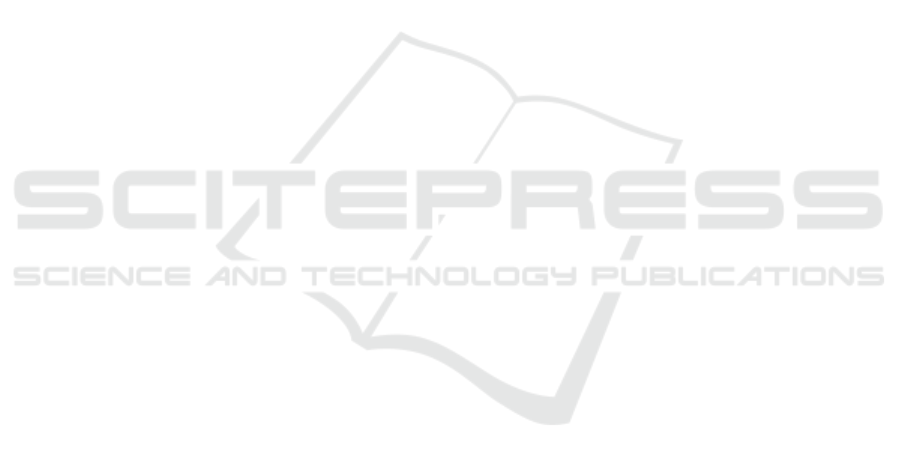
actions that should look realistic which is especially
difficult to achieve from motion-capture data (Bragg
et al., 2019). It gets even more challenging because
movements can convey endless nuances (Bragg et al.,
2019). A similar issue arises due to the numerous
possible combinations of signs, according to (Bragg
et al., 2019).
One approach to improve prosody in sign lan-
guage avatars by (Adamo-Villani and Wilbur, 2015) is
described in section 2.2.2. So far, realism of signing
avatars seemed to be the goal. However, avatars also
face the uncanny valley problem. That means that
strong but not fully realistic avatars are not received
as pleasing, which could explain that the cartoonish
avatar was ranked best in the study by (Kipp et al.,
2011b). Lastly, the acceptance of signing avatars will
likely rise with the involvement of the Deaf commu-
nity in research and development of sign language
systems, as described in section 3.1.5. (Kipp et al.,
2011b) found that participation of Deaf persons in
such studies significantly increases the positive opin-
ion about signing avatars.
3.2 Opportunities and Visions
When the challenges outlined in section 3.1 can
be resolved, opportunities for automated sign lan-
guage translation systems are enormous. Especially
a standard notation and bigger sign language datasets
could significantly evolve training and performance
of sign language recognition and generation technolo-
gies (Bragg et al., 2019; De Martino et al., 2017).
They would also entail numerous advantages of their
own – such as a written form of sign languages, ac-
curate dictionaries and better resources for learning
sign languages (Bragg et al., 2019). In the near fu-
ture, static one-way information could be presented
through signing avatars, for example next to text on
websites. This is already the case in some small-scale
projects, for instance a part of the website of the city
of Hamburg (Elliott et al., 2008).
Various research has been conducted in an edu-
cational context, not only for making material more
accessible to students using sign language, but to as-
sist those who want to learn a sign language, too.
One example is the system that (Deb et al., 2017) de-
scribe. An Augmented Reality (AR) application was
developed that presented 3D animations of signs on
mobile devices via AR as an overlay to the scanned
Hindi letters. This involves various technologies of
image capturing, processing, marker tracking, anima-
tion rendering and augmented display. If it could be
extended to whole texts and transferred to different
languages and sign languages, there would be numer-
ous use cases for this system.
Involving the additional step of speech processing,
students of the NYU have developed a proof of con-
cept app that can translate American Sign Language
to English speech as well as vice versa and display
a signing avatar through augmented reality (Polun-
ina, 2018). Unfortunately, technological details are
not given. Taking this concept further, a daily life
application based on smartphone technologies could
be developed and automatically translate speech to
sign language and vice versa. A range of (spoken and
signed) languages could be supported and the signer
might additionally be able to choose or individualize
the signing avatar.
Concluding, the mentioned approaches are
promising. In the future, they could enable sign
language users to access personal assistants, to use
text-based systems, to search sign language video
content and to use automated real-time translation
when human interpreters are not available (Bragg
et al., 2019). With the help of AI, automated sign
language translation systems could help break down
communication barriers for DHH individuals.
REFERENCES
Adamo-Villani, N. and Wilbur, R. B. (2015). ASL-Pro:
American Sign Language Animation with Prosodic
Elements. In Antona, M. and Stephanidis, C., edi-
tors, Universal Access in Human-Computer Interac-
tion. Access to Interaction, volume 9176, pages 307–
318. Springer International Publishing, Cham.
Al-khazraji, S., Berke, L., Kafle, S., Yeung, P., and Huener-
fauth, M. (2018). Modeling the Speed and Timing of
American Sign Language to Generate Realistic An-
imations. In Proceedings of the 20th International
ACM SIGACCESS Conference on Computers and Ac-
cessibility - ASSETS ’18, pages 259–270, Galway, Ire-
land. ACM Press.
Bavelier, D., Newport, E. L., and Supalla, T. (2003). Chil-
dren Need Natural Languages, Signed or Spoken.
Technical report, Dana Foundation.
Bragg, D., Verhoef, T., Vogler, C., Ringel Morris, M.,
Koller, O., Bellard, M., Berke, L., Boudreault, P.,
Braffort, A., Caselli, N., Huenerfauth, M., and Ka-
corri, H. (2019). Sign Language Recognition, Gener-
ation, and Translation: An Interdisciplinary Perspec-
tive. In The 21st International ACM SIGACCESS
Conference on Computers and Accessibility - ASSETS
’19, pages 16–31, Pittsburgh, PA, USA. ACM Press.
Camgoz, N. C., Hadfield, S., Koller, O., and Bowden, R.
(2017). SubUNets: End-to-End Hand Shape and Con-
tinuous Sign Language Recognition. In 2017 IEEE In-
ternational Conference on Computer Vision (ICCV),
pages 3075–3084, Venice. IEEE.
Camgoz, N. C., Hadfield, S., Koller, O., Ney, H., and Bow-
den, R. (2018). Neural Sign Language Translation. In
CHIRA 2020 - 4th International Conference on Computer-Human Interaction Research and Applications
176