheng, H., Xue, L., Wang, P., Zeng, P., and Yu, H. (2017).
Ontology-based web service integration for flexible
manufacturing systems. In 15th Int. Conf. on Ind. Inf.,
pages 351–356. IEEE.
Ciortea, A., Mayer, S., and Michahelles, F. (2018). Repur-
posing Manufacturing Lines on the Fly with Multi-
agent Systems for the Web of Things. In Proc. of
the 17th Int. Conf. on Autonomous Agents and Multi-
Agent Systems, pages 813–822. Int. Found. for Au-
tonomous Agents and Multiagent Systems / ACM.
Hepp, M., Leymann, F., Domingue, J., Wahler, A., and
Fensel, D. (2005). Semantic business process man-
agement: a vision towards using semantic Web ser-
vices for business process management. In Int. Conf.
on e-Business Eng., pages 535–540. IEEE.
Humm, B., Bense, H., Bock, J., Classen, M., Halvani, O.,
Herta, C., Hoppe, T., Juwig, O., and Siegel, M. (2020).
Applying machine intelligence in practice. Informatik
Spektrum.
Jammes, F. and Smit, H. (2005). Service-Oriented
Paradigms in Industrial Automation. IEEE Trans. Ind.
Inf., 1(1):62–70.
Janiesch, C., Koschmider, A., Mecella, M., Weber, B., Bu-
rattin, A., Ciccio, C. D., Fortino, G., Gal, A., Kan-
nengiesser, U., Leotta, F., Mannhardt, F., Marrella, A.,
Mendling, J., Oberweis, A., Reichert, M., Rinderle-
Ma, S., Serral, E., Song, W., Su, J., Torres, V., Wei-
dlich, M., Weske, M., and Zhang, L. (2020). The
Internet-of-Things Meets Business Process Manage-
ment. A Manifesto. IEEE Syst. Man Cybern. Mag.
Janowicz, K., Haller, A., Cox, S. J., Le Phuoc, D., and
Lefrançois, M. (2019). SOSA: A lightweight ontol-
ogy for sensors, observations, samples, and actuators.
J. Web Semant., 56:1–10.
Järvenpää, E., Siltala, N., Hylli, O., and Lanz, M. (2019).
The development of an ontology for describing the
capabilities of manufacturing resources. J. Intell.
Manuf., 30(2):959–978.
Klein, P. and Bergmann, R. (2019). Generation of Complex
Data for AI-Based Predictive Maintenance Research
With a Physical Factory Model. In 16th Int. Conf. on
Inform. in Control Automat. and Rob., pages 40–50.
SciTePress.
IN4PL 2020 - International Conference on Innovative Intelligent Industrial Production and Logistics
42